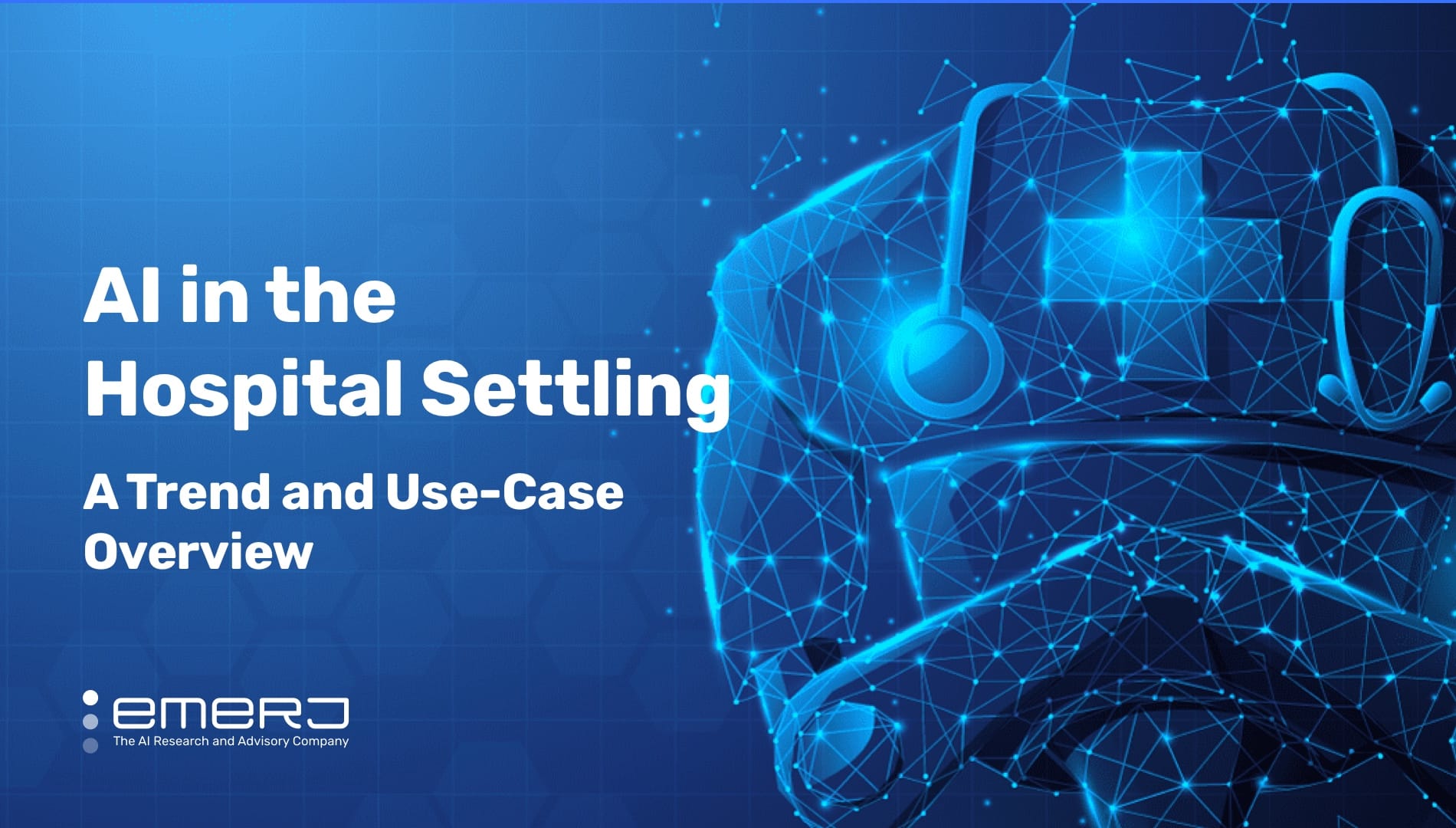
This article is based on a presentation at the 2017 AI Applications Summit conference in Boston, entitled “Artificial Intelligence in the Hospital Setting” (slide deck embedded below) delivered by Emerj CEO Daniel Faggella – updated with recent insights and interviews from Emerj’s podcast and AI Opportunity Landscape data.
In 2017, the wealthiest countries spent anywhere from $4,246 to $10,224 per capita no healthcare. In the case of the US, studies show that administrative costs have led to this high cost of healthcare. Some in the healthcare industry believe that artificial intelligence can reduce inefficiencies endemic in hospital administration in its delivery of care.
Today, AI has only nascent traction in healthcare, even in the most developed countries – but the technology shows promise for diagnostics, computer vision-related tasks, and some administrative and data management-related tasks. Hospital AI adoption lags behind what might be expected given the VC funding being poured into AI-related healthcare firms, and the huge number of AI healthcare startups around the world.
In this article, we’ll explore the challenges to healthcare AI deployment, and existing application trends:
- Major challenges with AI applications in the hospital setting
- Compliance and the “Black Box”
- Complex Stakeholder Relationships
- Chicken-and-Egg Problem of Case Studies
- Trending applications
- Diagnostics
- Medical Operations Software
At the end of this article, we’ll discuss what the priorities for executives in hospitals should be in the next few years.
The State of AI in Healthcare
We polled AI vendor executives selling into the healthcare environment, we asked them about the main reasons that AI adoption in healthcare has been slow. We found that the top two reasons for the lack of AI adoption in hospitals were:
- Buyers are not convinced of the ROI of the applications
- Lack of technology talent and resources to implement AI
We’ll explore both of these issues in greater depth:
Unclear ROI
It became clear in our interviews that not only is AI ROI hard to measure in healthcare (are we measuring financial savings to the hospital? To the patient? Are we measuring patient health outcomes? If so, by what metric?), but ROI is measured differently by different stakeholders. A new AI-enabled diagnostic software may help a hospital CEO reduce costs, but it must be something doctors are willing to use, and it must be something that nurses can be trained to understand, and its impacts on patient outcomes must be understood and potentially measured.
In banking or pharma, a top-down business decision to adopt AI has a chance of turning into real change and deployment. In healthcare, this is vastly less likely. We explore this issue in greater depth in the sections below about AI adoption challenges.
Scarcity of AI Talent
It’s not realistic for hospital and healthcare firms to be able to afford top-tier AI talent to help them integrate and implement AI technology. While the pharmaceutical giants wield billions in R&D and can recruit talent from top schools – most hospitals or hospital networks are unable to afford – or unable to retain – top data science talent.
A data science Masters or PhD graduate from Carnegie Mellon or Stanford is awash in lucrative and potentially exciting (or prestigious) job opportunities. Even if hospitals had enough funding to hire top-tier talent, they’d be placing those professionals in an environment what is wholly unprepared for AI transformation – a world with terrible data infrastructure, with very few peers who can speak the language of data science, and with regulations and compliance issues at every turn.
Many top graduates would rather get paid less working for Amazon or Google (of course, these are exactly the firms that are able to pay the best salaries) just in order to put their skills into action and stay sharp with other extremely skilled peers.
The AI Startup Ecosystem is Full of Pretenders
When we look at any given list of these companies, we would find that maybe only a third of them are actually leveraging AI in any way. Additionally, only about a third of those companies are past the stage of “past” their product or service.
This means that less than 1 in 8 or 9 AI companies with the talent and experience in AI to have actual traction in any meaningful way. In 2018 these numbers were more like 1 in 10, so while we’ve seen aggregate improvement in AI traction (and less blatantly faulty AI startups), the traction has been slow and arduous.
The AI Healthcare Startup Ecosystem is Still Nascent
Don’t believe your social media feed. Tech media perpetuates AI hype by implying that many hospitals are doing revolutionary things with AI. That is very much not the case – for reasons that we’ll address in the sections below.
Original presentation slides below:
Challenges with AI Adoption in the Hospital Setting
Assuming that AI is not yet there in the hospital space as sci-fi movies would have you believe, it is undeniable that it is getting some attention from executives. If you are considering jumping on the AI bandwagon, you should probably consider three major challengers with AI applications in the hospital setting.
Healthcare – Challenges (I): Compliance and the “Black Box”
In the early days of modern artificial intelligence, developers tended to “bake” human expertise into a software system, formulating if-then rules to come out with a prediction or solution. This gradually moved into the concepts of “teaching” software through machine learning or deep learning principles, which involves setting up a series of pattern-detecting nodes and filtering them with labeled examples to the point where the system can predict the output based on the input.
In the hospital setting, the if-then scenario was a lot easier for users to understand because they were based on deductive reasoning based on established human logic. For example, if the if-then scenario involved diagnosing an infection based on a consensus of 10 experts on the symptoms for that infection, then people would understand how the software came up with a particular diagnosis.
This is the “black box” problem of machine learning – and one that also holds back AI adoption in other sectors with heavy regulations or safety concerns.
However, in the ML version of the same scenario, there would be no easily understood if-then conditions. The software would ideally take the data of millions of patients, and detect patterns in their medical history, lifestyle, age, occupation, ethnicity, and so on to come up with possible diagnoses for a specific patient.
Doctors may have a difference of opinions about the accuracy of the diagnoses, but even if they agree with them, they would not be able to explain how the algorithm worked. As a doctor it’s difficult to really treat folks without being able to come up with an explanation for their diagnosis without going into complex issues.
It would be akin to asking me why I picked these three major points of friction in healthcare. I would know, but I wouldn’t be able to explain it simply. Similarly, if the doctors diagnosed someone with cancer, they couldn’t simply say, well, based on what we put into the system, this really seems to be what’s best. Right now machines are very poor at answering those problems.
The diagnostic software might be right more times than human doctors, but the machine learning systems would not be able to show a human how they arrived at a certain diagnosis. There would be no “black box.”
As a result, doctors are unwilling to use AI systems without understanding their logic, even if these systems could statistically deliver better diagnoses than doctors themselves. Additionally, compliance with regulations such as the Health Insurance Portability and Accountability Act (HIPAA) further makes machine learning challenging to apply with patients.
We looked for solutions to the black box problem, and we got some practical views. Steven Gullans of Excel VM is a venture investor in the Boston area and is one of dozens of investors we’ve interviewed about certain AI in healthcare applications. These are the belief that in terms of machine learning, what the black box problem of diagnostics and treatment might not the first areas that machine learning knocks out at the park.
He stated that AI may be better used in applications not related to diagnosis or treatment planning, such as:
- Drug discovery and development
- Managing patient populations/appointments
- Gleaning best-practices from hospital operations
He pointed out that fundamental developments in AI must be made to make machine learning explainable, or else AI’s role within a hospital setting will be significantly hindered. We might be able to see more traction in the drug discovery and development world and in terms of managing patient populations in healthcare operations software than in diagnosis and treatment as it is really going to be hard to move past the inherent issues with a generally opaque technology like AI, including regulatory compliance.
Healthcare – Challenges (II): Complex Stakeholder Relationships
Another inherent challenge in the hospital setting is complex stakeholder relationships. You might think that a hospital is in the service industry just like Amazon, but there are very few parallels between the two when it comes to the impact of AI for stakeholders.
For instance, when Amazon implements AI to improve product recommendations, Amazon pays for the technology that gives customers a better experience on the Amazon platform. This in turn encourages the customers to spend more money on the platform, which is a good thing for all of Amazon’s team members. They are incentivized to use AI to improve revenue and/or profit even if it’s a little bit tough to set up.
On the other hand, when a hospital applies AI technology to improve for MRI and CT scans, the patient might not even realize that AI is in play. There would be no upside to investing in AI in terms of patient satisfaction. Even if the technology leads to better patient outcomes and lower costs, there would be no concrete way to measure it right now.
Integrating AI technology in the hospital also requires doctors, nurses, and medical technicians to learn to use it, and in most cases, might not be paid for their efforts. Additionally, they might feel threatened that AI technology will take over their jobs, so they have no incentive to use AI at all.
Active resistance is understandable in situations when machines can outperform humans. Doctors in particular might be emotionally sensitive about being replaced by a machine after all that time spent in medical school.
The best way to overcome this friction to AI adoption in hospitals is for AI companies to align incentives with all stakeholders and get them onboard with the idea of AI. This would include:
- Providing massive research-based patient outcome improvements to demonstrate concrete ROI
- Positioning AI to do jobs that doctor, nurses, and medical technicians don’t want in the first place
- Develop specialist AI to supplement the skills of general practitioners outside of big-city hospitals as this will pose less of a threat
Healthcare – Challenges (III): Chicken-and-Egg Problem of Case Studies
AI is a hot topic across the board, but it is also disruptive technology. People trying to sell it often have to provide strong proof that the AI product or service they are selling work in a relevant scenario. This is not usually a problem as AI technologies are moving so fast in other industries. However, this comes to almost a complete halt as soon as they get to the healthcare industry.
When we talk to AI company executives selling into the healthcare space, we ask them to name their biggest sales difficulty in getting people to close. The issue is hospitals are somewhat resistant to try new technology.
Hospitals are slow to adopt new technologies without strong case studies to prove they work. Unfortunately, case studies for AI technologies are challenging to get because hospitals are reluctant to take a chance on them, hence the chicken-and-egg problem. Even if a hospital can be persuaded to take part in a pilot project, it often requires a long time to garner verifiable results. This factor compounds on top of compliance issues to slow down the industry adoption of AI in general.
Investors are also a big concern in this. They don’t want to invest in companies only to wait 40 years until they get returns, and AI companies will have a hard time developing and selling products without funding and without case studies.
To get past this friction point, Ai companies might want to concentrate on getting a foot in the door by offering non-controversial functions for their products and services. It could be business intelligence applications such as appointment management that would be less likely to meet resistance than diagnostic tech, for instance.
They could also develop AI to fill specialist roles in smaller hospitals to pose less of a threat to doctors and nurses, such as image identification. Once AI becomes part of the standard technology, it will become easier to introduce changes in other areas in the hospital space. It might take a while to break through the naturally conservative healthcare field, but it would be worth it,
Application Trends
Despite the challenges facing AI companies selling to the hospital setting, there is still significant progress in that space.
Healthcare Trends I: Diagnostics
In our assessment of the companies who are raising money in the healthcare field, diagnostics is by and large the most popular. It is worth noting that there’s a lot of promise in diagnostic technologies being developed as Software-as-as-Service (SaaS). There is also worth noting that machine vision for medical imagery is exceptionally common in terms of new AI applications, and may become a commodity.
The question is how many of these companies will come up with toyish level applications of AI and how many will truly leverage AI to layer that on top of someone’s genetic profile and health history. There is a lot of interest in using machine vision on images to diagnose diseases, but that’s actually a pretty shaky business model right now. Companies would have to integrate more data and do some rigorous testing and vetting of these predictive capabilities for hospitals to really adopt them.
Healthcare Trends II: Medical Operations Software
Medical operations software was the second most common AI applications being developed in our assessment of hundreds of healthcare AI companies. It’s something that we see people raising a great deal of money around, and I would say would gain much more traction in the hospital setting than the diagnostic technology.
The reason is simple: it is much less threatening and complex. For one this, it gets less rigorous scrutiny from regulations such as HIPAA. For another, there are clear financial and operational advantages to increasing efficiencies in tasks such as patient scheduling and financial collections.
For example, AI-based patient scheduling software would be able to predict staffing needs based on the number of patient visits and ER activity in the last few weeks. There would be just enough people on the floor at any one time. AI-based financial collection software would be able to track and monitor payments made by patients, schedule email reminders, and prompt staff to do follow-up calls. There would be no need to have a staff member to figure out who to call and save time in making calls or sending emails.
There is a lot of promise in AI-based software for managing hospitals. I think in terms of machine learning, we’ll see more traction here than we will in diagnostics in the near term within a hospital. That said, these top two types of AI applications being developed by companies are by no means common, even in the big R&D hospitals.
Adopt or Wait?
The hype around AI leads executvies across industries to the false belief that their peers are ravenously adopting AI, and that they are falling behind. While a realistic understanding of AI – and long-term digital transformation vision informed by that understanding – are important, that doesn’t mean healthcare leaders should aim to be “innovators” or “early adopters” of artificial intelligence.
However, very few hospital organizations have the R&D budget to handle genuine AI innovation – only the largest and most technical hospitals are likey to be actual innovators or early adopters – and the barriers to adoption will plague even these most cutting-edge facilities.
Most AI innovation in the healthcare space is likely to happen outside of hospitals entirely, namely:
- Diagnostic technologies will be developed at universities and research institutes. Hospitals themselves may provide data and serve as partners to universities, but hospitals will rarely have the data science talent or savvy to train unique AI models for their purposes. Common diagnostic issues (i.e. detecting lung cancer from chest X-rays) are best served by organizations that can pool data and expertise from many healthcare sources and train a single algorithm. Each hospital “reinventing the wheel” on training a computer vision system to detect hairline fractures in bone scan imagery would be madness.
- Non-healthcare specific administrative AI use-cases will be developed in other industries. AI-enabled search and discovery. Conversational interfaces. Optical character recognition and data intake. These applications are being used in insurance, banking, manufacturing, and other sectors – and will become more mature as they make their way into more enterprises. In addition, many of these “white-collar automation” AI use-cases aren’t mired in nearly as many regulatory issues, which might allow for easier adoption and deployment.
Smart healthcare leaders will stay abreast of AI developments in more innovative environments – and will aim to find vendors and partners who can transfer proven use-cases into the healthcare setting. Being in the early or late majority of AI adoption is likely to be the best position for the vast majority of hospitals and hospital organizations – particularly those without massive R&D budgets and high digital maturity.