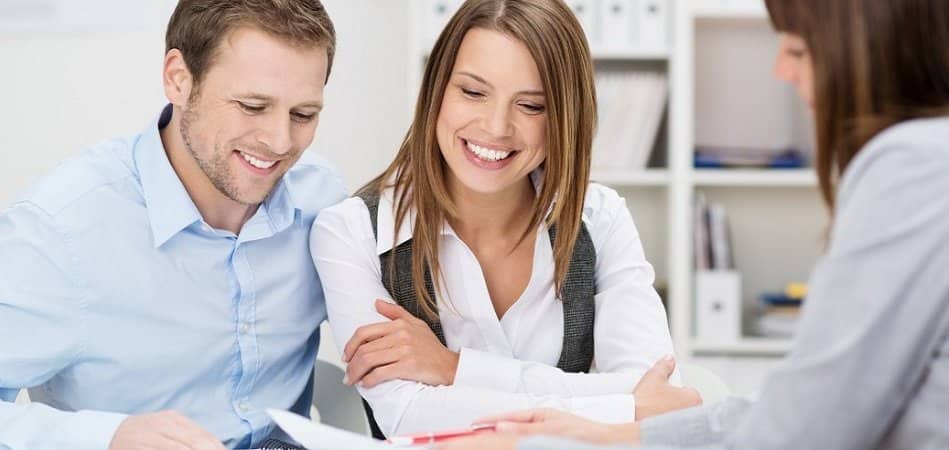
Along with the rise in popularity of chatbots and simple conversational interfaces, there is growing interest around other natural language processing (NLP) capabilities in the banking, finance, and insurance industries.
More often than not, large businesses find it challenging to unearth new insights from customer support. For instance, a large insurance firm might receive millions of text-based messages every year in the form of customer feedback or interactions during customer support activities.
Large firms may find it’s difficult to have human employees crawl through customer data to identify key customer issues at scale. The sheer volume of these incoming messages makes it difficult for banks to consistently leverage insights that might be gleaned from customer data.
For businesses that are looking to garner insight from their millions of historical customer interactions, natural language processing and machine learning techniques could help automatically discern what customers might be talking about.
We spoke with Peter Hoopes, VP WW Sales at Gamalon, Inc., who laid out some of the value that NLP might bring to customer service in industries such as insurance and finance.
According to Hoopes, machine learning and NLP can help extract insights from what customers are talking about when interacting with support representatives, commenting on social media, or filling out customer satisfaction surveys. Not only that, machine learning could help represent that data in a structured format that might allow businesses to take action. As Hoopes puts it:
We look for data that can be distilled into actionable insights from two main areas in businesses. (1) Customer support interactions: In many cases, the only interactions that a business might have with a client is when they have a problem that needs resolving. (2) text surveys: open ended surveys where customers can give their opinions on a product or service in natural language.
Natural Language Processing in Insurance – an Overview
The insurance industry has historically been dominated by large, slower-moving enterprises. However, in recent years, AI uses cases in insurance have gained traction, likely due to access to large volumes of customer data and resources in the industry.
A few forward-looking insurance firms seem to be applying AI to customer analytics. According to Hoopes, correctly identifying and extracting customer insights from this mountain of data is harder than it might appear, especially when training a computer software to understand the way humans communicate.
For a company with a large insurance customer base that interacts with multiple product types across communication channels, the difficulty is compounded.
NLP and machine learning could allow insurance firms to take large volumes of textual customer interaction data and find patterns within it to categorize and cluster these messages. In most cases, this still requires humans to read through a part of the data to label each message before it’s able to be fed to a machine learning algorithm.
These labeled messages allow the algorithms to better “understand” the context in a sentence. According to Hoopes, NLP software can look at patterns such as common words used in the beginning of a sentence or words used together in several sentences to either categorize new messages automatically.
Some NLP software, such as that offered by Gamalon, work a little differently. Instead of people labeling messages as “refund” or “cancellation request,” for example, the algorithm behind the software works by way of unsupervised learning.
Unsupervised learning doesn’t require people to label messages before they’re fed into the machine learning algorithm. Instead, the algorithm runs through raw text data and categorizes messages itself. Afterward, human subject-matter experts can tweak the categories that the algorithm creates, which continues to train the algorithm.
Hoopes suggests there are two areas where NLP can garner the highest ROI for insurance firms:
- Customer Support
- Survey Analytics
We look at both of these use-cases in more detail below:
NLP for Customer Support in Insurance
According to Hoopes, a big factor contributing to why insurance firms might invest in AI for customer service is that customers have most of their interactions with insurance firms when they have an issue, when something has gone wrong.
Hoopes believes that businesses that have low levels of interaction with their customers will find it most attractive to start NLP initiatives to improve customer service.
He explains the challenges in garnering insights from customer feedback with a health insurance example. If a large health insurance firm received a million customer messages about claims applications or as feedback, one challenge it faces is to identify which phrases are referring to the same things. Hoopes says insurance customers might say the same thing in many different ways:
Let’s say a business receives a million messages from customers every year, across many channels. Assuming that 50% of these people are looking for a doctor, there might be 500,000 ways in which people are asking for a doctor. For example, customer might use the terms I’m looking for a doctor, I’m looking for a specialist or I’m looking for a medical expert, all of which mean that the customer is looking for a doctor.
An insurance firm looking to read through a large number of customer service tickets could use NLP to automatically categorize the top issues customers are facing and map action-items to relevant departments. The AI software can run through the customer service tickets and list the things that are being talked about the most, along with further details about each issue.
For example, the NLP algorithms might identify that several customers have mentioned a particular insurance product alongside terms that signify negative sentiment. Additionally, the software might also identify that the word “price” was often used in complaints about that product. The insurance carrier can then identify the product that customers don’t like, which in this case might be due to its price, to alert the relevant division.
There could also be a case where a customer calls in for feedback and states that the customer service representative was rude, but the online experience was good. In such cases, identifying how messages that contain both positive and negative sentiments about two or more different concepts might be challenging for NLP algorithms without a significant amount of either labeling or tweaking by subject-matter experts depending on the approach taken to train the algorithm (supervised or unsupervised).
In addition, NLP might also allow insurance firms to monitor incoming customer data in real time to help identify issues and take action before they affect a large number of customers.
For example, a large health insurance firm might be able to use NLP software to automatically categorize customer service tickets into buckets. As a result, customer service managers might notice that over 25% of the complaints categorized in the last one month were about login and password issues, for example.
The company could then proactively take action and alert their IT team before the issue affects more customers, essentially giving insurance firms the ability to prevent problems from compounding.
NLP for Surveys Analytics in Insurance
In the insurance sector, businesses might not have regular interaction with their customers through customer support channels. Hoopes explains this with an example:
If you use an internet connection and it fails, the internet providers are aware that the customer is facing an issue. It’s possible to find out about customer perceptions a lot easier.In insurance, businesses might not have much information on how customers are talking about their products. Auto insurance providers might see the transactions on a customer’s services account, but might need to survey the customer to find insights about what the common customer perceptions towards brands and products are.
As such, many insurance large insurance firms will have backlogs of survey data from which employees are unlikely to be able to glean actionable insights; there are simply too many surveys to sift through.
According to Hoopes, insurance firms could make better sense of their survey responses through the use of NLP and machine learning.
For example, an auto insurance firm may only interact with its customers when they file a claim or when the customer reaches out for customer support. To remedy this, they might collect data about what customers think about their brand and product through open-ended text surveys that they send to customers. If they fail to anticipate just how many surveys they’ll receive, they might find that the cost to sift through all of them to garner any meaningful insight is too much to bear.
Given a large number of survey responses, the auto insurance firm could use unsupervised NLP to automatically categorize these responses. Subject-matter experts could then review the categories to get a general sense of how customers feel about their brand and products. If they have on-staff data scientists or are working with a vendor that allows for it, they could also tweak the algorithm so that it categorizes responses the way they want it to.
Hoopes states that Gamalon worked with a major health insurance player to identify the top customer issues from millions of customer survey messages.
According to Gamalon, the health insurance carrier was facing challenges in gaining valuable insights from their survey responses due to the large volumes of survey data. The existing rules-based survey analysis tools seemed to be failing on delivering insights.
The vendor worked with the insurance firm to analyze the customer feedback collected by the firm. The data was in the form of unstructured open-ended customer survey responses. Gamalon claims they helped the health insurance firm categorize the survey responses in a way that provided actionable insights for the sales team.
A supervised learning NLP approach could also work for such a scenario, but it would require time spent beforehand on labeling survey responses as the categories that the insurance firm wants the algorithm to sort responses into.
Concluding Thoughts on NLP for Insurance Customer Insights
In summation, the core value propositions for investing in NLP for customer support and generating customer analytics in insurance include:
- Categorizing customer support tickets
- Categorizing survey responses
- Routing customer support tickets to the appropriate departments
- Learning what customers think about an insurance firm’s brand or products
For the full scope of what’s possible with NLP in insurance, business leaders may want to read our full report on the topic.
Insurance firms are right now applying NLP to improve their customer service operations and inform product development. Any such endeavor still might be most relevant for medium to large enterprises with sufficient access to data, capital, and data science talent. Local insurance firms will need to wait a while before AI, in general, becomes more available to them.
For insurance enterprises looking to adopt NLP into their customer service workflows, they will first need to figure out the kinds of insights they are looking to garner from their customer support data. To do this, they will often need their subject-matter experts to work with data scientists, either those that work at the company itself or those that work at an AI vendor company.
Insurance enterprises might work with a vendor that provides an unsupervised NLP software that can start categorizing customer messages relatively quickly after purchasing the software, but such vendors are rather uncommon. In these cases, such as with Gamalon, subject-matter experts don’t need to label data, but instead, tweak the categories that the algorithm creates on its own.
For the most part, NLP vendors offer supervised learning, which requires an integration process that includes subject-matter experts (in this case, likely customer support staff at the insurance firm), to label customer messages as certain categories. This would train the algorithm behind the software to accurately categorize messages itself.
NLP software might now be able to help large insurance firms get a deeper understanding of what their customers are most often talking about or having issues with. It’s important, however, to begin AI initiatives with a clear objective. In this case, insurance firms should know the kinds of insights they’re looking to garner from an NLP software before they work with an AI vendor or hire a team of data scientists to build an algorithm.
This article was sponsored by Gamalon, and was written, edited, and published in alignment with our transparent Emerj sponsored content guidelines. Learn more about reaching our AI-focused executive audience on our Emerj advertising page.
Header Image Credit: Paradigm Life