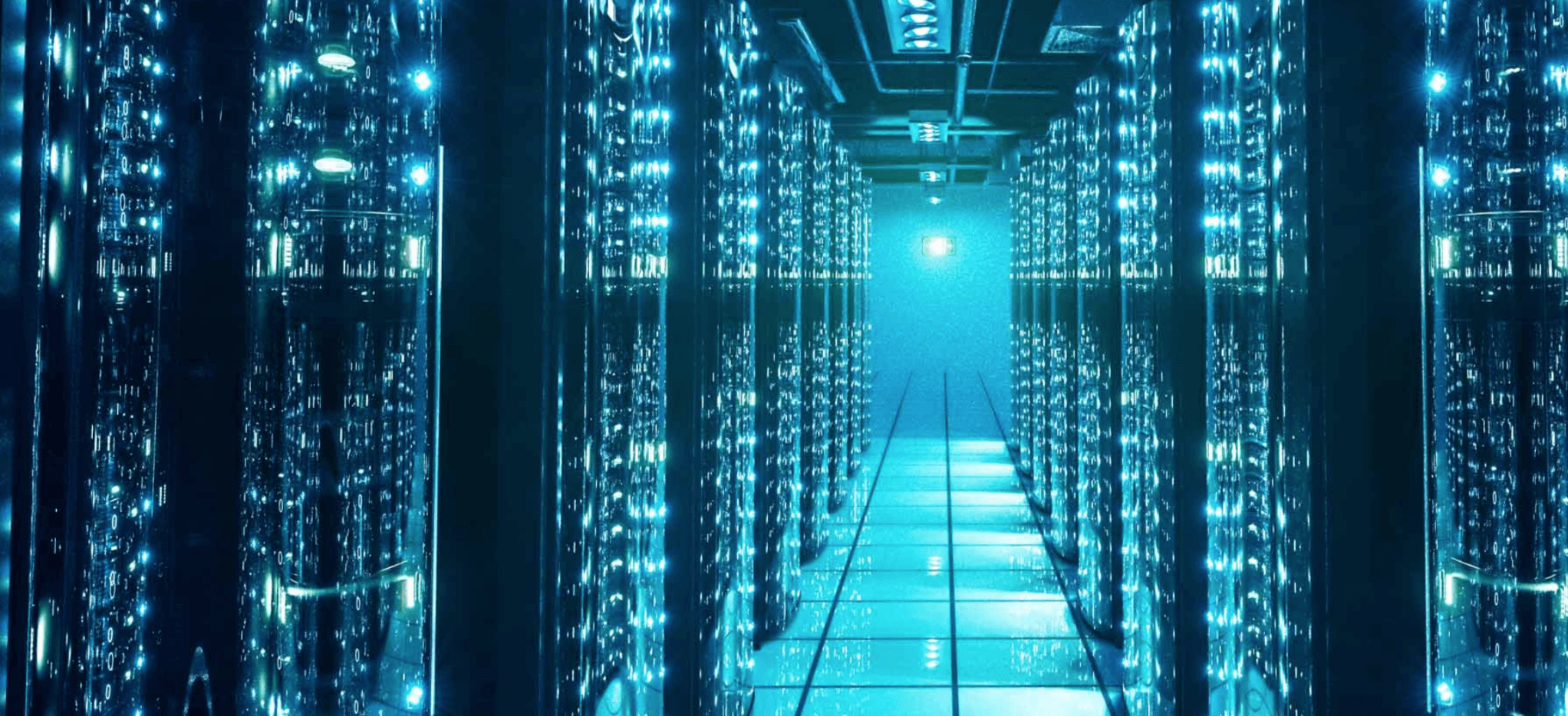
Implementing artificial intelligence into an existing business is about more than algorithms. In fact, many AI researchers believe that algorithms are the easiest part of an artificial intelligence implementation.
Algorithms need data, and for a business to assess, organize, clean, and use it’s data requires ways of thinking that are entirely foreign to most existing enterprises.
Partnering with Corinium Global Intelligence, we asked six experienced AI and analytics professionals (all speakers at Corinium’s Chief Analytics Officer Spring event in on May 14th-16th in San Francisco) the following three important questions:
- What does an executive need to understand about data management before they can successfully leverage AI?
- What kind of fundamental data infrastructure and data management technologies need to be in place before an enterprise can successfully leverage AI?
- If an executive is planning to use AI in their firm, what do they (personally) need to understand about data management in order to properly lead such a transition?
In the sub-sections of the article that follows, we’ll explore each of these questions in depth, highlighting the best insights from the professionals we corresponded with.
What Executives Need to Know About Data Management
IT procurement, software development, and software aren’t new concepts to many experienced executives. They may not do the development themselves, but they know how the process works, how it is managed, and – quite often – some reasonable estimate of how long the process will take.
Data management, however, isn’t something that many C-level executives or VPs have had to think about seriously in the previous decade.
While some of the technologies of data science are new, some principles never change. Robert Lanning is Director, HRIS and People Analytics, Silicon Valley Bank:
“My father was one of the old-school FORTRAN and COBOL programmers: ‘We used punch cards to get things done and we liked it.’ While the programming languages and methodologies have evolved tremendously over the past 50 years, the principle of ‘garbage in, garbage out’ still remains.
I’m amazed to see a number of data scientists think that they can just throw data at a tool and hope that the tool produces the right result. Careful planning and meticulous data management (including rigorous data integrity checks) need to take place before any semblance of quality results will emerge from any AI solution.”
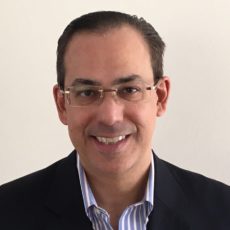
As many of the respondents mention, however, the specific data management practices depends the applications you’re aiming to build. Jose Antonio Morillo is Chief Analytics Officer, Grupo Financiero Banorte:
“New technology is one of the main driving forces. However, the correct usage depends on the application. For instance, there is no need to change from SQL databases to NonSQL if the application doesn’t require it.”
This sentiment is echoed by Eugene Wen, Vice President, Group Advanced Analytics, Manulife:
“They need to understand common options of data strategies. They need to have a good understanding of business strategy of the company too. Data management is subject to the AI plan.”
It should be noted that the strategic decision-making for determining a company’s data needs (given its AI projects and objectives) is a job that involves a high degree of expertise in and of itself.
Peggy Tsai, Vice President in Analytics and Data, Morgan Stanley Wealth Management, mentions this overtly in her advice for business leaders.
“Hire a Chief Data Officer who can strategically plan and lead a data management program. Data is complex and needs the support of senior executives in order to be successful. Stay personally engaged and give the data organization a place at the table to present their findings.”
This is not an easy hire to make – particularly given the talent crunch in the machine learning and data science domain – but it’s a position that’s critical for getting data right.
The Data Infrastructure Needed to Leverage AI
In addition to asking Corinium’s speakers about data management basics, we also asked them about what businesses need to know about data infrastructure.
Vituity’s Chief Data & Analytics Officer, Dipti Patel-Misra, listed some of the infrastructure elements that she recommends for businesses:
“Data collection, processing, storage, privacy, security, data governance, and someone who understands data and process.”
Again the emphases on a data expert is emphasized – reinforcing the notion that data infrastructure challenges related to AI are indeed a new domain involving specialized experience.
Jose Antonio Murillo:
“It really depends on the data we have and the AI applications we need. Does the data need to be processed in real time? Is the data structured or unstructured?
In general, we would like to aim for technology that is secure, highly and easily scalable and accessible, and that can be integrated with other technologies.”
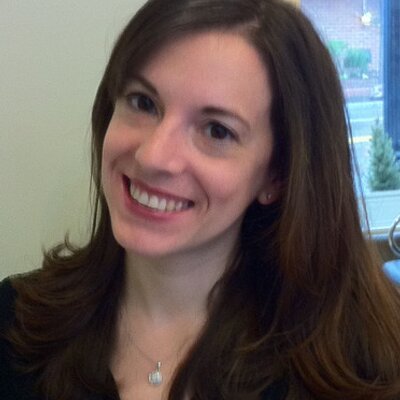
Amy Gershkoff is Former Chief Data Officer at Ancestry and states that:
“Before building AI or ML algorithms, the most successful organizations ensure first that strong data governance is in place, which generally involves a combination of technologies, policies, and best practices.
Most successful organizations also remember that AI/ML is a journey, not a destination – therefore, it is critical to create an infrastructure that incorporates technologies that are flexible and scalable to meet the future needs of the organization.”
Readers who are less familiar with the concept of “data governance” may benefit from reading the main article on data governance basics from the Data Governance Institute.
Advice for Executives Planning to Adopt AI
Our third and final question for our panel of experts was a request for final advice on data management for business leaders who are planning to adopt artificial intelligence in the near future.
Amy Gershkoff hammers home points echoed by many of the respondents. Namely, that the vigilance of teams around data and data-related expertise is much more important for leveraging AI in business than the mere choice or use of algorithms:
“Success with AI or ML is rarely about the algorithms themselves, nor is it typically about the technology choices made or vendors utilized – rather, it is about creating and maintaining a platform for data-driven and algorithmic-driven decision-making across the organization.”
She also mentions the need for business leaders to remain flexible, establishing systems purpose-built to adapt to new applications. In addition, she makes it clear that executive buy-in and leadership is critical to bring AI to life in a business. The investment of time, money, and new expertise is far too much to make a haphazard or merely symbolic commitment to data management and governance.
“The platform and systems need to be flexible to scale to future use cases, and there must be widespread organizational buy-in across departments for AI/ML to be successfully leveraged.”
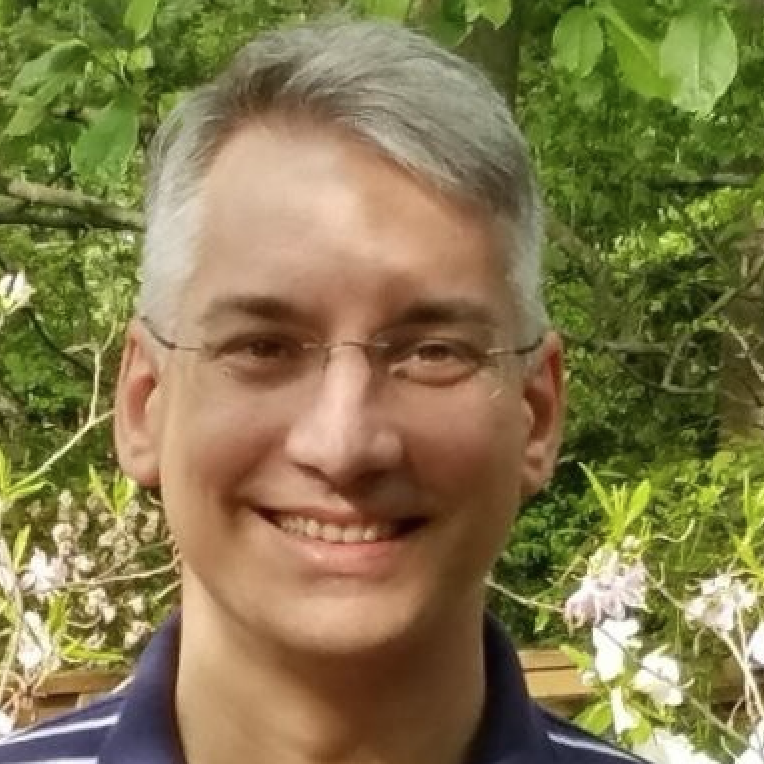
Kevin Bates, VP of Enterprise Data Delivery at Fannie Mae, brought up a point that we hoped would be mentioned. Namely, that companies without a firm grasp on their data-related processes should be tentative to implement AI. When it comes to enterprise AI adoption, “walk before you run” is the phrase that comes to mind:
“AI, Machine Learning, and advanced analytics are excellent tools to enable an organization that already has a strong handle on their data.
If an organization is still early in the process of developing data management capabilities, they would need to be careful moving forward with AI or advanced analytics solutions. It is still possible, but the solutions would take a different form, and the type of actions that could be justified by insights from the AI practice would vary.”
As a nascent field involving entirely new processes and expertise, many companies would be best served by clarifying their AI vision, but working towards that vision in steps that are likely to succeed – rather than with naïve gusto that’s destined to hit a brick wall with unprepared people, departments, and infrastructure.
This article was written in partnership with Corinium Intelligence. For more information about content and promotional partnerships with Emerj, visit the Emerj Partnerships page.
Header image credit: Corinium Events