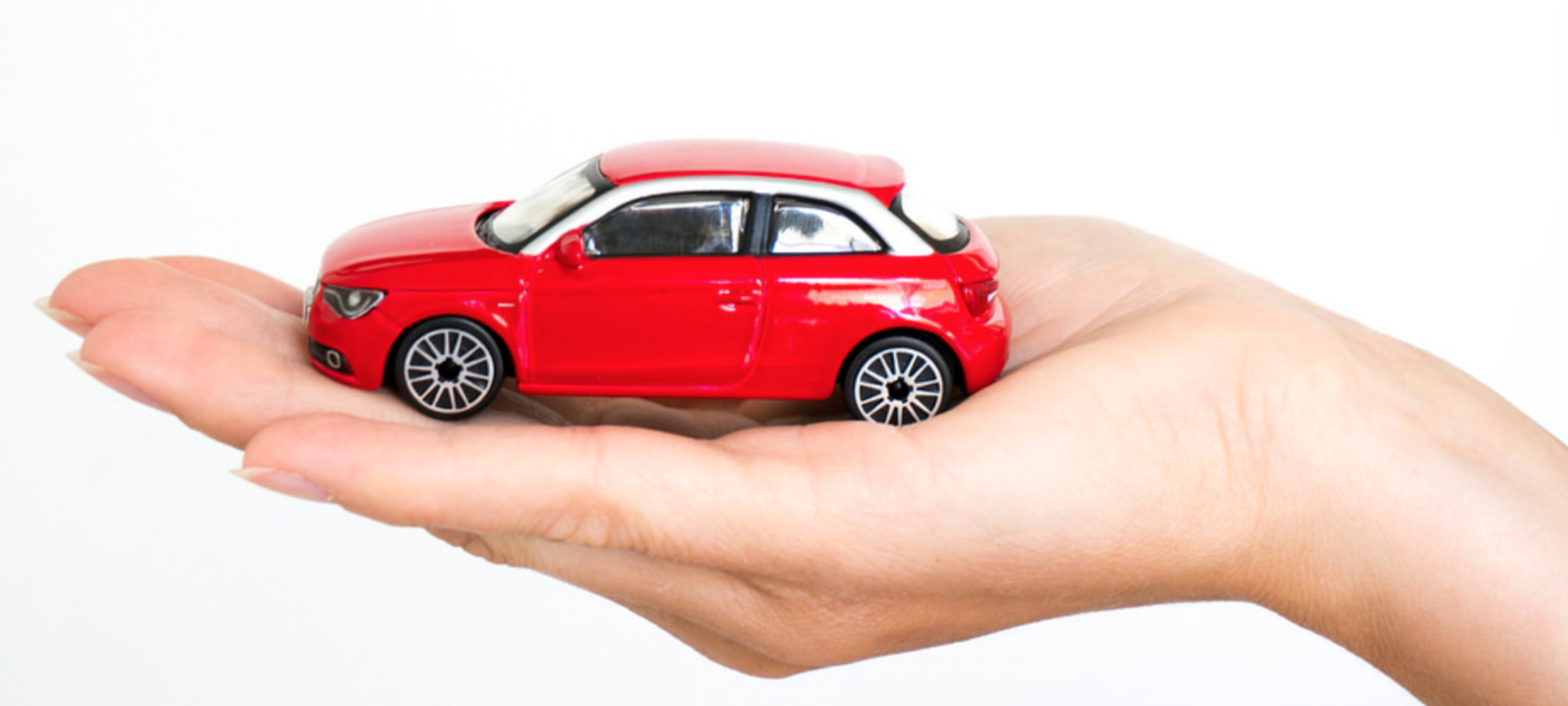
The ability of companies to collect external data is likely to change the insurance industry as it is today. Traditionally, insurance companies would collect internal data from customers: data such as their weight, gender, and any family history of health issues. However, with the advent of technologies making possible the collection of data specific more to individuals than large groups of people, insurance companies could be able to offer unique, tailor-made policies to their customers.
This external data includes behavioral data; in other words, using AI software, insurance companies could be able to track customer use patterns that will inform insurance companies on the risk of specific individuals. A driver who speeds or stops abruptly might pay more than a driver who does not, and with IoT tracking devices, insurers might be able to adjust customer policies in real time based on this information.
IoT devices could prove themselves the future of quite a few types of insurance. In this article, we break down the possibilities for IoT devices for different types of insurance and take a look at the IoT devices that may have the most impact on insurance companies going forward.
The insights in this article were derived from the recent Insurance Nexus white paper titled: Turning Innovative Tech into a Competitive Advantage: 4 Tools to Effectively Leverage AI, Blockchain, Chatbots, IoT and More. Click here to download the report.
Auto Insurance: Driving Sensors, and Autonomous Vehicles
Perhaps most prominently, some auto insurance companies, such as Progressive, have already begun implementing AI into their business by way of IoT devices. Their Snapshot program works as follows: customers put an IoT sensor into the glove compartment of their car, and the device tracks the customer’s speed, stop frequency, turn motions, frequency of maintenance, and other driving habits.
The following 1-minute marketing video is intended to convey the value proposition (lower prices for safe drivers) is conveyed to customers:
Progressive in turn receives a large volume of driving data—reportedly over 14 billion miles worth at the time of writing this article—that its machine learning software can use to extract the patterns that exist between a driver’s demographics and their driving habits and then offer a rate to customers that is tailored specifically to them. In other words, customers would pay a rate based on the risk they pose to the insurance company.
Indeed, vehicles themselves are becoming “smart” IoT devices. Self-driving cars have the potential to seriously disrupt the insurance market. Warren Buffett believes that the popularization of autonomous vehicles will result in less drivers on the road and in turn hurt the Berkshire Hathaway-owned Geico.
In fact, a 2015 KPMG report predicted that autonomous vehicles could contribute to shrinking the auto insurance industry by 60% by 2040. Considering that auto insurance makes up 40% of the total insurance market, it would stand to reason that, in this case, an IoT device like the self-driving car would actually hurt insurance companies across the board.
While it’s likely that some forms of insurance may become obsolete with changing technology (as is the nature of the market), a recent Accenture report estimated that autonomous vehicles could generate $81 billion in revenue for insurance companies as a result of new forms of insurance, such as insurance for cybersecurity and product liability insurance for IoT devices.
Health and Life Insurance: Wearable IoT Devices
In the health insurance industry, wearable IoT devices could send customer data to the insurance company that gives a more complete profile of the customer, taking into account their behavior in addition to their demographics. For example, the IoT may be able to track whether a customer drinks or smokes or whether they lead a mostly sedentary lifestyle. Their premiums would likely increase, whereas conversely, a person who does not uses substances and exercises often would see their premiums decrease.
In theory, these premiums could rise and fall in real time. Perhaps a month of increased drinking would increase a person’s premium for just that month before returning to their baseline premium the next month as their drinking returns to their more standard frequency of alcohol use. As a result, IoT devices could incentivize customers to lead healthier lifestyles because the consequences of not doing so would be more tangible and more immediate (a larger bill at the end of the month).
This could be great for insurance companies because it means less of their customers would be likely to lead riskier lives, increasing the likelihood that they’ll end up profitable.
That said, according to Insurance Nexus’ Morag Cuddeford Jones in her piece 4 Tools to Effectively Leverage AI, Blockchain, Chatbots, IoT and More, author Morag Cuddeford Jones writes, “‘Plugging in’ to the customer isn’t simple across every line of business.” She recounts the words of an unnamed life insurance company’s representative:
If a life insurance company looks at my health data and says it’s not great, knowing that I could get a better rate if I could make my numbers look better, all of a sudden I’m more worried about making my past data look good than making lifestyle changes that improve my health. That creates problems. I could see people coming up with ways to manage those numbers. Right now we don’t know how do we effectively handle that, which is why most of the life insurers are staying away from leveraging wearables for anything but offering a few incentives.
At least according to this life insurance representative, life insurers seem to be avoiding IoT devices for ethical concerns. If they were to adopt the technology, however, it’s likely it would look similar to the wearables that might be offered through health insurance providers.
Voice and Customer Service Data from IoT Devices
More generally, as millennials enter the insurance market, companies are going to have to respond to that generation’s expectations, those which they are carrying over from their values as consumers of other goods and services. According to Jones, millennials:
“Feel they understand the bargaining chip inherent in a fitness wearable, telematics system or smart thermostat. [They] aren’t wondering why carries sell policies that include connected devices. Instead, they don’t understand policies that can’t use wearable or connected home data.”
In other words, despite ethical concerns, Jones believes millennials are possibly eager for IoT devices and willing to try them in various aspects of their lives if it means convenience or cheaper prices. Theoretically, insurance companies would be able to take advantage of millennial sentiments in order to experiment with IoT technology.
Roman Dumiak, Director of Technology at Allstate, believes that there’s a benefit to being able to, as Jones puts it, “create pilots” or “sprints:” low-investment trials for implementing technology such as IoT devices. Dumiak claims that Allstate was among the first companies to offer Alexa skills to customers. With the Allstate Insurance Skill, customers can ask Alexa for their Allstate balance, ask when it’s due, and ask for the location of the nearest Allstate representative.
For what appears to be customer loyalty, Allstate also offers customers the Good Hacks and Flash Briefing Skills. These skills have nothing to do with the customer’s policy, nor anything to do at all with insurance. Instead, they seem to be offered to the benefit of customer loyalty. The Good Hacks Skill provides those who ask with helpful tips for navigating mundane tasks, such as how to remove stains from clothes. The Flash Briefing Skill provides customers with ideas for good deeds.
Below is short 1-minute demo video of Allstate’s Good Hacks Alexa skill:
These seemingly incongruent applications apparently have real business value for Allstate. Roger Tye, Vice President of Digital User Experience at Allstate, believes “As the technology evolves, Alexa will become a discovery mechanism. Companies with the best answers will strive to the top.” We have no information on how exactly Allstate’s Alexa Skills have affected the company’s bottom line at this point, although we believe Tye’s reasoning makes some sense.
Insurance companies could possibly garner a customer’s loyalty by asserting themselves into customer’s lives (when requested) by way of a technology that is certainly becoming ubiquitous. Theoretically, a customer might think twice about changing their insurance company because Allstate is the one that keeps helping them with their everyday lives, whether they need to find and seal an air draft or remove pet hair from their clothes.
Dumiak also says that this venture into Alexa also provided the company with customer information that allowed them to make use of machine learning, specifically when it came to developing chatbots.
It’s likely that the chatbot itself was built off of information garnered from the Allstate Insurance Skill and not the Good Hacks or Flash Briefing Skills, considering that questions to Alexa going through the Allstate Insurance Skill would relate directly to customer service. It stands to reason that their chatbot is built for customer service as a result, although we could not find information on how exactly the Allstate Insurance Skill informed the chatbot.
It might also be possible that, based on conversations with the Good hacks and Flash Briefing skill, Allstate could retrieve customer data on individuals. For instance, perhaps customers who ask Alexa certain kinds of questions (potentially questions that signify affluence, or signify a new customer need) are more or less likely to be upsold to a new insurance product. Perhaps certain kinds of questions are a signal that a user might be a higher or lower risk for accidents. We haven’t seen any evidence of this kind of customer profiling at present, but it seems like a viable use case is Allstate was able to garner a critical mass of Alexa users.
It follows that a foray into customer data is likely a good investment because robust customer data sets will allow for the implementation of more accurate machine learning algorithms, thus saving businesses on time and money in areas like customer service and, as previously discussed in this article, allowing companies to offer tailor-made policies that may entice customers.
Achieving AI Success – Data Cleaning and Data Infrastructure Considerations
However, not all customer data is the same. Dumiak asserts that clean data is the way to go, and we agree. Clean data is that which is organized, accurate, and easy for an AI system to work with. Jones suggests “Companies that already have a data organization system in place and are already confident their data is clean are in a good position to move forward fast.”
What this means is that even before experimenting with IoT devices, a company should make sure that the data they have on their customers is already relatively robust and organized. It’s also imperative for an insurance company to make sure that the data they do receive from IoT device usage enters into a data repository that allows for relatively simply access and reference, such as a data lake (though data storage needs will vary depending on the company, applications, and types of data collected).
Disorganized data will make it harder for the machine learning system to parse the patterns from the data, which is its entire function. Any patterns it does find may be compromised as a result of inaccurate data, rendering it useless and, ultimately, a waste of time and resources. In writing our last article with Insurance Nexus, it became clear that many insurance leaders feel that their current data storage approaches need to change – and the process isn’t easy.
Data cleaning involves removing inconsistencies and redundancies within data tables. For example, an insurance company might theoretically have one client listed multiple times under different policies due to some oversight in their sign-up process. If a problem like this happens in numerous instances, a machine learning system may be making predictions based on overrepresented data.
A thorough data clean would involve removing these duplicate customer entries, correcting any spelling errors within the data, removing data marked invalid, and filling in any missing data that an insurance company might not yet have on a customer or which may exist elsewhere in the system. Although a manual cleansing may involve considerable human resources depending on the size of the company, there exists software that claims to speed up the data cleaning process.
Below are three methods for ensuring that a business does not find themselves with a data set that is too chaotic to feed into a machine learning system. The steps below relate to CRM and marketing data, but could potentially be applied to other domains as well.
- Businesses should make sure the data they import into CRMs is clean prior to import, and this is especially true when switching CRMs.
- Entering your data into a spreadsheet can help you clean the columns that the CRM will read, naming the columns in the spreadsheet those which are listed in the CRM.
- Update customer and prospect information whenever you receive updated information.
- If a customer has multiple emails that create for them multiple accounts with your company, consolidate those accounts right when you learn of the multiple emails.
- Businesses that call customers and find that they’ve called a wrong number should be diligent in reaching out to the customer by other means in order to obtain the customer’s updated phone number.
- CRMs that are capable of it can search for customers who are missing information on their records, such as a phone number or an address. Then, businesses can reach out to these customers for the missing information.
We’ve said it before: Any company interested in AI needs to have a coherent strategy for data collection and storage, and this is especially true for the insurance industry, where customer data is the name of the game (and the potential for bottom-line business impact is massive).
Concluding Thoughts
The ubiquity of IoT devices might very well be inevitable if it isn’t already. As younger people enter into the insurance market, insurance companies will have to offer products that suit their lifestyles and values, and according to some in the industry, millennials may allow IoT devices in their homes, in their cars, and on their bodies in order to secure discounts, convenience, and more personalized experiences with the companies from which they purchase.
If this pans out, it may bode well for insurance companies looking to collect customer data that they can use to target marketing not just to specific demographics, but individual people. Companies that make use of the clean data they receive from IoT devices may find themselves with a competitive advantage over their competitors who waited on experimenting with IoT devices despite having the funds. If anything, even a failed venture into IoT devices could prove valuable if enough customer data is collected during the trial run.
This article was sponsored by Insurance Nexus, and was written, edited and published in alignment with our transparent Emerj sponsored content guidelines. Learn more about reaching our AI-focused executive audience on our Emerj advertising page.
Header image credit: Adobe Stock