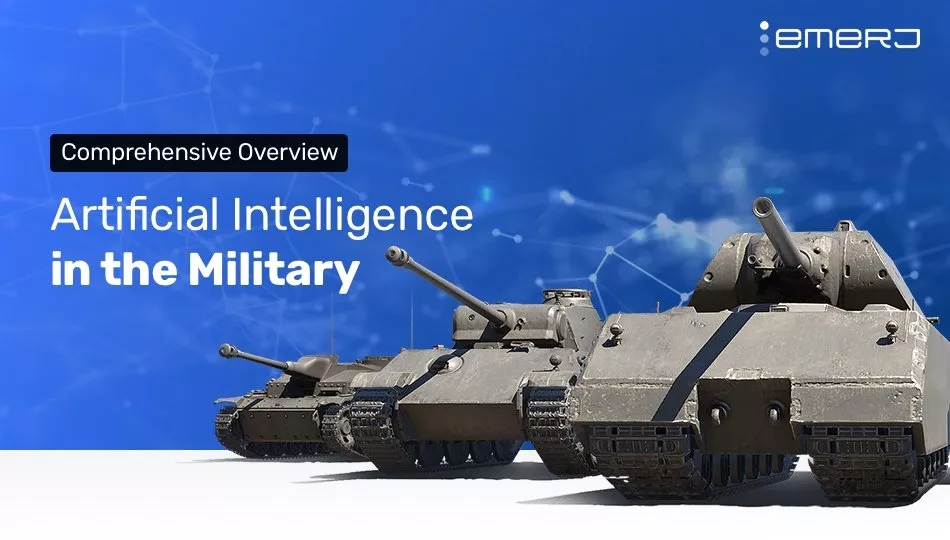
We researched the military and defense space to discover how and where AI is utilized today by the world’s militaries and intelligence organizations as well as the capabilities artificial intelligence could bring to the sector shortly.
Modern militaries are comprised of many subsections. They have marketing departments like any large company, must issue payroll for their staff, and process documents in ways very similar to corporations. For the sake of brevity, this report predominantly focuses on the capabilities of artificial intelligence specific to combat operations.
Militaries and defense organizations can use AI for:
- Autonomous Weapons and Weapons Targeting
- Surveillance
- Cybersecurity
- Homeland Security
- Logistics
- Autonomous Vehicles
Interested readers may want to read our report on AI at the top 5 US defense contractors, but we’ll start this report with AI for autonomous weapons and weapons targeting.
Autonomous Weapons and Weapons Targeting
Targeting systems are judged on their accuracy and how quick to lock on to a target they are. As countermeasures for this style of targeting grows in popularity, electronic targeting systems must then innovate to become less susceptible to those countermeasures. It follows that machine learning and computer vision would be the next application for this technological game of cat and mouse.
Today autonomous weapon platforms are using computer vision to identify and track targets. An autonomous weapon primarily becomes autonomous when the system is able to identify, and track targets in the space it has been deployed to guard.
The artificial intelligence behind the targeting would need to be trained on what exactly a strategic target is worth focusing its firepower on and alerting the operator monitoring the platform. This may be an enemy aircraft flying into contested airspace at extreme speeds, a rocket fired at a town, or an armored personnel carrier driving up a minored road.
Currently, there are no autonomous weapon platforms that are being designed to fire its ordnance without the express approval of a monitoring operator. One strategic advantage autonomous weapons may provide over traditionally manned systems is that the latter are susceptible to human distraction and needs.
Autonomous weapons allow the ever-vigilant “eyes” of computer vision to be trained on the skies above to prevent surprise rocket attacks by targeting and shooting down enemy rockets in the air before they can detonate in a populated area. Valuable seconds to respond may be lost if a human operator is distracted or dozes off while posted.
We broke down this technology at work in “RAPIDFire,” an autonomous weapons turret manufactured by Thales, in our article on the AI projects at the top European defense contractors.
Below is a short 3-minute video demonstrating and highlighting RAPIDFire’s targeting capabilities:
The machine learning models behind this kind of targeting software would be trained on visual data from camera mounted on turrets and other weapons platforms collected from previous projects undertaken by the company from various angles and in various lighting conditions.
These images and footage would have been labeled as a target, hostile action, or natural terrain. They would then be run through the machine learning algorithm. This would have trained the algorithm to discern the sequences and patterns of 1’s and 0’s that, to the human eye, form the image or footage of a target, hostile activity, or natural terrain as displayed in the tracking interface.
Computer vision is currently being applied to missile systems for handheld missile launcher systems as well as larger Naval Strike Missiles.
While in flight and viewing a target attempting to deploy countermeasures or evasive maneuvers, the missile targeting software would allow the missile to react appropriately and still connect to its target.
Rafael Advanced Defense Systems claims their GIL 2 handheld rocket system uses computer vision for enhanced targeting, which we cover in greater depth in our report on AI at Israel’s top defense contractors.
According to Navy Recognition, the HE-4G missile by L3 technologies is still in development but demonstrated success at the Potomac River Test Range where it hit a moving target with more accuracy at longer ranges than standard projectiles. In 2017, the US Navy reviewed its design and passed it, allowing the program to proceed to important qualification activities and then to potential deployment.
AI can also be used as a weapon in the cybersphere. The Behavioral Learning for Adaptive Electronic Warfare system, or BLADE, is a system meant to attack and disable wireless communication networks using artificial intelligence. The BLADE Program, in late-stage testings, is a system created by Lockheed Martin in a $29 million contract with DARPA.
Although details on this project are obscured, likely for security purposes, it is known that AI plays a pivotal role in BLADE’s ability to detect and characterize new communication threats, learn how to jam it, and then assess jamming effectiveness in the field. Additionally, Lockheed Martin claims BLADE is able to predict the countermeasures of the enemy software and adapt with them for sustained jamming.
A cyberweapon able to disable wireless communications on the battlefield like BLADE goes beyond voice and text communication disruption as it can also provide a means of Improvised Explosive Device (IED) neutralization.
Often, small commercially available drones can be used as remote control bombs, allowing terrorists to attach small explosives on a drone and fly it over a military base. The drones are wirelessly detonated either via a cellphone or the drone remote itself. BLADE could disable the wireless communication signal of the remote or cell phone attempting to detonate an IED, resulting in the neutralization of the drone and the explosive, preventing the terrorist attack.
Surveillance
Militaries around the world acquire enormous amounts of visual surveillance data a day from various sources, such as phone cameras, laptop feeds, video surveillance, planted cameras, UAVs and satellite footage. The challenge is not collecting the data, but processing it for strategic information, and this is where machine vision and AI could be of use.
Machine vision software has the potential to sort through large sums of data for insights faster than trained human analysts. The US Department of Defense and other national organizations currently use machine learning and computer vision software for surveillance operations.
For example, as described in our report on machine vision in the military, Project Maven is an attempt by the US DoD to create computer vision AI that can categorize and identify the huge sums of surveillance footage taken by low altitude combat surveillance equipment like UAV’s.
We can infer from material stated by Google and the US Department of Defense the machine learning model behind the software used in Project Maven was trained to identify 38 different kinds of objects and so the AI was shown those individual objects across hours of footage from various angles and in various lighting conditions.
The objects within the footage would have been labeled as what we know the objects to be, such as a traveling car, a weapon, or a person. This labeled footage would then be run through the software’s machine learning algorithm. This would have trained the algorithm to discern the sequences and patterns of 1’s and 0’s that, to the human eye, form the video of a combat zone as displayed in drone surveillance footage. The Pentagon has not publicly defined these 38 objects the software flags.
The surveillance team could then upload newly taken footage that is not labeled into Project Maven’s software. The algorithm behind the software would then be able to determine the contents of the footage and identify any anomalies or relevant objects it has been trained to flag. The system then alerts a human operator in some unknown fashion and highlights the flagged objects within the video display.
Orbital Insights offers a product that showcases the capabilities of machine vision when applied to satellite images. The company conducts geospatial analytics on space satellite images of large-scale areas. In addition, we can infer the CIA uses Orbital Insights currently because it received $20 million in funding from the In-Q-Tel venture fund in 2016.
The In-Q-Tel venture fund’s sole purpose is to fund companies developing products that it predicts could benefit American intelligence and military operations. Additionally, In-Q-Tel claims Orbital Insights is used by several US government agencies, although it has not commented on the identity of those agencies.
Below is a 3-minute video of Orbital Insight’s CEO showcasing the Orbital Insight’s software:
Satellite imaging allows operators to track target movement over large areas, determine normal patterns of activity for a location, and detect anomalies when those patterns differ. Orbital Insights links together a large amount of satellite imaging data from various networks to assemble high definition images of any location on the globe, taking the most useful pieces of each while removing clouds, smog, weather effects, or haze from the images.
Orbital Insights has written a case study on predicting China’s above-ground oil reserves. Orbital Insight’s computer vision software can identify oil tanks and then measure their capacity in order to calculate overall supply. They state, “The volume of the oil is visible because the tanks have floating roofs. Using our shadow detection techniques, we can gauge the size of the crescent-shaped shadow on the roof and calculate how full it is.”
The Trump administration has proposed more than tripling funding in next year’s budget to $83 million for just one of the AI-driven missile detection programs relying on military visual data.
Homeland Security
One core capability of artificial intelligence is identifying trends and patterns within a data set to then predict the likelihood of, and when, that trend will occur again. This is called predictive analytics, and it’s currently being applied to matters of homeland security.
Predictive analytics models can be used to correlate signs of preparation for unlawful activities, such as purchasing a weapon or makeshift bomb-making material at a store, which allows intelligence agencies to intercept the act before a plot unfolds. Predictive analytics software can also give a prediction of possible suspects of a crime based on various environmental factors and past criminal record data.
The CIA, FBI, LAPD and other US government organizations currently use Palantir as predictive analytics software. Below is a 5-minute video that describes how machine learning can pool message data, state identity data, charts, spreadsheets, telephone records, documents within a state database such as filed police reports, network data, sensor data, and full motion video to discern unseen patterns and aid in criminal investigation:
Pooling vast sums of data allows for the AI to find patterns correlative to unlawful action that data analysts may struggle to investigate and identify on their own. Starting in 2011, The US Marines integrated Palantir’s software during the War in Afghanistan to compile lists of possible bomb makers aiding terrorist cells.
According to Palantir’s case study, investigators detected correlations between weather data, command wire signals from IED attacks and linked biometrics collected off of explosive devices to individuals and bomb-making networks. As a result, they were able to deploy manhunting teams for specific individuals in order to reduce the number of IED attacks in a region.
We covered another predictive analytics software, Stabilitas, in our report on AI at the CIA. Stabilitas is a software that the CIA might use in order to gauge the stability and safety of a region in the world. Stabilitas seems able to make these predictions by crawling through social media posts, local news articles, and government reports for signs of regional destabilization.
Cybersecurity
We found that AI solutions for cybersecurity have a high degree of demand. This seems understandable for cybersecurity due to the high level of risk associated with the data breaches in military and defense networks. Several AI vendors and defense contractors seem to be using machine learning to offer security products that can identify and predict threats before they can affect the networks.
Given the volumes of collected data referenced in the last section as well as the danger of leaked government intelligence, cybersecurity seems to be a high priority for the US Army and Navy. Many of the cybersecurity solutions within this report can be utilized by private businesses as well; hackers of the world can just as easily target private entities for their secrets as they can militaries.
Cybersecurity threats come in numerous shapes and sizes. Artificial intelligence has the capability to play a large role in preventative measures for a military. Today, software is able to identify various digital situations, such as an email or a new flash drive, likely to be a trap or tool for implanting malware, and then neutralize the cyber threat lying in wait for a military operator before the malware can active.
BAE Systems is a London-based defense, security, and aerospace company. DARPA entered into a $5.2 million collaboration with BAE Systems to develop CHASE, software tools that can identify and predict cyber threats to large enterprise networks using artificial intelligence.
The CHASE software might first require labeled datasets which indicate the “normal” values for various metrics from internal network servers and non-AI intrusion detection systems. This would allow the software to identify a baseline reading for standard operating network conditions.
The software would then be trained to identify anomalies in the network characteristics due to previous security intrusions. Once the software “learns” what parameter values could come from an active threat, the system can be used to prompt human security analysts through a dashboard to ensure that all new security threats are identified at the earliest.
An example of CHASE’s capabilities or other such software using AI for cybersecurity may be a software that monitors the normal levels of download data that occur by each department per day. The software could indicate a potential threat to a security professional when a specific departments exceeds its normal daily download prediction
SparkCognition claims it uses machine learning in its DeepArmor solution to identify and analyze unknown files and detect cyber threats such as malware before it can breach military IoT networks. Remote devices such as laptops, other wireless and mobile devices, and IoT sensors are called “endpoints.”
These endpoints are highly vulnerable to cyber attacks, and the software could automatically monitor weapons systems, mobile devices, and aircraft. SparkCognition claims DeepArmor was used to catch and block several global cybersecurity threats, such as the WannaCry ransomware attack, Popcorn Time, and Adylkuzz.
A similar system of predictive analytics was designed by Cylance, a start up company funded by In-Q-Tel.
Below is a video showing how Cylance’s software could neutralize malware:
Cylance published a case study showcasing the value of Cylance Safelite reported. Safelife’s Matthew Coy, VP of Information Technology commented on the current capabilities of Cylance stating, “Cylance detected and stopped tens of thousands of events per day. Not one of them was noticed or acted upon by the existing anti-virus system.”
In the case study, Matthew Coy referenced the low administration effort required to manage CylancePROTECT has resulted in significant cost savings for Safelite. He estimated that managing their previous anti-virus product required 150 hours per week.
Logistics
As the saying goes, “Knowledge is power” and artificial intelligence has the capability to enhance the knowledge at the disposal of a decision-maker allowing them to make better, more informed decisions. There are large edges to be gained in an organization when it comes to the logistics and maintenance practices of those organization. AI has the capability to allow for more efficient, data-backed logistics and maintenance of military equipment.
Receiving Alerts and Report Generation
One facet of improving the logistics of an organization is improving the speed at which decisions are made and executed on. Conversational interfaces have the capability to increase the effectiveness and speed of an officer’s decisions.
Conversational interfaces allow officers to request a diverse variety of information related to the system it is installed on and have it accurately displayed before them or broadcasted to them without the need for human interaction and human error.
For example, the commander on the bridge of a battlecruiser while in battle could instruct the conversational interface, constantly listening for instructions, to immediately alert them when the ammunition of a specific battery reduces below 15%.
Knowing precisely when that threshold is achieved could allow for the commander to give orders to replenish the ordinance of that battery at a strategically optimal time.
Conversational Interfaces for military use have additional issues than for use with the public. They need to work with a high degree of certainty or else the drawbacks of error or inaccuracy could cost the lives of one or many operators. A system that does not properly deliver a scheduled report to a leader while in battle may cause the leader and the vessel they command to be caught severely off guard and put into a potentially dangerous scenario.
Performing Maintenance on Vehicles
As a military maintains numerous vehicles, those vehicles must be maintained when damaged in combat by the environment or through normal wear and tear. Given the immense number of craft that requires maintenance, any method that increases the efficiency to repair those craft would likely be welcomed. Artificial intelligence may have the capability to aid in the repair of vehicle fleets.
Lockheed Martin collaborated with NGRAIN (Canada) Corporation in big data analytics projects to improve the maintenance efficiency for the F-35 aircrafts used by the US Air Force. The project streamlined the capture of damage data, reduced costs, and enhance pilot safety through efficient maintenance operations.
According to a case study published by the Industrial Internet Consortium, Lockheed Martin currently produces the The F-35 Lightning II aircraft and also offers training, prognostics, and maintenance support services for the aircraft. In order to provide efficient maintenance, Lockheed Martin needed a way to accurately assess any damage to the aircraft hull and airframe due to combat or environmental hazards.
Traditionally, maintenance technicians assess airframe damage areas by placing a transparent film on affected areas and marking reference points on the film. The line drawings from the film are then cross-referenced with repair data history in a spreadsheet. Lockheed Martin maintenance engineers were finding this process tedious and time consuming.
Lockheed Martin developed the Autonomic Logistics Information Systems (ALIS) software suite for use with the F-35 and needed a way for its engineers to visualize damage reports in 3D with a high level of accuracy. The defense contractor used the Canadian AI company NGRAIN’s Software Development Kit (SDK) to generate a 3D virtual model of the aircraft.
According to the case study, maintenance technicians can use the NGRAIN tool to enter the aircraft’s unique tail number and pull up historical damage and repair data. The technician then enters the damage type and dimension details during each new maintenance cycle. This information is automatically stored in the ALIS software and can be retrieved in the future.
NGRAIN claims their 3D-modeling solution helped Lockheed’s maintenance engineers reduce maintenance time. The US Air Force additionally requested the damage reporting solution to be deployed on the F-22 Raptor. We’ve covered Lockheed Martin’s AI projects in greater depth in a previous article.
Autonomous Vehicles
Defense Undersecretary for Research and Engineering Michael Griffin stated that military operators performing logistic support runs account for a minimum of 50% of the casualties while at war. He said to lawmakers in Congress, “If that can be done by an automated unmanned vehicle with a relatively simple AI driving algorithm where I don’t have to worry about pedestrians and road signs and all of that, why wouldn’t I do that?”
As Michael Griffin eluded to in his commentary, the safety regulations and training of an autonomous vehicle for military purposes can be at a lower level of sophistication compared to autonomous vehicles being sold to the commercial public. When human lives are on the line, utilitarian calculations of value become a lot easier; essentially, an autonomous vehicle can operate with less regard for other drivers if its mission means saving the lives of one or more operators.
The theoretical capabilities of autonomous vehicles in the military are numerous as the military utilizes many wide varieties of vehicles. Numerous military defense contractors are researching autonomous cars and work trucks for use in military construction and logistics. As an example, an autonomous truck could travel back and forth from a sand silo while military laborers continue creating sandbags.
The Israeli Aerospace Industry is constructing an autonomous bulldozer, and a vehicle that transforms its dimensions to optimize for certain situations. Lockheed Martin has launched their Convoy Active Safety Technology system. CAST is a system which is installed on military trucks which, when activated can lock-on to the vehicle ahead of it and drive itself using machine vision, creating a convoy.
Boeing has designed and is testing autonomous spacecraft for routine satellite deployment. Boeing offers autonomous drones, and aircraft to militaries today and is designing autonomous submarines. We’ve covered a host of AI projects at Boeing in a previous article.
Below is footage of Northman Grumman autonomous jet conducting the world’s first autonomous mid-air refueling:
With the formation of the Joint Artificial Intelligence Committee (JAIC) by DARPA and the number of large military defense contractors with various autonomous vehicle projects in development, it is clear that development of autonomous vehicles is a priority for the US Department of Defense.
Additionally, the traction seen in the defense contractor industry seems understandable because vehicle autonomy allows for a productivity boost and an increase in human operator safety. As an example, autonomous vehicles able to patrol a secure area and investigate any signs of intruders by focusing its cameras on places of possible disturbance and then alerting human security forces of intrusion would result in a significant reduction in human patrolmen.
Those patrolmen would be able to then spend their time on more valuable tasks without the facility seeing a reduction in the quality of surveillance. A military may also see an increase in surveillance quality due to the removal of human operator factors such as the need to use the bathroom or patrolman distractions within their personal life.
Numerous companies are attempting to add autonomous capabilities to a variety of vehicles. Boeing is working on autonomous submarines. Both the Chinese government and the US DoD are developing kits of hardware and software that can be installed into different ground platforms, like tanks and armoured personnel carriers, to increase the level of autonomy, said Osie David, Chief Engineer for Mission Command, Communications-Electronics Research, Development and Engineering Center in the United States of America.
Our CEO, Daniel, has reported on the beginnings of the international competition between the US and China with regards to AI at the National Defense University in Washington DC as well as at a United Nations Conference in Shanghai. His presentation focused on the economic implications of artificial intelligence and national security.