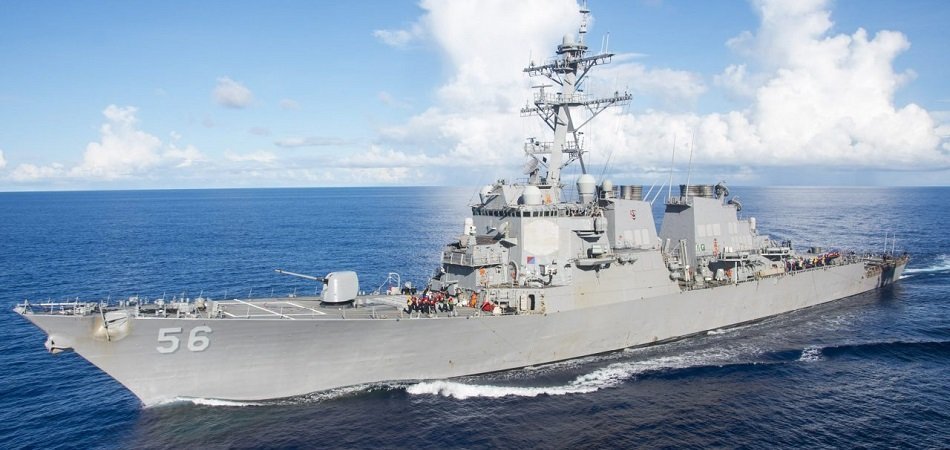
Militaries around the world are starting to make increasing investments in artificial intelligence and machine learning capabilities. The top military defense contractors in the US, Europe, and Israel are all working on AI software to sell into the defense sector. That said, the military adoption of AI is as of right now slow in comparison to that of contractors.
In this article, we’ll be covering the AI applications that military defense contractors intend to sell for use in the US Navy. Many of the applications covered in this report appear to currently be in the exploratory or testing phases.
The defense contractors discussed in this report offer software for the following use cases for artificial intelligence in the Navy:
- Alion Science & Technology and Hydroid – Entity Detection and Classification
- L3 Technologies and Raytheon – Precision-guided Munitions
- Lockheed Martin and Rite Solutions – Cross-platform Data Access and Analysis
We’ll run through each of these companies and their AI-based software, munitions, and vehicles one by one, starting with Alion Science & Technology:
Entity Detection and Classification
Alion Science & Technology
Alion Science & Technology offers Findr, which it claims can help the Navy detect and characterize entities using machine vision.
Alion claims the Navy can integrate the software into the navigation system of an autonomous vehicle to sense and track targets through obstructions in the working environment.
The company states the machine learning model behind the software was trained on traditional Doppler radar data showing the nature and activity of the entity during observation from various angles and in various lighting conditions. These images would have been labeled as targets or non-targets. These labeled images would then be run through the software’s machine learning algorithm.
This would have trained the algorithm to discern the sequences and patterns of 1’s and 0’s that, to the human eye, form the image of a target and non-target as displayed on the radar.
The user could then upload radar images that are not labeled into Findr. The algorithm behind the software would then be able to identify images representing targets from non-targets. The system then alerts a human employee of potential targets in the area in real time.
Alion lists GSA Federal Systems Integration and Management Center and the Naval Undersea Warfare Center as clients.
Chris Milroy is Director of Artificial Intelligence at Alion. He holds a BA in Economics, Philosophy from the University of Chicago. Previously, Milroy served as Chief Scientist of the Nascent Technology Center at Engility Corporation.
Hydroid
Hydroid offers the Remote Environmental Monitoring Unit System, or REMUS, series of autonomous underwater vehicles (AUVs), which it claims can help the Navy detect threats using machine vision.
Hydroid claims the Navy can integrate the REMUS software into existing intelligence and surveillance and reconnaissance systems above water.
The company states that the REMUS AUV would be able to detect and identify threats to depths of as much as 6,000 meters underwater.
Below is a short 4-minute video demonstrating how the REMUS works:
Hydroid claims to have helped the U.S. Navy find and destroy underwater mines. The Navy used REMUS AUVs together with land-based systems to launch mine countermeasure (MCM) exercises. According to the case study, Hydroid increased the Navy’s warfare capabilities by keeping adversaries “guessing.” We were unable to find any more tangible results for the application, although it would likely be difficult to quantify the results of such an application.
Hydroid also lists Microsoft co-founder Paul Allen and the Woods Hole Oceanographic Institution as some of their past clients.
Andrew Keefer is Software Engineer at Hydroid. He holds a BS in Physics and Physical Oceanography from the University of Rhode Island. Previously, Keefer served as Software Engineer at Teledyne Benthos.
Precision-guided Munitions
L3 Technologies, Inc.
L3 Technologies, Inc. offers the MK 332 Mod 0 High-Explosive, 4-Bolt Guided (HE-4G) projectile, part of the Advanced Low-Cost Munitions Ordnance (ALaMO) Guided Projectile program, which it claims can help the U.S. Navy and Coast Guard improve accuracy in hitting moving targets at longer range and less cost using machine vision.
The company claims the Navy and Coast Guard can integrate the software used to guide the “smart” projectiles into BAE Systems Mk110 systems. The company states the projectiles are for use with the Littoral Combat Ship and Fast Frigate of the Navy and the National Security and Offshore Patrol Cutters of the Coast Guard.
Below is a short 2-minute video demonstrating how the HE-4G works:
According to Navy Recognition, the HE-4G is still in development, although it has shown in demonstrations at the Potomac River Test Range that it can hit a moving target with more accuracy at longer ranges than standard projectiles.
L3 also lists NASA and Lockheed Martin as some of their past clients.
Megan (Cramer) Fillinich is VP of Engineering, Space & Sensors Sector at L3 Technologies, Inc. She holds a Ph.D. in Systems Engineering (Information Theory Research Focus) from George Washington University. Previously, Fillinich served as Chief Technology Officer at the Naval Surface Warfare Center.
Raytheon
Raytheon offers the Naval Strike Missile, or NSM, which it claims can help the US Navy and other defense organizations with over-the-horizon defense using an artificial intelligence-based guidance system. The precise AI-based technology is not specified, but the company claims the NSM has the ability to seek and identify targets under challenging conditions, perform “evasive maneuvers,” as well as climb and descend with the terrain, which likely indicates some type of machine vision.
Raytheon claims the Navy and other defense organizations can integrate the system into existing defense infrastructure as it is an “off-the-shelf solution that exceeds requirements for the over-the-horizon mission.”
Raytheon is a well-established manufacturer of missiles. We can infer the machine learning model behind the software was trained on long-range target-seeking and maneuvering images and video data collected from previous projects undertaken by the company from various angles and in various lighting conditions.
These images and footage would have been labeled as a target, hostile action, or natural terrain. These labeled images and footage would then be run through the machine learning algorithm. This would have trained the algorithm to discern the sequences and patterns of 1’s and 0’s that, to the human eye, form the image or footage of a target, hostile activity, or natural terrain as displayed in the tracking interface.
Since it claims the NSM is “off-the-shelf,” this indicates it does not require fresh input or data from new users to further train the machine learning system to find its target or execute evasive maneuvers. The company does not specify how the NSM handles any image or footage that has not been previously labeled. It also does not specify any human interference once the NSM is deployed, indicating that it is a fully autonomous system.
Below is a brief overview of Raytheon’s “Advanced Naval Strike Portfolio,” including the NSM:
Raytheon claims to have the means to help the Navy save a significant amount of money in development costs as the NSM is now fully operational. It claims that the NSM can easily integrate into the defense systems of any combat ship or frigate. According to the demonstration shown above, the NSM could be an effective weapon for over-the-horizon mission, with a range of over 100 nautical miles.
Raytheon also lists Norway-based defense company Kongsberg Defence and Aerospace AS as a partner in developing NSM.
Tod Newman is Leader of the Artificial Intelligence and Machine Learning Center of Excellence at Raytheon. He holds a BS in Marine Engineering from the US Coast Guard Academy. He has not listed any previous positions from other companies. However, he has been with Raytheon for over 21 years in various capacities, all related to software development, information systems, and machine learning with emphasis on missile systems.
Cross-platform Data Access and Analysis
Lockheed Martin Corporation
Lockheed Martin Corporation offers the Distributed Common Ground System (DCGS) Integration Backbone, or DIB, which it claims can help establish a common data infrastructure across the military and associated agencies using machine learning.
Lockheed Martin claims the Navy and intelligence agencies can integrate the software into multiple intelligence, surveillance and reconnaissance or ISR sensor systems worldwide with data from a variety of sources. The company claims the open standards interface of the DIB supports access, merge, and share intelligence data among DCGS users for more effective planning and decision-making.
The DIB is still under development. However, Lockheed Martin does provide a case study that it claims demonstrates the importance of data access across intelligence services and agencies. The client was the Distributed Mission Site USAF Warfare Center, which tasked Lockheed Martin to update the DCGS to allow access to the Nellie Air Force Base intelligence system. Second-party coalition forces required the access during aerial combat training exercises.
According to the case study, Lockheed Martin was able to reconfigure the security classification to allow the coalition forces to access the Geospatial Intelligence system at certain workstations and participate in combat exercises.
Lockheed Martin also lists the CIA and FBI as some of their past clients.
Sadananda Narayanappa is Chief Data Scientist at Lockheed Martin Corp. He holds a PhD in Mathematics & Computer Science from the University of Denver. Previously, Narayanappa served as a Data Scientist at Microsoft.
For more on AI at Lockheed Martin, read our full report: Lockheed Martin’s AI Applications for the Military – An Overview
Rite Solutions
Rite Solutions offers a software tool called Automated Protocol Translator or APT, which it claims can help interoperability efficiency by automatically generating the software needed to integrate new technology with legacy systems using natural language processing.
It is likely that users can integrate the software tool into existing systems to eliminate the need for custom software or middleware to integrate and access data from multiple systems using different platforms.
We can infer the machine learning model behind the software was trained first on hundreds of thousands of relevant messages and events defining different protocols, such as CORBA (Common Object Request Broker Architecture ), GPB (Google Protocol Buffer), and AMQP (Advanced Message Queuing Protocol) under various levels of translations from complex to simple. The machine learning algorithm behind the protocol translation tool would then automatically create code and other data to translates those messages and events into recognizable forms by different protocols. Human coders would then monitor these translations and correct any errors and feed this back into the machine learning algorithm.
This would have trained the algorithm to recognize and correctly transcribe these translation requests.
Then, the machine learning model would need to be trained on the appropriate translation according to the needs of specific clients. This would have required thousands of messages and event responses to these requests from multiple systems. This data would have been labeled as triggers for specific types of code and other data. The labeled text data would then be run through the software’s machine learning algorithm. This would have trained the algorithm to discern the types of messages and events from one protocol that a human might interpret as equivalent to corresponding messages and events in another protocol.
A customer could then integrate the APT tool into the existing system, and the algorithm behind the software would then be able to translate each message and event to and from another system automatically. The system might then come up with a confidence interval on how likely it correctly translated the message or event.
Below is a short 2-minute video demonstrating how the APT tool translates data and events from one system to another.
Rite Solutions claims to have validated the efficacy of the APT tool in promoting interoperability of digital systems. Rite Solutions integrated the APT tool into a simulated environment translating different types of real-world CORBA events to and from AMQP and GPB messages. According to the test, the APT tool performed as expected, correctly translating 2 million messages in 65 hours. The company claims the tool can translate a complicated message in a few milliseconds.
Rite Solutions also lists Coca-Cola, Boeing, and GE Healthcare as some of their past clients.
Ian Mitchell is Senior Software Engineer at Rite-Solutions. He holds an MS in Computer Science from the University of Rhode Island. Previously, Mitchell served as a Software Engineer at Raytheon.
Header Image Credit: Safety4Sea