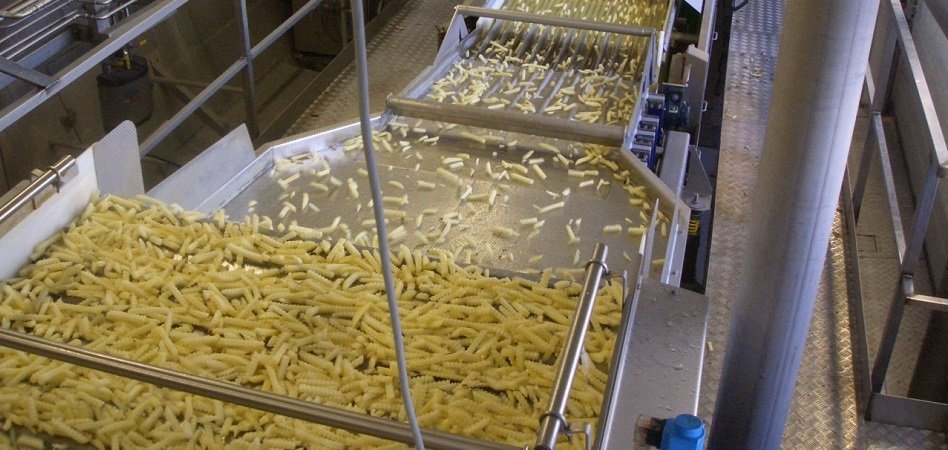
Although it’s apparent that AI development is slow-moving in the industry, food and beverage companies may be able to use AI for food processing, in particular:
- Sorting and Quality Control
- Food Production
Machine vision seems to be the most common AI approach with use in the food and beverage industry. Food processing equipment vendors offer machine vision software in tandem with their equipment, claiming they can automatically sort out poor quality food and non-food items from assembly lines and/or conveyor belts on which food travels through the machinery systems.
Other vendors offer software for optimizing ingredient mixtures to prevent wasteful use of ingredients and low-quality food and beverage batches. We’ll begin by looking at sorting and quality control:
Sorting and Quality Control
Tomra Systems ASA
Tomra Systems ASA offers a line of food sorting machinery with analytics capabilities which it claims can help food manufacturing companies perform automated food analysis, such as measuring the size, shape, and color of french fries or analyzing the fat content in meat using shape recognition technology, a subset of machine vision.
Tomra claims that clients can integrate the POM/DYN french fries length analyzer with their own existing french fries production machinery.
We can infer that the machine learning model behind the software was trained on images of french fries of different sizes and shapes from various angles and in various lighting conditions. These images would have been labeled as french fries of the correct length, for instance between 3 to 4 inches, and those that are too short or too long.
These labeled images would then be run through the software’s machine learning algorithm. This would have trained the algorithm to discern the sequences and patterns of 1’s and 0’s that, to the human eye, form the image of a french fry of the correct length and those that are too short or too long, or one that is discolored and possibly contaminated, as displayed on in an image on a dashboard.
The machine operator could then run the POM/DYN camera over the actual french fries, and the algorithm behind the software would then be able to discern the pieces that are between 3 to 4 inches, which are accepted on the main stream of the machinery. The pieces that do not meet the specified length are redirected to another stream. Through the LDC display, the system shows the machine operator a single random sample of a french fry that passed the shape, color, and length standards and compares it with a sample of a french fry that is rejected.
Below is a short 2-minute video demonstrating how Tomra’s sorting equipment is able to discern the correct shape, length and color of a french fry and separate fries that do not meet the specifications:
Tomra claims to have helped Agristo in Portugal update its food processing plant in 2014 and eliminate bottlenecks due to slow outdated machinery. However, limited space and sustained capacity meant Agristo did not need and could not install larger machinery. Agristo, which produces frozen French fries and other potato products, chose to deploy Tomra’s optical sorter in the Agristo facilities in Belgium and The Netherlands.
The case study reported that Tomra upgraded an existing sorting machine that inspects the frozen fries before they are packed, adding that Tomra replaced the cameras, computer, software, but kept the frame, the belt, and the manifold.
According to the case study, the project took only four days for each facility, one day of which was for training Agristo’s personnel. Agristo also reported that the plant did not encounter any problems once the machinery was put in production, and helped the company sustain its more than 140,000-ton production.
For 2015, Agristo had also planned to consign another project to TOMRA for a sorting machine for its Netherlands plan.
Tomra also lists Sun Valley Raisins, TANN Marchtrenk, Mrs. Gerry’s Kitchen, Hata Foods, Morada Nut, Salaisons Jouvin, and Kadi as some of their past clients.
Volker Rehrmann is the Executive Vice President and CTO at TOMRA. He holds a PhD in computer science from the University of Koblenz. Previously, last name of the person served as title of the person’s position at name of marquee company or leadership at an AI-focused company.
Key Technology
Key Technology offers a line of sorting machinery, including the Manta Digital Camera/Laser Sorter, which it claims can help vegetable packaging companies keep food quality and safety by removing foreign materials from fresh farm produce such as green leafy vegetables, corn, green beans, and peas using shape recognition technology.
We can infer that the machine learning model behind the software was trained on thousands of images of farm produce and foreign materials showing the differences between good-quality produce from poor-quality produce and dirt clods, insects, sticks, small rocks, and cotyledons from various angles and in various lighting conditions. These images would have been labeled as good and bad quality produce.
These labeled images would then be run through the software’s machine learning algorithm. This would have trained the algorithm to discern the sequences and patterns of 1’s and 0’s that, to the human eye, form the image of good and bad produce. It is not clear if the system has a dashboard that shows single object inspection.
The machine operator could then start moving the actual farm produce on the conveyor, as the camera captures images that are not labeled into the software. The algorithm behind the software would then be able to distinguish the shape and color of the good produce from the substandard produce and foreign materials.
Below is a short 2-minute video demonstrating how The Manta 2000 sorts the quality corn kernels from substandard pieces:
Key Technology claims to have helped Pure Pacific Organics (PPO), a processor of organic fresh-cut products such as baby spinach and spring mix maintain product quality and safety. PPO deploy Key Technology’s Optyx with FluoRaptor, a fluorescence-sensing laser sorter. According to the case study, the machinery is able to detect and remove defective, extraneous vegetable matter, and foreign material based on the levels of chlorophyll as well as color, size, and shape.
The machine features a 48-inch (1220-mm) wide scan area where up to 6,500 pounds (3 metric tons) of product could be sorted per hour. The machinery is also fitted with a top-mounted laser, two top-mounted Vis/IR cameras, and two bottom-mounted Vis/IR cameras to view products from the top and bottom.
As a result, the case study claimed PPO is experiencing nearly 100 percent product efficiency, has decreased labor costs while maintaining production targets.
Key Technology also lists Eisberg Group, The Clements & Son Ltd, Pizzoli, Quebec Wild Blueberries, Emblème Canneberge, Antarctic Foods, and Nut Company as some of their past clients. The company was acquired by Duravant on January 2018 for $175 million.
Louis Vintro is the Senior Vice President of New Products and Business Development at Key Technology. He holds a PhD in physics from Stanford University. Previously, Vintro served as Director, eMarket Solutions at Vitria Technology and Senior Director, Technical Programs at KLA-Tencor.
Food Production
Rockwell Automation
Rockwell Automation offers a manufacturing production analytics application software called FactoryTalk Analytics, which the company claims can help a variety of industries, including food and beverage companies, leverage data to save time, prevent errors, reduce energy consumption, improve quality, increase efficiency, and provide access to self-serve analytics to relevant staff using machine learning.
Rockwell Automation claims that food and beverage companies can integrate the software into manufacturing and business systems.
The company states the machine learning model behind the software was trained on both structured and unstructured data from devices, sensors, tags, and business systems that might relate to the production process, asset function or administrative processes. The data would then be run through the software’s machine learning algorithm. This would have trained the algorithm to discern which data points correlate to production and capacity, a measure of ingredients, energy consumption or condition of machines.
The software would then be able to predict the optimal production capacity, correct amount of ingredients, minimum energy consumption, or preferable machine maintenance dates, among others. This may or may not require the user to upload information about their plans for increased production or new formula for the food recipe, etc into the software beforehand.
Below is a short 3-minute video demonstrating how FactoryTalk Analytics can access any type of data from within the internal enterprise database and ingest it to create predictions:
Rockwell Automation claims to have helped Miraka, a dairy processing company implement the Pavilion8 solution in phases to introduce model predictive control technology and meet regulatory requirements, consumer demand, product quality, and rising costs. To improve performance and profitability, Miraka invested in Rockwell’s application to optimize the milk powder process.
After the evaporation process was deployed in phase 1, the second phase implemented the spray dryer model predictive control, which enabled the company to improve production through closed-loop moisture control to determine the optimal amount of water required by the milk powder product. Capacity was also increased within the limits of safety.
The third and final phase of the project then implemented a multivariable model predictive control to track the liquid composition through the drying process of the milk powder product and standardize the process. The result of this was better consistency in the protein and fat content of the final product, and less use of standardizing ingredients.
Rockwell Automation also lists DuPont, Kraft Heinz, Galbusera-Tre Marie, Caffè Borbone, AFA Systems, Hydro Tasmania, Arnott’s, Henshaw, Arkansas Steel, Vreugdenhil, Deyu, Tomatic, and Lotte as some of their past clients.
Sujeet Chand is the Senior Vice President and Chief Technology Officer at Rockwell Automation. He holds a PhD in electrical engineering from the University of Florida. Chand has served at Rockwell for 17 years.
Header Image Credit: Wikipedia