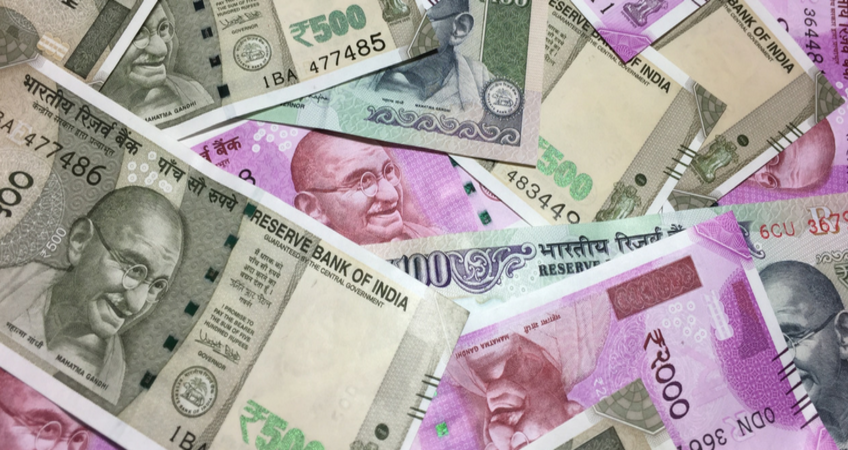
The banking and financial sectors are among the top five applications for artificial intelligence across the globe. With India seeming to have woken up to the potential of AI, we decided to cover the developments in the Indian artificial intelligence sector. We then researched the use of AI in the Indian financial services market to better understand where AI comes into play and to answer the following questions:
- What types of applications is AI being applied to in the Indian financial sector?
- What tangible business results have been achieved by Indian banks and financial institutions by applying AI?
- What opportunities and trends for AI in finance do Indian business executives need to be aware of?
This report covers companies and institutions offering and using software across four applications:
- Credit Scoring and Loan Management
- Fraud Detection
- Risk Management
- Financial Transactions
This article intends to provide Indian business leaders in finance an idea of what they can currently expect from AI in their industry. We hope that this report allows business leaders in retail to garner insights they can confidently relay to their executive teams so they can make informed decisions when thinking about AI adoption. At the very least, this report intends to act as a method of reducing the time business leaders in retail spend researching AI companies with whom they may (or may not) be interested in working.
Credit Scoring and Loan Management
Loan Frame
Loan Frame was founded in 2015 in New Delhi and has around 36 employees. Loan Frame is a fintech company that claims to be using AI to originate, underwrite, and distribute small business lending products by creating a lending marketplace to connect small and medium enterprises (SMEs) with lenders.
Loan Frame provides secured and unsecured loans ranging from 5 lakh to 50 crore for small and medium-sized businesses. The company claims to be using machine learning to give small businesses insights into which loans to take out, such as how much to borrow and from whom.
The process by which the machine learning system takes user data and turns it into insight is unclear. We do not know if the software requires an in-house data scientist to operate or not.
We can infer from Loan Frame’s website that small and medium-size businesses can start a loan applications process on their website and input a number of relevant details, such as the purpose for the loan, the financial history of the small business, and information about other existing loans the business might have.
Loan Frame’s machine learning model might then use the financial history of the small business and cash-flow analysis to create a borrower risk profile. Additionally, the company’s machine learning software might also crawl through social media data, online footprints, and eCommerce data to supplement these risk profiles.
The startup also claims to be developing behavioral and psychometric scores that would help assess customers who have little or no credit history. We could not find a demonstration video available detailing how the company claims to be using machine learning.
Loan Frame exclusively uses their machine learning software internally, and so there are no available case studies for the software.
Loan Frame raised a seed round of $2.25 million and is backed by Parag Saxena, co-founder of Vedanta Capital, William Campbell, former chairman of Visa International and Toos Daruvala, co-chief executive of MIO Partners, an internal investment arm of global consultancy McKinsey and Co.
The company claims to have helped 20,000 customers, including small businesses, and has 10 unnamed lending partners across 175 locations in India. There does not seem to be any C-level executives on the team with an AI background. We caution readers to be wary of companies that claim to do AI without any C-level AI experts on their team.
Capital Float
Capital Float was founded in 2013 in Bangalore and has 491 employees. The company’s website seems to suggest that they use machine learning internally to automate loan underwriting and to deliver financing solutions for SMEs across eCommerce, manufacturing supply chains, retail, travel and hospitality, and digital remittances.
Capital Float offers unsecured business loans to small businesses by collecting data, such as a business’s value-added tax (VAT) returns for the last 12 months, income tax returns, Know Your Customer (KYC) documents for the applicant and the business, bank statements for the last siz months, and loans or overdraft sanction letters.
The company claims their internal software uses machine learning to track loan repayments and identify potential defaults.
Capital Float states a significant portion of the company’s small-ticket size loans are completely automated in terms of risk profiling and underwriting. The company also claims that when the risk prediction is not within reasonable accuracy levels (predefined by the company), their software can defer to human loan officers on what must be done for a particular application.
For example, if the ticket sizes for the loans are too high or if the customer has very little credit history, the software may send an alert to human loan officers for a review. We could not find a demonstration video available showing how the software works, likely due to the fact they use it internally. As such, there are also no available case studies for the software.
The company has raised funding from marquee investors such as SAIF Partners, Sequoia India, Aspada Investments, Creation Investments Capital Management LLC, Ribbit Capital, and Amazon.
The company’s Chief Growth Officer, Tushar Garimalla, seems to have previous experience in AI projects for risk management with HSBC Bank and in portfolio management with Capital One. We could find no evidence of the company’s executive leadership having an AI background.
Fraud Detection
National Stock Exchange of India Ltd (NSE)
The NSE recently announced that they have made efforts towards using machine learning to identify market patterns that might lead to improving monitoring efforts on the exchanges and preventing manipulation of its high-frequency trading (HFT) markets.
The exchange’s CEO Vikram Limaye stated their aim is to develop a system that can help human security officers make better-informed decisions and provide better outcomes faster. He added that NSE also hopes to apply AI to their historical trading data and derive insights on markets and clients to potentially provide trading recommendations to financial advisors and to detect fraud.
Limaye also states that the NSE currently does not have the data scientists for the ML adoption and is focusing on partnerships with regulators, member institutions, and other capital market players. NSE also recently organized a hackathon attended by over 37 teams and 250 developers from data science backgrounds in an effort to kickstart their AI program.
At this stage, it is unclear whether the NSE has started a pilot project. We could also find no further details on how the NSE’s machine learning system might function in terms of inputs and outputs.
Risk Management
The Bombay Stock Exchange
The Bombay Stock Exchange (BSE) introduced a data analytics solution it claims can track social-media-shared news related to companies listed on the exchange.
The Exchange claims they use machine learning to detect and mitigate potential risks of market manipulation and rumors. The press release from the BSE stated the software can provide information, such as the reactions of investors to news media or the impact of rumors involving listed companies on BSE through the exchange’s website for the benefit of investors.
The software alerts human security officials at BSE so they can compare possible rumors appearing in print to those in online channels, as per Securities and Exchange Board of India (SEBI) regulations. When the human security officials spot a difference between the online news and the news in print media, the companies are required to justify the reasons to a BSE review board.
For instance, if news about a large business deal about to occur is found through alerts from the software in social media data and other print media suggests no such deal might occur for a period of time, then the involved companies are required to give a reason to the BSE for the non-alignment in news media. The BSE then disseminates the business’ provided reasons on the Exchange website.
We could find no more information on what the inputs and outputs for the machine learning software used by BSE were. There were also no details made available by the BSE relating to tangible results for their software’s performance.
Dulal Mali is the Big Data Analytics Practice Lead at BSE Limited and was previously the general manager of BSE for 15 years. He also worked on data science projects with the BSE, such as leading big data HADOOP implementations and a business intelligence system implementation using IBM Smart Analytic System.
Financial Transactions
Niki.ai
Founded in Bangalore in 2015 with over 71 employees, Niki.ai states on its website that it has developed a chatbot called Niki. The company claims Niki guides customers of finance, eCommerce, and retail businesses with recommendations to reach the right service. It could also potentially make transactions for the customer.
For example, their chatbot might be integrated within the website of an online retailer in the form of an app or Facebook Messenger’s chat interface to improve customer service. The chatbot could then help the bank’s customers search, make payments, and complete transactions through conversations on the messaging interface.
Niki.ai claims their chatbot can perform tasks such as topping off a pre-paid mobile phone with more minutes, booking a cab, assisting with online shopping. Niki is available on Android, Messenger iOS, and some website platforms. After a brief integration period, the chatbot application can potentially help brands offer around 20 services, which form the backbone of what Niki can offer businesses.
Below is a short 2-minute video demonstrating the Niki chatbot:
According to a case study from Niki.ai, the company worked with HDFC Bank to help the bank discover additional sales channels for their banking services. Niki.ai also claims that after a brief integration period, they developed the HDFC Bank OnChat, which was launched on Facebook Messenger.
Individual customers of HDFC bank could then search for “@hdfcbankonchat” on Facebook Messenger and start using the services offered through chat.
Although Niki.ai claims their chatbot integration takes a very short time (less than a day), and it indeed might be closer to the plug-and-play spectrum of AI products, we could not find more information on what was involved in the integration nor how many and what types of employees from both companies were involved in the integration for HDFC bank.
The company is backed by Ratan Tata, the former Chairman of Tata Sons, and recently secured a $2 million round of Series A funding.
Apart from HDFC Bank as mentioned above, NIki.ai also seems to have worked on a chatbot project with Federal Bank to create a virtual assistant for the bank’s smartphone application called FedMobile.
We could find no history of previous AI experience among the company’s executive leadership team.
Key Insights for Business Leaders in the Indian Finance Sector
We found the following trends emerge as the most important for business leaders to be aware of before engaging in AI projects in India.
- Scarcity of trained AI data scientists
- India might still have a relatively small number of good data scientists available for AI work compared to the US or China. Industry collaborations with universities in India to develop skilled data scientists and in-house training programs might be one way to stay ahead of the curve on this challenge.
- Availability of the right kind of data
- Unverified data is a serious concern for any AI project, and business leaders can in a rudimentary sense think of AI systems as garbage in, garbage out systems. For example, in a KYC compliance AI system, if the data input about the customers is incorrect, an AI system would output incorrect results. Businesses need to plan for collecting, validating, standardizing, correlating, archiving, and distributing AI-relevant data.
- Over 100 languages spoken throughout the country
- Natural language processing (NLP) applications for AI generally tend to use existing software libraries and techniques. What this means for banks and financial institutions is that AI can be used to support a few major Indian languages, but support for all of them may take a while.
- Data Privacy and Security
- The introduction of regulations in Europe, such as GDPR (General Data Protection Regulation), might be a precursor to what Indian banks might need to follow while building AI systems in terms of privacy regulations.
Header Image Credit: english.samajalive.in