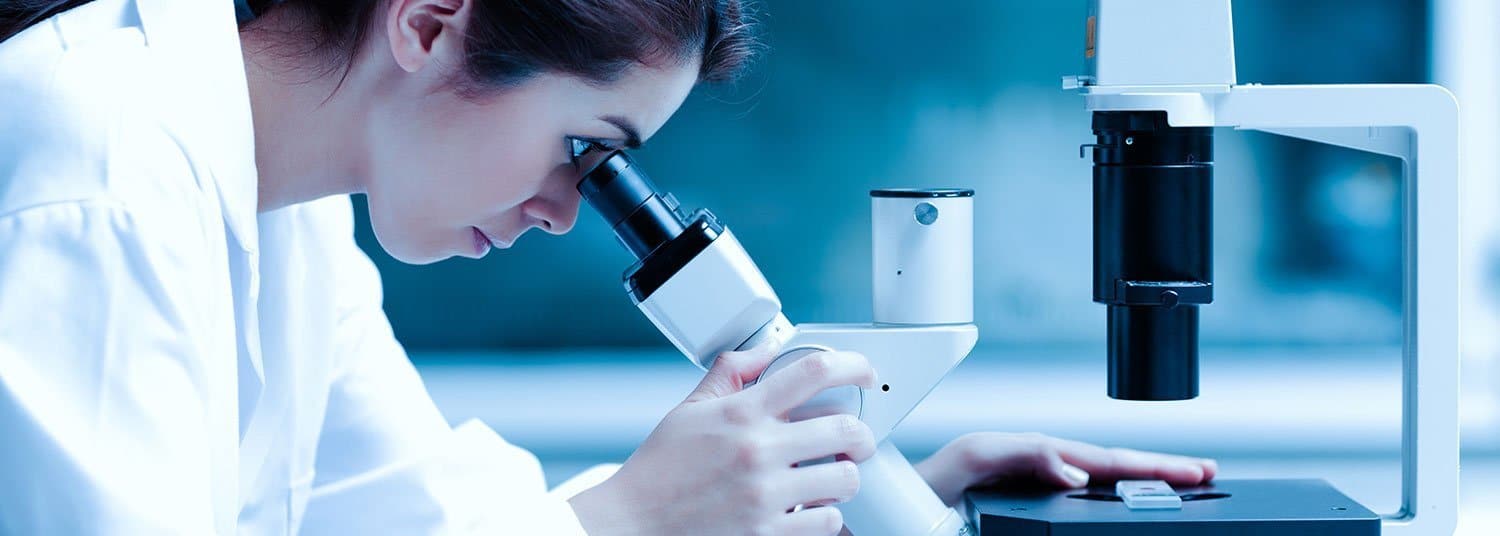
AI and machine learning software are beginning to integrate themselves as tools for efficiency and accuracy within pathology. According to a March 2018 digital pathology report produced by Allied Market Research, such software enables the “procurement, management and interpretation” of information and can be applied to multiple functions within the field of healthcare.
In addition to providing functional support for practitioners, students and even patients, interest in applied AI is garnering growing profits; the global market for this technology was valued at more than $3.3 million in 2016, and researchers predict this total will rise to $8.6 million by 2026.
Software is being developed by startups–often in tandem with prominent educational institutions or large hospital research laboratories, addressing different diseases and conditions, most notably forms of cancer. Real-world applications include, but are not limited to:
- Intraoperative diagnosis
- Training
- Primary diagnosis
- Disease prevention
- Manual and semi-quantitative review of immunohistochemistry (IHC)
- Diagnostic consultation and decision support
- Clinical research
After reviewing the functionalities of AI and machine learning software in the field of pathology, we found they generally belong in one of the following categories:
- Whole slide imaging, analysis and diagnosis
- Tissue genomics and other prevention technologies
- Companion diagnostic devices
Considering this software’s potential to disrupt the healthcare industry and individual health care markets, this report will: identify the myriad of ways in which AI is impacting this health sector; survey the functions of this technology and provide industry case studies of these applications; and draw conclusions regarding its profitability and scalability of AI beyond studies and product development.
Note: Companies working within digital pathology software often collaborate with other businesses in this field to provide more accurate, multi-functional software solutions. When applicable, we will highlight these collaborations in the categories below, in addition to further discussing the implications of this “merging” in our conclusion.
Whole Slide Imaging, Analysis and Diagnosis
Philips + Inspirata
A majority of AI and machine learning software within pathology strives to create databases of digitized tissue samples in high resolution, allowing pathologists to digitize many of their routine processes. Common to all software in this space is the ability to scan biopsied tissue samples into a system, wherein these images can be annotated with measurements and diagnoses.
Most software uses a deep learning function, meaning the software identifies associations between the collected images and works to create ever-changing algorithms for diagnosis. For example, a pathologist may scan in a slide and through the imaging software, identify a specific cancer cell within that digitized tissue sample, and note this within the image by selecting the cancerous area. The software will use this diagnosis and search for other annotated images that look similar, creating data criteria that will pair like images in the future and associate them with a specific cancer status (i.e., benign, malignant).
The following video from the healthcare company Philips’ YouTube channel gives an extensive, but approachable overview of how pathologists are integrating AI and machine learning software into their workflows to cut down work time, reduce misdiagnoses and connect with fellow pathologists–in general, making their departments more cost-effective and high functioning.
Mark Lloyd, PhD, the founder, lead scientist and executive VP at Inspirata, a biotechnology company that produces this type of software, says this about the increased adoption of AI software within pathology:
“Digital pathology enables us to collect precise numbers about pathological images, which enables our scientists to delve into the numbers, delve into the values of morphological or molecular changes, and then correlate that information back with patient data, which gives pathologists the power to not only contribute to precision medicine, but to drive precision medicine.” (Source)
Beyond precision, another problem this software attempts to address is the ability to store petabytes of data, which is necessary for continued refinement of diagnostic algorithms and collaboration between multiple medical institutions.
In March 2015, Philips and Inspirata announced their joint development agreement, promoting this SaaS as a product of Philips’ clinical informatics capabilities with Inspirata’s service model. This collaboration is indicative of the many companies who are partnering to better their own respective software with the technological capabilities of outside companies.
Looking at specific products within the two companies, first: Philip’s Intellisite Pathology Solution software is “an automated digital pathology image creation, viewing and management system comprised of an ultra-fast pathology slide scanner, an image management system and a display.” Within this system are tools to perform the variety of functions mentioned above, including scanning, storage, presentation, review and sharing of data.
TissueMark, the system’s computational pathology tool, purports to use deep learning AI technology to accumulate information from several hundred images and apply that knowledge to accurately identify tumor samples and estimate tumor percentages. The algorithms used in this software specifically focus on identifying lung and breast cancers.
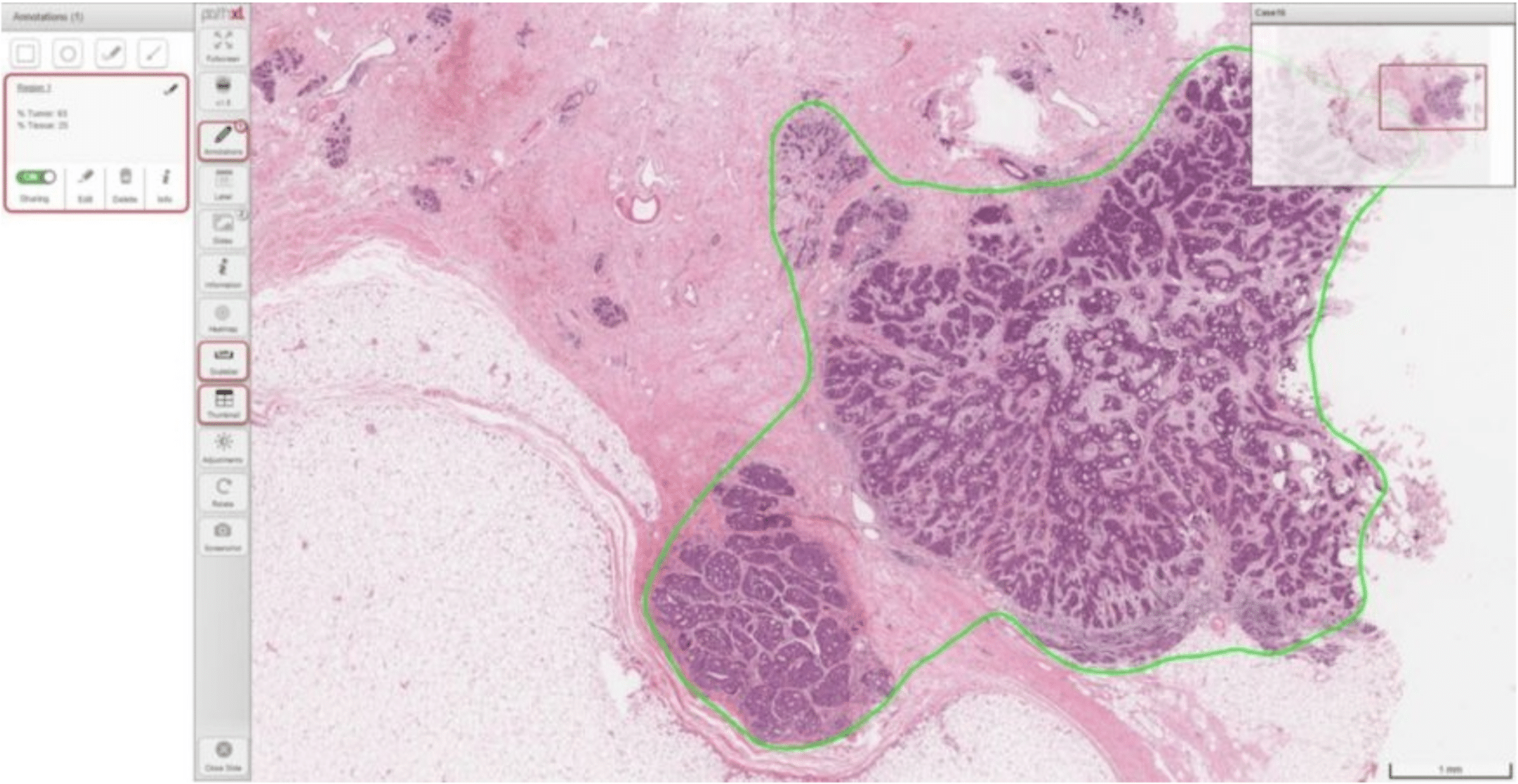
Phillips does not readily provide a cumulative list of hospitals and companies with which it has partnered on its site, nor does it identify specific hospitals using the IntelliSite software (beyond AZ Sint-Jan hospital featured in the video above).
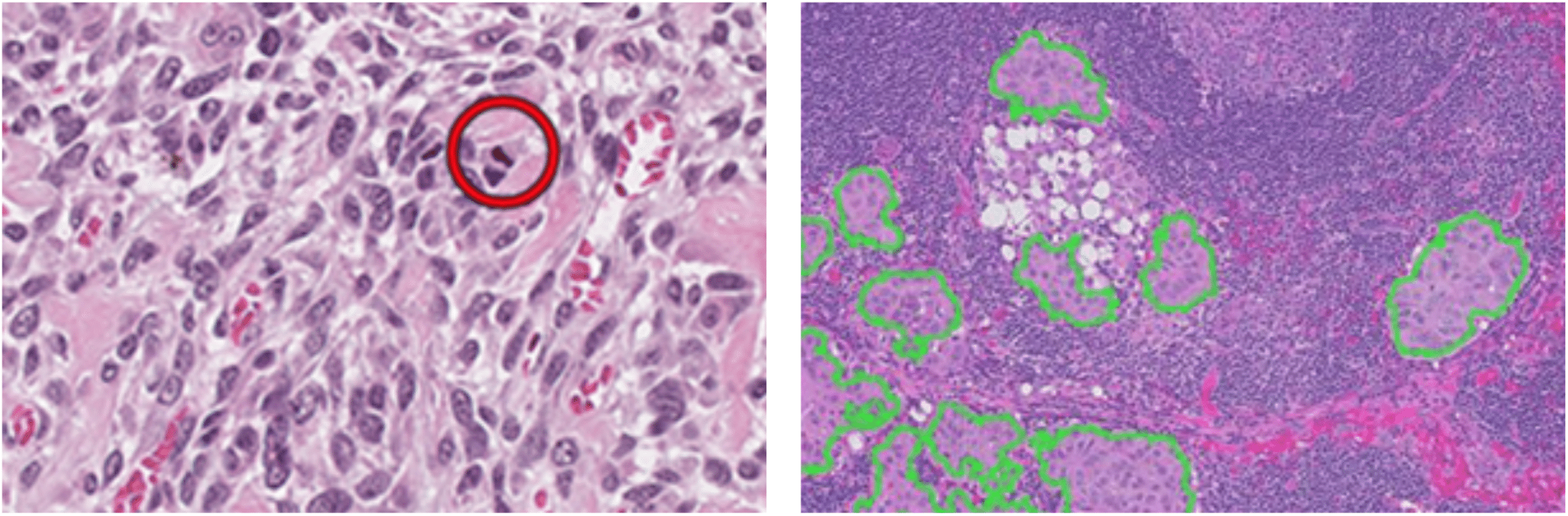
Visuals of mitoSĒK™ and histoSĒK™ software identifying potentially problematic areas of digital slides, respectively (Source: Inspirata website)
Second, partnering with Philips, Inspirata has two pieces of software directly pertaining to digital pathology: mitoSĒK™ and histoSĒK™. mitoSĒK™ is a first-pass magnifying tool that identifies “all high-likelihood mitotic figures,” that is split cells that have a high likelihood of being malignant growths. It is meant to serve as a tool to save pathologists’ time by instantly highlighting potentially problematic areas of the scan, instead of having to do this manually, without magnification.
histoSĒK™ claims to automatically identify and quantify regions of a scanned slide which may contain harmful growths. The Inspirata website gives sample use cases for this software: microdissection, necrotic area quantification or tumor burden measurements.
Inspirata also markets workflow management software, a consultation portal, a data repository and its platform, through which users can connect with colleagues and other pathologists. In addition to partnering with Philips, Inspirata lists seven other companies and association with which it partners. It does not readily provide measurable successes or its number of clients; however, it does have video testimonials from doctors and other clients using the technology.
Tissue Phenomics and Other Prevention Technologies
Definiens
Another up-and-coming application of AI in pathology is tissue phenomics, which helps in immunotherapy, finding ways to use the body’s immune system to treat diseases. Definiens, a biotechnology company, brands itself as “The Tissue Phenomics Company.” It draws a comparison between traditional therapeutic strategies, which “direct[ly] assault cancer,” and and the potential of treatments based in phenomics, which measures changes in physical and biochemical traits as they respond to genetic mutation and environmental influences.
Definien’s mission, according to the website, is to help scientists leverage tissue phenomics to better understand how the immune system can help cure disease and to apply this data in creating individualized care for cancer patients.
One way in which they do this is by gathering “multi-omics data,” which includes information from the genome (genes), proteome (proteins), transcriptome (RNA; nucleic acid), epigenome (chemicals affecting the genes) and microbiome (microorganisms). Essentially, more data sets are measured within a biopsy slide.
Like the previously discussed software facilitating whole slide imaging, analysis and diagnosis, Definiens software purports to integrate into current workflows and serve as a “science-driven partner.” It’s highlighted functions are:
- Quantifying tissue sample data to identify biomarkers, substances indicative of cancer
- Integrating multi-omics data with clinical outcomes, identifying patterns
The company’s two promotional videos, both from 2012 (one of which is featured below) does not give details of how these expanded data sets better facilitates immunotherapy; in fact, Definiens technology is modeled similarly to previously mentioned software. This may be because the overview videos are several years old, and processing phenomic data might be a recently-added process. It is also notable that the co-founder of Inspirata was featured as a research scientist working with Definiens software at the time.
Definiens does not readily list its clients or evidence of profitability on the website; however, the company is very active in publishing articles, white papers and videos on the benefits of digital pathology, and claims it “has been used in thousands of projects, yielding results that have accelerated drug development and produced over 650 peer-reviewed publications.”
The company’s position on the role of AI in the field of pathology is definitively of the opinion that technology is a tool, rather than a substitute, for human pathologists, and that the best diagnostics and treatment come from the two working in tandem.
In Vitro Diagnostic (IVD) Devices
IDx LLC
Most recently introduced to the market, IDx is the first autonomous, AI-based diagnostic system authorized for commercialization by the FDA. This specific device is marketed for those people with a risk for developing diabetic retinopathy, a complication of diabetes that may lead to blindness. IDx claims the device “instantly detects disease,” and that the can easily be administered at a doctor’s office. The video on the IDx websites gives a simplified explanation of the device and how it is used.
Deep learning software searches for lesions specific to the complication by processing each uploaded image through a series of filters; according to their website, the filters evaluate for specific biomarkers associated with diabetic retinopathy.

As the device was only very recently authorized for sale, no specific users of the device are listed; however, the website presents ample data on research studies and clinical trials. According to its website, IDx “demonstrated 87% sensitivity and 90% specificity at detecting more than mild diabetic retinopathy” in a 2017 U.S. clinical trial with 900 subjects.
Additionally, the operators who administered the test were “novices” who hadn’t had prior experience with this type of photography (of the patients’ eyes).
IDx partnered with two universities, in addition to the Iowa Institute for Biomedical Imaging and the UI Research foundation, to build its AI technology.
Readers with a particular interest in diabetes should explore our full machine learning in for diabetes management article.
Future Profitability of Applied Digital Pathology Software
As evidenced by the companies above who represent applied AI in the field of pathology, there is plenty of potential for these technologies to be adopted in the near future; however, these software solutions, for the most part, will be more applicable and profitable for large and established research laboratories, hospitals with large caseloads, and educational institutions affiliated with prominent hospitals.
In these instances, profitability would result from saved time, more accurate diagnosis, scalability and the opportunity to offer more individualized treatment plans, like those determined through tissue phenomics. Additionally, access to data from multiple institutions could be attractive to research firms and hospitals looking to partner with businesses who use this software.
Smaller hospitals who have a low number of caseloads and small pathology departments may not benefit from utilizing this software regularly as part of their workflow; however, they may benefit from establishing a relationship with a larger hospital with this software, to whom they could refer patients who need a second opinion or further testing.
As shown in the examples we covered, many software companies specializing in pathologic solutions continue to partner with other software companies in this space, along with research institutions and large hospitals, in order to mutually benefit from each other’s technological capabilities, particularly in AI applications. We predict this trend will continue and serve as a means to visibility within the market.
Most of the technology we found dealt with diagnosis of prominent cancers (see our full article on deep learning in oncology), which are of great interest to both the medical community and the public at-large. We predict that these technologies will eventually be applied to tracking, diagnosing and curing other diseases; however, funding may be an issue if institutions are not willing or able to focus research on other areas beyond breast cancer, diabetes and the like.
Header image credit: University of Maryland School of Medicine