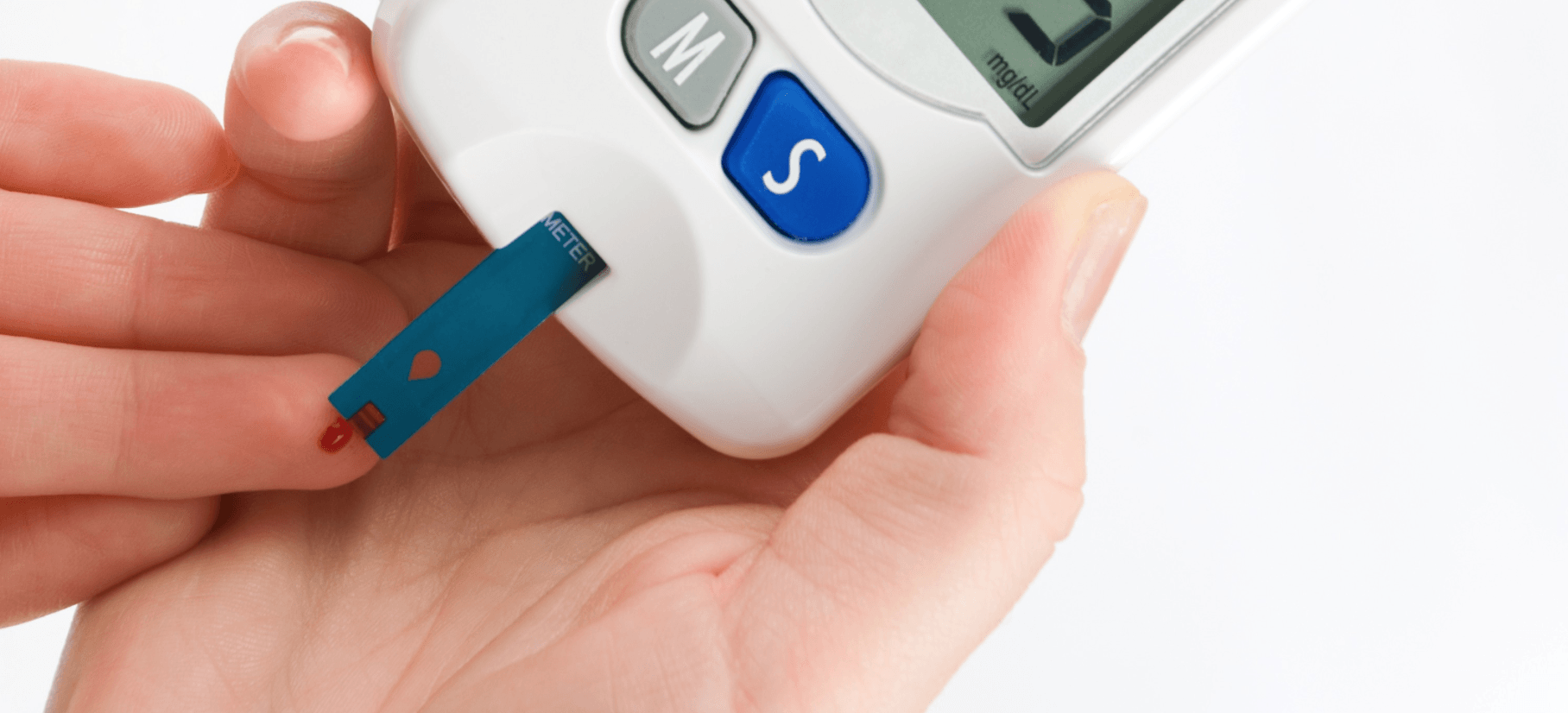
Diabetes is a leading chronic disease that affects more than 30 million people in the United States. The disease results from high blood glucose (blood sugar) due to an inability to properly derive energy from food, primarily in the form of glucose. Insulin is a hormone that normally helps process glucose in the body. However, in the case of diabetes, insulin is inadequate (Type 2 diabetes) or obsolete (Type 1 diabetes).
The economic impact of diabetes is estimated at $105 billion, according to a study published in 2016 and led by researchers from Imperial College London, Harvard T.H. Chan School of Public Health and the World Health Organization. Managing diabetes normally involves a commitment to a healthy lifestyle, routine checking of blood sugar levels and adherence to a medication regimen. Today, machine learning is emerging in an effort to improve the quality of life for diabetics.
Some of the questions business leaders are asking today related to the role of machine learning in managing diabetes include:
- What types of AI applications are currently used to manage diabetes?
- How has the market responded to these AI applications?
- Are there any common trends among these innovation efforts – and how could these trends possibly contribute to reducing the rates of people living with diabetes?
Diabetes AI Applications Overview
The majority of AI use-cases for managing diabetes appear to fall into three major categories:
-
- Glucose Monitoring Systems: Machine learning algorithms help automate the process of monitoring blood sugar levels and recommend adjustments in care.
- Nutrition Coaching: To help recommend meal options based on the specific diet criteria of the user.
- Early Diagnosis Tools: Deep learning to predict the onset of diabetic retinopathy, the leading cause of vision loss among diabetics.
Glucose Monitoring Systems
DreaMed Diabetes – Machine Learning for Optimal Insulin Dosages
Founded in 2014, DreaMed Diabetes claims its DreaMed Advisor cloud-based analytics platform uses machine learning to recommend optimal insulin dosages to maintain balanced glucose levels.
For example, data from diabetes management systems such as glucose monitoring devices and insulin dose regimens are transmitted to the cloud. Advisor can then access the cumulative data from the cloud and learn the patient’s unique habits and needs using a proprietary algorithm. The patterns derived from analysis are referenced to provide automated recommendations for insulin dosing.
This animated 1:58 minute video shows how Advisor works for patients and their providers:
The clinical trials database provided by the U.S. National Library of Medicine indicates that DreaMed began recruiting participants in December 2016 for an evaluation study in children and adolescents with type 1 diabetes. The post was last updated in November 2017 and the study is expected to run through December 2018, when final data will be compiled.
Medilync’s “Insulync” Helps Monitor Patients’ Blood Sugar
Founded in 2012, the Iceland-based startup Medilync claims its glucose monitoring device leverages machine learning to continuously monitor patient blood sugar levels. The company’s Insulync device is built with a glucose reader and includes space to store a user’s insulin pen (device used for insulin injections) and test strips (used for blood samples).
Data collected by Insulync is automatically synced to Medilync’s cloud platform called Cloudlync. Machine learning is reportedly used to analyze the data that is continuously fed to the cloud. Patient data is also integrated with meal plan data as well as a patient’s electronic medical record (EMR), preventing manual data entry.
These features allow for continuous, remote patient monitoring by clinicians and the ability to identify any potential health risks based on real-time glucose levels. The system also provides alerts if a user misses an insulin injection or a glucose reading.
This video shows how the platform captures data to monitor patient glucose levels:
Currently, the two case studies featured on Medilync’s website focus on how the company uses Microsoft solutions such as Azure machine learning algorithms to optimize its functionality. In fact, Microsoft seems to have taken a particular interest in the startup.
In November 2017, Medilync was honored as Startup of the year by Microsoft Iceland. Prior to that, in June 2017, the startup was selected to participate in Microsoft’s IoT & AI lab to continue to scale up its platform. However, results on how Medilync’s platform is impacting the health of its users is yet to be reported.
Nutrition Coaching
Suggestic Uses AI to Recommend Meal Options
Founded in 2014, California-based Suggestic is taking a nutrition-focused approach to helping diabetics and others living with chronic diseases manage their health. The startup claims that its app uses AI to recommend meal options that comply with the diet restrictions of the user.
According to the company’s website, the platform is built on an extensive database of over 1 million recipes and 500,000 restaurant menus.r This data is used to train algorithms to recognize which food selections complement specific diets.
For example, users begin by selecting a diet plan such as vegan or gluten-free. Using natural language processing the app’s chatbot assists users with planning weekly meals by recommending recipes or menu options when dining out. The platform uses an Adherence Score scale ranging from green (optimal) to red (least optimal) to determine how well a meal option fits a user’s diet. The app also reportedly integrates augmented reality (AR) to capture restaurant menu info using a smartphone camera.
In the brief 38-second video below, the company demonstrates how restaurant menu options are logged and scored in the app:
Aside from general reviews, specific data on diabetic user outcomes is not currently available on the company’s website. It does appear, however, that there is a strong interest in attracting partners such as diet and nutrition professionals, corporate wellness entities and research institutions. Current partners include the Institute for Functional Medicine and the Health Coach Institute.
Virta Health – Machine Learning to Individualize Treatment
Founded in 2014, Virta Health describes its platform as an “online specialty medical clinic” with an ambitious goal to “reverse diabetes in 100 million people by 2025.” Virta Health uses a nutrition-centered approach to help users adhere to a ketogenic (low-carb/high-fat) diet. The startup claims that it uses machine learning to individualize treatment recommendations and to scale its operations.
For example, Virta provides clients with devices to record information such as blood glucose and blood pressure. Patients enter their data into the Virta app, which can then be continuously monitored by the assigned clinician and health coach (who is available via text messaging or videochat).
Machine learning is used to learn the patient’s specific needs and can recommend videos on recipes and general diet tips based on these patterns. Patients can also indicate their general level of hunger, energy and cravings throughout the day, helping the care team integrate findings from data and inform patient care.
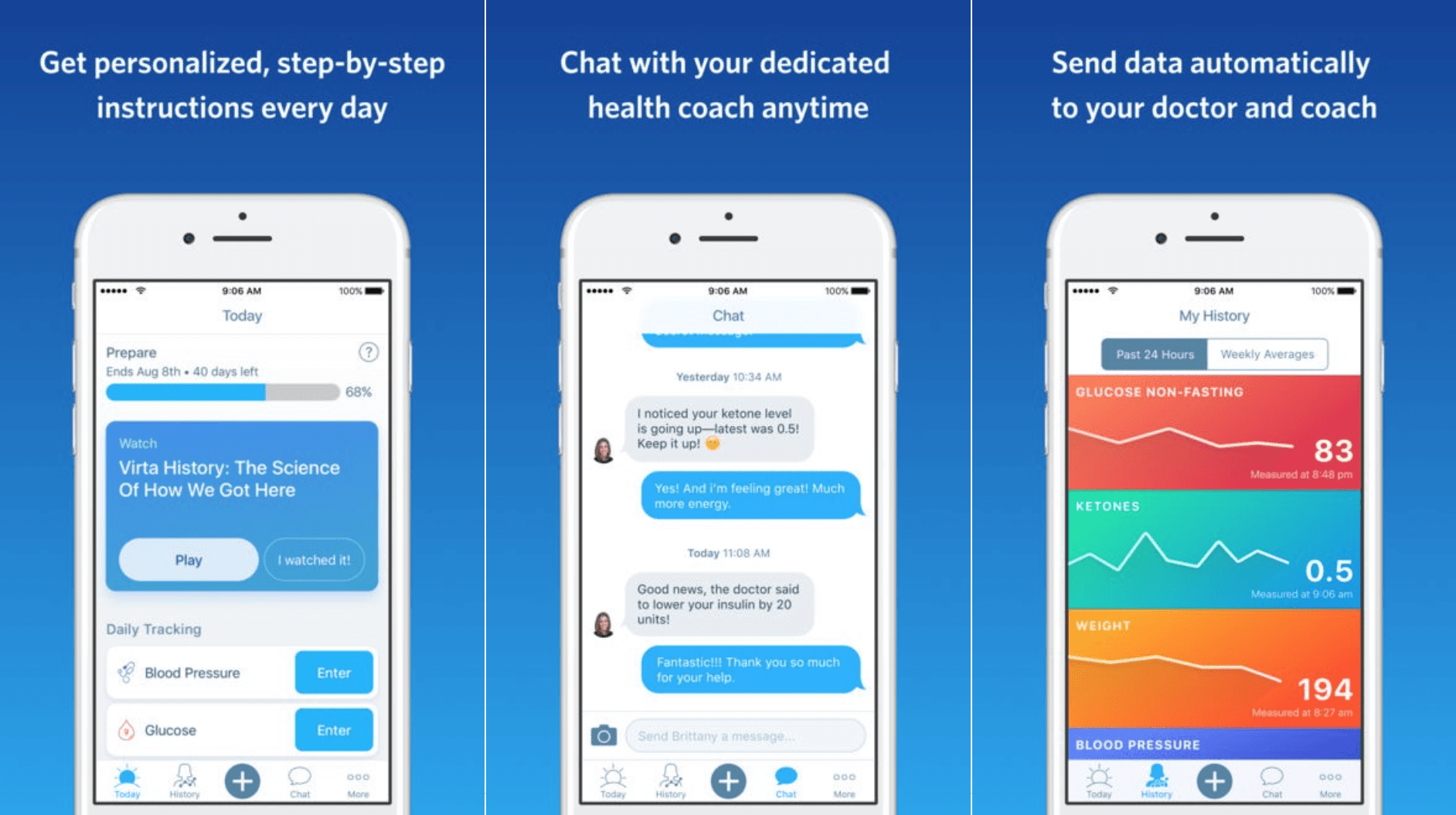
Based on preliminary findings from a 10-week clinical trial conducted in partnership with Indiana University Health, researchers indicate that 56 percent of approximately 240 participants lowered their blood sugar below diabetic levels and 87 percent no longer required insulin injections. Follow-up studies will help to validate if the reported outcomes are replicable across patient population and sustainable over time.
Early Diagnosis Tools
DreamUp Vision Uses Deep Learning to Screen for Eye Diseases
Diabetic Retinopathy (DR) – a form of vision loss caused by diabetes – has been documented as the leading cause of blindness in adults aged 20-74 years. Early screening is crucial but is currently outpaced by increasing rates of diabetes and an anticipated shortage of over 100,000 physicians by 2030, including eye specialists.
Founded in 2016 in Paris, DreamUp Vision claims that its software leverages deep learning to help automate screenings for eye diseases that may cause blindness.
DreamUp Vision’s algorithm was trained on a dataset of over 70,000 labeled retinal images. The images were rated by ophthalmologists on a scale from 0 (no retinopathy) to 4 (most severe). As a result, the algorithm is reportedly able to quickly identify the location and degree of severity for retinal abnormalities.
In the 2:54 minute below, members of the DreamUp Vision team discuss how the software works in a clinical setting with a demonstration:
According to results from a research study, DreamUp Vision’s algorithm achieved significant accuracy with a receiver operating characteristic (ROC ) score of 0.946. An excellent ROC score typically ranges from 0.90 to 1.
Diabetes AI Applications to Complement Physician Care
Diabetes management, to a large degree, involves pattern recognition thus positioning it well for applications of AI. For example, key factors such as blood glucose, weight and blood pressure must be consistently measured and monitored to inform patient care.
By automating routine processes, clinicians can devote less time to data entry and more time to patient interaction. Patients may also gain an increased sense of control over their diabetes by having coaching tools and support at their disposal.
The real potential for AI is the ability to scale up these innovations while still personalizing the user experience. Additionally, a better continuity of care may be achieved between face-to-face visits with physician and patient.
Challenges for market penetration include data privacy, patient consent and the current limited data on clinical results. Strategic partnership with hospitals and healthcare systems in general will be necessary to conduct the research required to validate company claims regarding products.
An important theme that emerged from our research is that these applications are not supplementing but rather complementing care delivered by clinicians. This is an important consideration going forward as more applications emerge in an effort to reduce the rate and negative impacts of diabetes.
Header image credit: Clinical Asiesta