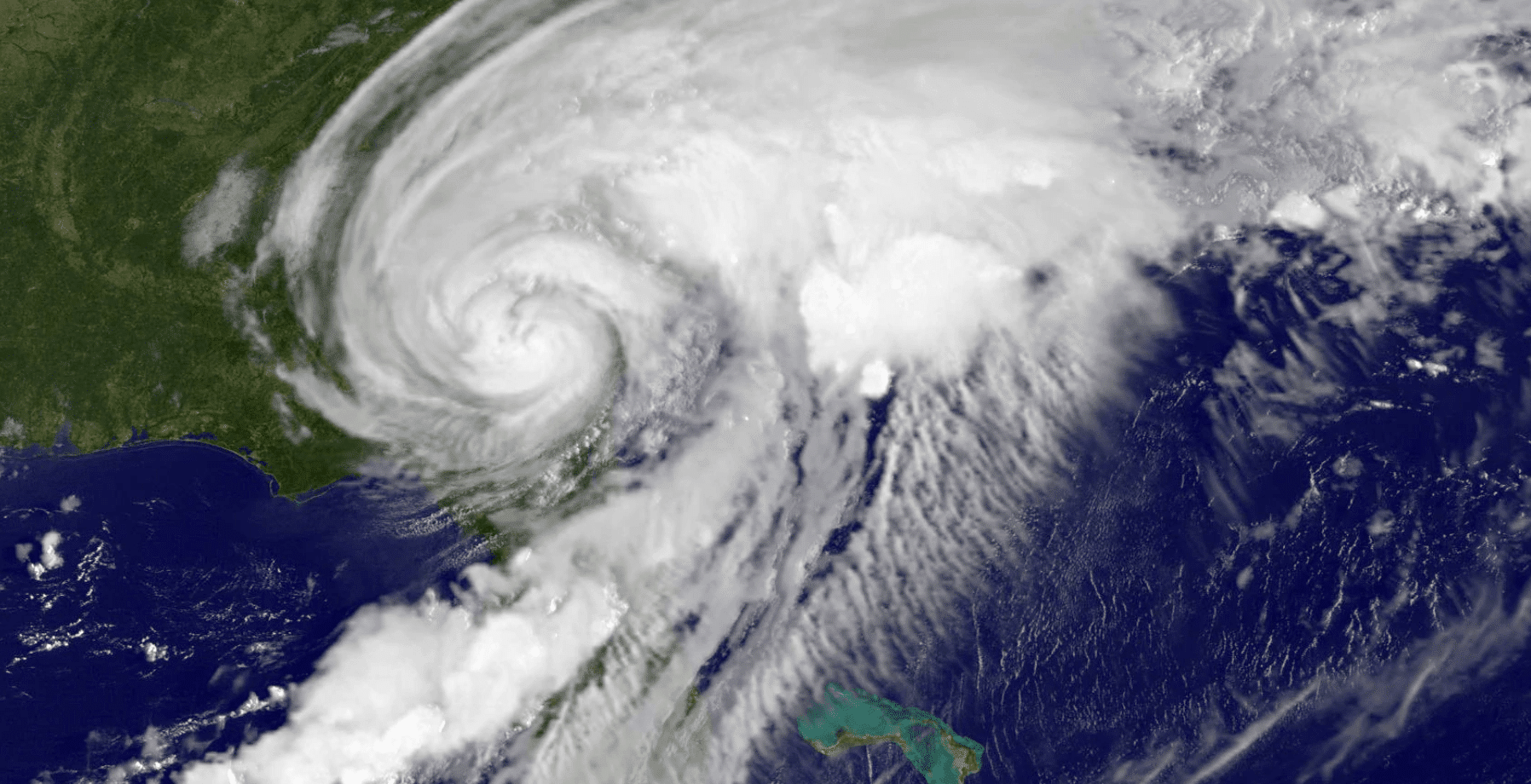
For most people, the direct impact of improvements in weather forecasting may seem to be that it simply makes vacation planning easier, but even smallest advancement in predicting the weather can produce massive improvements for businesses and governments.
More accurate weather forecasting allows farmers to make critical decisions about planting and harvesting. It allows airlines to maximize the use of their planes. It makes shipping easier and safer. Utilities can better make decisions about their capacity needs during heat waves or large rainfalls. As we recently saw with the hurricanes this year, it allows governments and businesses to better prepare their responses to natural disasters that impact the lives of millions.
This article will look at how big data and machine learning are transforming weather forecasting and what it means for businesses and governments. In this article we’ll explore:
- How companies and government agencies are using AI to improve weather forecasting (including IBM, Panasonic, and the US Government)
- Sector-specific machine learning applications for improving business performance (including Retail, Agriculture, Transportation)
Weather forecasting is a strong fit for machine learning. The incredible volume of relevant information — historical data and real-time data — that can be analyzed is simply too great for any group of unaided humans to even begin to process on their own.
Expanding Sources of Weather Data
The scope of the weather-related data available is positively massive. There are over a thousand weather satellites in space currently providing a wealth of data about cloud patterns, winds, temperatures, etc… These satellites are only one small part of the data production taking place. There are hundreds of thousands of government and private weather stations on Earth, constantly gathering real-time data. For example, the company Weather Underground, owned by IBM, claims to have access to 250,000+ personal weather stations, which can provide them with real-time information.
In addition, as cheaper sensors and better connectivity expand the accessibility of the internet of things (IOT), the number of devices and pieces of equipment that can provide useful real-time information about the weather will likely expand dramatically. Every car, truck, solar panel, connected traffic light, cellphone, smart household air conditioning systems, etc. could be used as a source of real-time information for improving forecasting.
For example, GE Current has installed smart street lights in several cities that can monitor things like light, humidity, and air quality. There are currently over 300 million street lights in the world and only a tiny fraction are smart. While it will likely be a long time before most street lights are connected, it should provide a glimpse of how just a single IoT development can radically increase the number of sources of hyper-local weather information.
The video below highlights the uses and applications of smart streetlights in the city of San Diego:
Organizations Applying AI to Weather Forecasting
Government – United States National Oceanic and Atmospheric Administration (NOAA)
Government institutions are still the major players in weather forecasting, both as a source of raw data used by private companies and as a source of predictive models. The Department of Commerce found that in 2014 all federal spending on meteorological operations and research was $3.4 billion while private sector spending on weather forecasting reached only $1.7 billion.
The US National Oceanic and Atmospheric Administration (NOAA) has recently been using machine learning more to improve their forecasts. A group of researchers from the NOAA found that “applying AI techniques along with a physical understanding of the environment can significantly improve the prediction skill for multiple types of high-impact weather.” High-impact weather includes events like severe thunderstorms, tornadoes, and hurricanes.
Their paper concluded these improvements have clear commercial applications stating, “AI methods extend easily to directly predicting impacts of high-impact weather, such as power generated by variable sources such as solar or wind, energy consumption in an area, or airport arrival capacity.”
One example the paper highlighted is that machine learning can provide more accurate hail forecasting. Hail cause billions of dollars damages every year. Even a modest improvement in hail warning could produce significant savings by getting individuals move their cars and themselves to safety. Provide these types of warnings for car insurance companies is one way IBM is commercializing their weather predictions.
IBM
Among companies using AI to predict the weather, few have invested so heavily as IBM. The company first got involved in trying to use their computer systems to improve forecasts in 1996 and have been refining their project ever since. In 2016 IBM closed on their purchase of Weather Company’s properties including weather.com, Weather Underground, The Weather Company brand and WSI, its global business-to-business brand.
“The Weather Company’s extremely high-volume data platform, coupled with IBM Cloud and the advanced cognitive computing capabilities of Watson, is unsurpassed in the Internet of Things,” said John E. Kelly, IBM’s senior vice president, cognitive solutions and research.
The acquisition gave IBM access to the Weather Company’s impressive network of sensors and models, providing a massive pipeline of weather data it could feed into IBM’s AI platform Watson to attempt to improve predictions. In 2016 the Weather Company claimed their models used more than 100 terabytes of third-party data daily.
The product of this merger is IBM Deep Thunder. The system provides highly customized information for business clients by using hyper-local forecasts – at a 0.2 to 1.2 mile resolution. Information useful for transportation companies, utility companies, and even retailers.
Below is an IBM video explaining how they are using big data to improve weather forecasting and making use of that information.
Earlier this year IBM announced a collaboration with University Corporation for Atmospheric Research (UCAR) and the National Center for Atmospheric Research (NCAR) using IBM supercomputers. The goal is to create first model to cover the entire globe instead of using just regional weather models, which leave some areas of the planet underserved. More accurate weather forecasting in these previously underserved area will make it easy for businesses to make decision about these locations.
Panasonic
Panasonic has been working on its own weather forecasting model for years, and it stepped up its effort with the purchase of AirDat in 2013. The company makes TAMDAR, a speciality weather sensor installed on commercial airplanes.
Panasonic’s supercomputers combine public data with the exclusive data it gets from thousands of flights a day. The company unveiled Panasonic Global 4D Weather last year, claiming it is the “ world’s most advanced global weather forecasting platform<”
While Panasonic is relatively new to commercial weather forecasting, they did score a big public relations win this year with Hurricane Irma. Their predictions about the storm turned out to be the most accurate of any model, particularly in determining where the storm would head four to seven days in advance.
In the video below Panasonic explains how they use TAMDAR to improve weather predictions:
Better weather forecasting allows airlines to adjust their routes to reduce fuel use, improve safety and increase on time arrives.
Monsanto – Climate Corporation
In 2013 Monsanto bought Climate Corporation for just under $1 billion. Among the services Climate Corporation provides, one if its main focuses is hyper-local weather forecast information for farmers. It uses a variety of sources and machine learning to optimize weather predictions specifically for agriculture.
In the video below, Brad Colman -Climate Corporation director of Science and Climatology – explains the numerous weather projects they give farmers:
To help enhance its forecasting and analytics, Monsanto acquired HydroBio, an agriculture software company based in Denver, CO. HydroBio uses a combination of satellite imagery, soil data and hyper-local weather data to deliver irrigation insights for farmers. It lets farmers better know when they should or should not irrigate. This prevents farms from wasting limited water resources and can improve yields. These tools will eventually be integrated into Climate Corporation’s Climate FieldView™.
Climate Corporation sells FieldView plus as a service to farmers. Currently it cost $749 for a one year subscription and they plan to release the pro version in October of 2017.
Uses of Weather Forecasting
Agriculture
The benefits of improved weather forecasting for agriculture are obvious, making farms a major customer of private forecasting companies. Weather determines the best time to plant, fertilize, spray, irrigate, and harvest crops. Having accurate weather information about every part of a field can allow farmers to maximize their yield.
Michael Stern, president of Climate Corporation, likes to point out that in competitions it is possible to produce 521 bushels of corn per acre with intensive management. By comparison, the national average is just 175 bushels per acre. The potential for improvement with better data, analytics, and management are dramatic.
According to IBM, 90 percent of crop losses are due to weather events and 25 percent of weather-related crop losses could be prevented by using predictive weather modeling. Weather forecasting doesn’t just help grow crops but also with their immediate shipping. Many rural roads around the world are dirt, and knowing when these road are dry enough to use is important for farmers. Over the past few years extreme weather has forced the US Federal Crop Insurance Program to pay out indemnities ranging from $3.5 billion in 2016 to 17.5 billion in 2012.
In the video below IBM explains how it is using Deep Thunder in agriculture
Transportation
General weather forecasting has improved shipping and transportation in numerous ways by making it easier for ships to avoid storms, but accurate hyper-local data combined with machine learning is allowing companies to squeeze extra efficiency out of vehicles.
According to IBM, weather related delays cost the freight industry approximately $8.7 billion a year. To try to reduce these loses this year IBM Operations Dashboard for Ground Transportation, their business tool for freight companies to help optimize productivity based on IBM’s weather data about road conditions.
The company Safety Line is using Panasonic’s weather forecasting to optimize the climb profile for commercial aircraft. They claim their system can reduce fuel consumption by up to 10 percent during ascent. To put that in perspective, US airline carriers spent $24.6 billion on fuel last year.
Disaster
Better weather forecasting saves lives and helps speed up rebuilding efforts. Hurricane Harvey was one of the most destructive storms to hit the United States in recent years, and the death toll currently stands at 82. By comparison, the equally strong 1900 Galveston hurricane killed between 6,000 to 12,000 people. Improved weather forecasting is partially to thank for this dramatic improvement.
IBM has started combining their weather forecasting tools with information about utilities’ distribution networks and data about local ground cover for severe storms. Using machine learning, they are predicting likely outages. IBM claims they deliver damage predictions that are 70 to 80 percent accurate 72 hours before the storm is expected. This allows utilities to better position crews before the storm hits so they can improve the speed of repairs afterwards.
Weather related outages cost the country between $25 billion and $70 billion annually. Even modest improvements in the speed at which outages can be fixed not only help the utilities’ bottom line but benefit the whole economy.
Retail
Weather impacts how people feel, travel, and spend their money. Rain can cause a surge in delivery orders. A decrease in temperature might cause a noticeable reduction iced coffee purchases, which could create waste if a company didn’t properly prepare. While we’ve covered a good deal of applications of machine learning in retail, but this article is our first focus on the factor of weather.
These are obvious examples, but machine learning is bringing some surprising ones to light. Walmart found that for some reason people want berries on calm days when the temperature is below 80 degrees. By targeting ads in locations where these specific weather conditions existed, the company tripled berry sales.
Two ice cream brands use IBM weather services to improve their sales. IBM found that not only were warm temperatures associated with increased ice cream sales but also colder temperatures as people sought comfort foods. IBM claims their weather insights lead the brands to increase sales by 26 percent and reduce waste by 35 percent.
The more data companies gather about how weather impacts people’s decisions, the better retail companies can plan. In addition, the better the weather forecasts, the more lead time companies have to prepare to take advantage of these patterns.
Concluding Thoughts
Governments and companies invest billions of dollars into weather forecasting every year for good reason. There is effectively no sector of the economy which is not directly or indirectly impacted by the weather.
The potential source of weather-related data will continue to grow dramatically and the new advances in machine learning are making it possible for government agencies and companies to make better use of all this data. Weather forecasting can never be truly perfect, but AI will allow the practice to continue to improve in its accuracy and in its resolution.
There will be no one pivotal movement when the industry is changed but just a continuous series of improvements. Even modest advantages can make a big difference when weather impacts so much of the economy. An extra day or even an hour of warning about a weather event can make a difference to farmers, airlines, utility companies, rescue crews, etc.
Improving and hyper-localizing weather forecasts enables numerous sectors to squeeze out extra efficiency — a small reduction in irrigation in part of a field, a slightly more efficient flight path for an airline, a few hours faster at fixing an outage.
Perhaps the most interesting thing about machine learning in weather forecasting is how it is being combined with other data about human behavior. We have only begun the understand all the subtle ways the weather impacts our choices from what food we eat to which entertainment we enjoy. The more refined and localized our weather information gets, the easier it will be to find distinct patterns and connections. Even small improvements in weather forecasting will give companies new useful pieces of data by finding new correlations and giving companies more lead time to take advantage of them.
Header image credit: The Washington Post