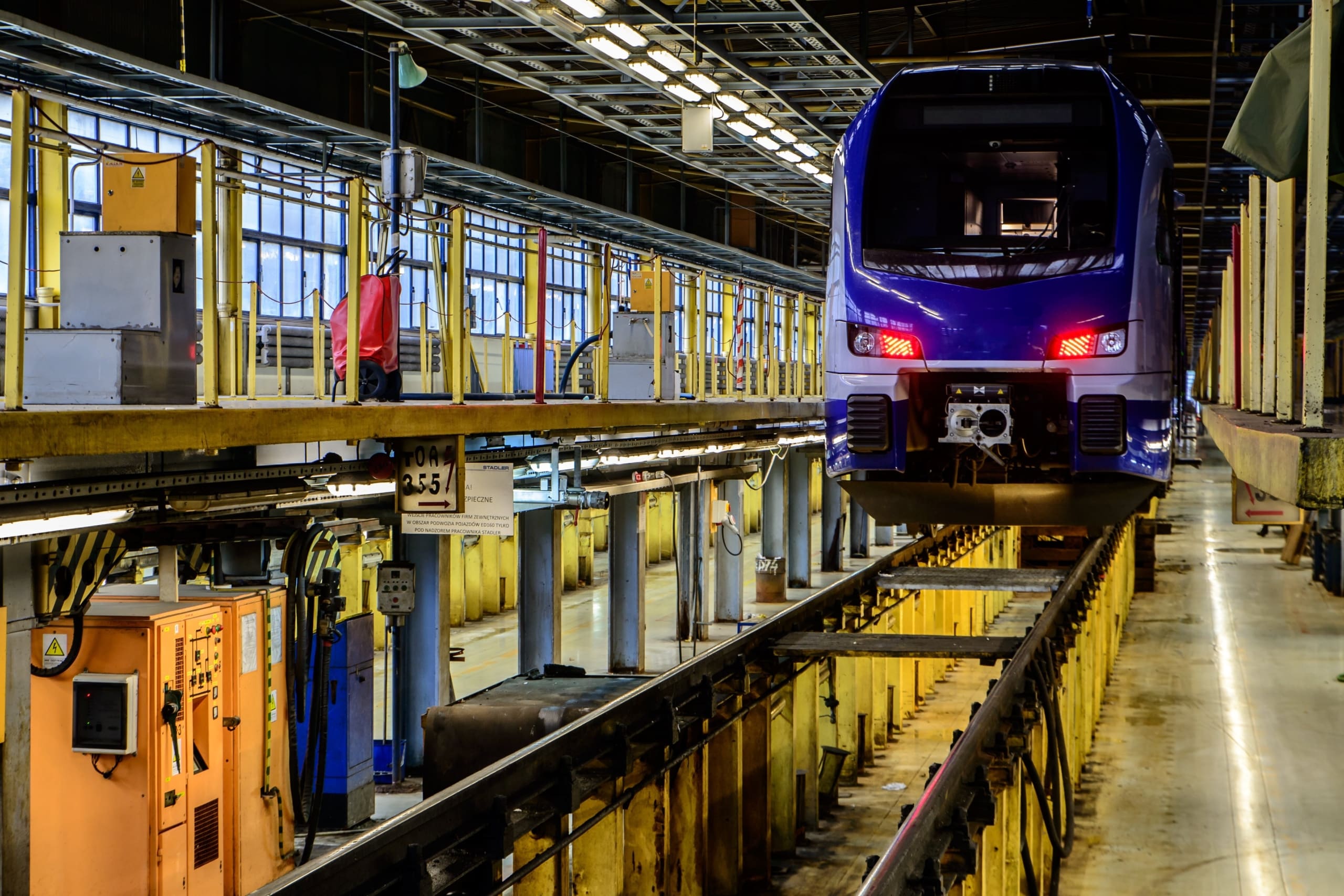
Episode summary: In the heavy industry sector, the cost of unpredicted repairs or machine failures can be very expensive. For example: A cargo train with an engine failure in will incur costs from its own repairs, from the transit required to reach the broken down engine, and with holding up other trains and cargo in the process.
Predictive maintenance has the potential to help businesses assess the condition of vehicles, equipment and parts in order to predict when maintenance should be performed. Using data collected by sensors on machines (including vibration, temperature, and more) heavy industry companies can potentially predict which machines or parts need imminent maintenance and which machines are least likely to break down.
In this week’s episode, we speak with Will McGinnis, Chief Scientist at Predikto, a predictive maintenance software provider based in Atlanta. Will speaks with us about predictive maintenance applied for the improvement railways and trains equipment, and how companies in the railway sector can use predictive maintenance to coax out patterns in maintenance schedules and heavy equipment data.
Subscribe to our AI in Business Podcast with your favorite podcast service:
Guest: Will McGinnis, Chief Scientist at Predikto
Expertise: Machine learning, mechanical engineering
Brief recognition: Will earned an M.S. in Mechanical Engineering from Auburn University before going on to going on to become a Graduate Research Assistant there. After this he served as the Senior Architect for Predikto before going on to become the Chief Scientist.
Big Idea
Predictive maintenance has applications in manufacturing environments like factories which are small local networks with access to high data transfer capabilities. In mobile heavy machinery (such as locomotive, cargo ships, or 18-wheeler trucks), data is often sent over cellular networks at lower bandwidths which in turn means that the data collected cannot be as ‘dense’ as that collected within an individual factory.
According to Will, predictive maintenance is capable of augmenting existing an maintenance crews’ abilities for applications in mobile heavy distributed assets like trains airplanes or shipping. For example, railway operators in the US are often required to bring their assets in for federally mandated maintenance; Will believes that predictive maintenance can assist maintenance crews in diagnosing any additional maintenance issues apart from those required by regulation to make sure the asset doesn’t come back for unplanned maintenance (which is much more costly and bothersome than planned maintenance).
Considering a typical locomotive use-case, Will explains that predictive maintenance for uses many kinds of data including:
- Sensor data – Vibration sensors or temperature sensors on locomotive parts
- Operational data – The schedules and routes for each locomotive
- Maintenance data – The records of past maintenance on each locomotive in the fleet
- Weather data – The weather along the planned route for each asset.
A few examples of end-results that predictive maintenance can produce for businesses in the mobile heavy machinery industry might include:
- Identifying the top priority assets that are least likely to have breakdowns on a particular day.
- Finding maintenance patterns that are likely causing repeat equipment failures.
- Ensuring that maintenance issues which may take a few hours or more, are handled at a location with the proper equipment and staff to make that repair quickly.
Will states that there are still many challenges for predictive maintenance in the mobile heavy machinery today, including standardization issues (data from different parts of a specific train car model might not transfer to understanding other models of train car), human error in collecting operational data, and difficulties in streaming the data from mobile assets (which requires drawing sensor data from sometimes remote locations and in sometimes difficult weather conditions).
It is often the case that certain maintenance industry experts work with the same mobile heavy machinery for at time even a few decades and Will believes that gaining the nuggets of wisdom that only comes with such long term experience will be an important factor in configuring better AI platforms. He also says that there may be a harder push for automated predictive maintenance in the next five years due to the smaller number of younger talent entering maintenance industry in recent years. According to Will:
“There is a huge average age gap among personnel in the industry (around 20 years) between maintenance and physical engineering fields which is a cause for concern today for the industry.
For example if a company owns 74 ports all around the world and the maintenance personnel all speak different languages, transferring the institutional knowledge from these ‘subject matter experts’ to younger generations is challenging and technologies like natural language processing may be one of the ways to resolve these issues”
(Readers with a more broad interest in AI for heavy industry might enjoy our interview with Caterpillar’s Dr. Sam Kherat.)
Interview Highlights with Will McGinnis from Predikto
The main questions Will answered on this topic are listed below. Listeners can use the embedded podcast player (at the top of this post) to jump ahead to sections they might be interested in:
- (2.55) What is possible with predictive maintenance in the heavy industry as opposed to what was done in the past?
- (6.30) What are some common use-cases for predictive maintenance today and how does it work?
- (19.50) Where do you think this technology will become ubiquitous in the next five years?
Subscribe to our AI in Industry Podcast with your favorite podcast service:
Header image credit: Adobe Stock