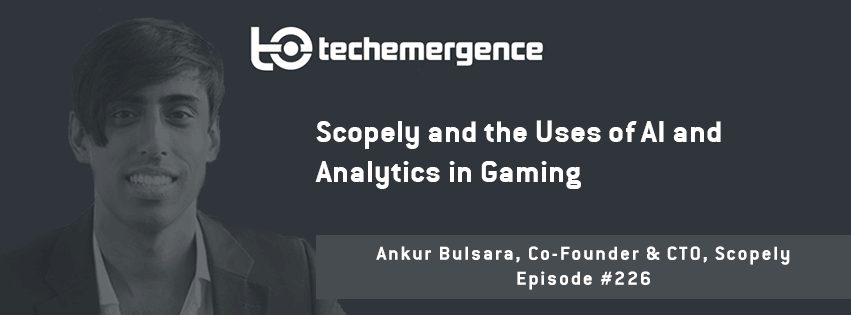
Episode Summary: One of the most clear insights from our recent consensus on machine learning in marketing was that companies who have more digital touch points along the path to conversion—and more conversion in general—have an advantage when applying AI and ML technologies. In this week’s episode, Scopely Co-Founder Ankur Bulsara shines a light on this dynamic and describes how gaming companies are taking advantage of digital trails and applying machine learning technologies. We don’t cover much gaming on the Emerj podcast, so this interview is a bit off the beaten path. Bulsara speaks about how dialed-in and instrumented the mobile gaming environment is and how data is used to leverage higher conversions over time, as well as how Scopely’s systems are set in place to ensure success of their business model. We think his insights on how gaming companies leverage higher conversions with (and without) machine learning can serve as an analogy for companies in other industries that are considering how to set in place similar, optimal digital processes over time.
Expertise: Software architecture and engineering
Brief Recognition: At Scopely, Ankur Bulsara oversees all aspects of technology and engineering. He has experience building large-scale and robust systems used by millions of people across multiple platforms. Prior to co-founding Scopely in 2011, Ankur was the Founder of Brainwave Software Inc., a consulting business that included clients like Myspace and uSamp, among others. While consulting, he held several different positions with his clients, including Architect of Revenue and Inventory Optimization at uSampa, Architect/Lead Developer at Internships LLC, and Architect at Freedom Speaks. In 2007, Bulsara became the Lead Software Developer on Myspace’s Developer Platform, where he was responsible for the developer website and the portal dashboard for developers to create and manage Myspace apps. He graduated with a Bachelor of Science in computer science from Cornell University.
Current Affiliations: Co-founder and CTO of Scopely; Member of Forbes Technology Council
Big Ideas:
1 – Use AI Only if It’s the Best Tool for Getting Business Results
Determining the business value in using machine learning to solve a problem is dependent on what goal you want to accomplish or achieve. Bulsara states that if you’re not aiming to implement solutions like machine vision or speech-to-text technology, it may be that the earlier work in problem solving can be tackled with a simpler model (he mentions regression analysis or heuristics). For example, Bulsara postulates that if you want a model for solving customer churn (churn defined as anyone who hasn’t played a game in 7 days)—a distribution problem—you can use a heuristic model and garner 80% of the results of a machine learning model to get immediate business value. Comparatively, building a more refined machine learning model might require three months time and investment of resources.
Turning Insight into Action:
Don’t use AI like a tool that’s looking for a problem. Solve your most important problems effectively, and only use AI when it is the best tool for business ROI. Ask yourself: Has the business problem that you’re working on historically been solved with good results through simpler regressions, heuristics, or other models? If you’re lacking the necessary machine learning infrastructure (a robust data platform, for example) and not working on areas where machine learning almost always has the advantage (say advanced analytics), then the cheaper and quicker tool may be the better option for making early progress. Bulsara emphasizes that it’s always worth questioning the ROI of building any machine learning model based on problem context and business resources available.
Interview Highlights:
The following is a condensed version of the full audio interview, which is available in the above links on Emerj’s SoundCloud and iTunes stations.
(3:40) You had talked about just how business intelligence heavy…how instrumented the nuance of the mobile gaming environment area is—why is that?
Ankur Bulsara: The first place to start is realizing how big the mobile gaming marketplace is, so I encourage listeners to go to the Apple app store or Google play store and click on top growing, and you’ll see that maybe 90% of the apps are all games. What this tells us is that most of the things that make money on the mobile smartphone platforms are games, and you pair that with low conversion rate of payers and free to play gaming market, and it becomes critical to optimize the 2% to 3%; this is where you really need deep understanding of your users and analytics to be able to hone in on that 2% that will actually pay in the game, and then convert some of that 2% and even smaller percentage into your whales.
(5:20) On the average…you had mentioned 2% or 3 %—is this what a well-instrumented, well-orchestrated, free to play mobile game might expect? Is this baseline metrics for your company or the industry at large?
AB: This is pretty industry standard, you’re going to get about roughly 2% to 3% payer base…the thing to realize about these games is 98% are playing completely for free and having a great time, and we still have to pay for those installs, those marketing costs, those infrastructure costs, and that’s typical for the industry, that you have a very low payer conversion and an even lower whale ratio…
(6:40) This is to say people who would regularly and consistently pay for…the best features, the best abilities, people who would upon regular use consistently take you up on “the cool thing”…what constitutes whaledom?
AB: We want to keep them highly engaged, we don’t judge them by recurring payments as much as we do cumulative lifetime spend, so the whales are those people who have spent the most in your game, and it depends on the game…in some cases you may be buying acceleration to make something happen…we also run a lot of live events, and the live events—these are things like tournaments or alliance wars…and these social competitive events help to drive a lot of monetization, and it’s very reusable…instead of selling physical goods, we’re selling virtual goods, and just like any eCommerce platform, it’s up to us to keep that merchandise fresh, to create sales and opportunities to spend, to create want and desire, to create vanity and social competition…
(11:38) Talk to us a little about what were some of the initial applications of ML in this space for you guys, where you saw the ROI and it became a legitimate use of whatever (language) you’re leveraging; where did you first implement ML where it really made a difference?
AB: Taking a quick step back, the first thing we had to do—and I think this is important for any company—is we had to build a data platform…we had made the mistake before of trying to apply ML to a poorly formulated data set, and there’s an old adage in data of “garbage in garbage out”, and really until you get rid of the all the garbage coming in your data platform, it’s hard to build any kind of modeling…we’ve taken very significant strides, and I’d say now we have a quite robust and highly competitive analytics platform, compared to what’s in the commercial marketplace…and once we were able to do hindsight analysis in an accurate way, we started considering what is the predictive stuff we can do…
…one of the first use cases—that was to predict, or really estimate, lifetime value of people that we wanted to bid for. This is important because you need to calibrate your bids, and rather than make up a bid, you want to have some confidence that you are not overpaying and you want to bid as high as possible in a growth phase without bidding above your LTV…it’s really critical at first to get these games at scale, because if we don’t, you’re going to have a hard time creating enough social critical mass to make the game interesting, to create enough social competition, to create enough of a user base.
(15:26) From what I gather, you’re looking at immediate activities that these people take…have they taken the micro-actions, are they the kind of engaged users that do these things that tend to become the kinds of people with this likelihood that end up paying us X…I take it that this is what models are getting trained on.
AB: That is a more accurate description, and the other point I would make is that the other reason why we can’t wait four or five months…is the game will have evolved so much in that five months that essentially it’s a different game…this goes back to live content and frequent updates, and we try to have one release a month with a big new feature…one of the things we look for, from a data perspective, is are the new cohorts monetizing at a higher level than the old cohorts? Our product needs to improve over time, and this is why you need a little bit more machine learning to be more highly adaptable to these kinds of early signals that may be indicators of future spend.
(18:54) I’m fascinated by this environment, this highly-instrumented jungle that you live in, which sounds like a hits-based world…when you went about implementing AI…what did you have to base your initiatives on, what informed your probably very expensive investment into the AI and ML space?
AB: I would say data science is one application of our data platform, and so the investment was into a data platform, not an investment in AI or ML specifically, it was an investment in an overall data platform…once you have a data platform, you can start to do some interesting things; you can build a search topography on top of that, you can build some predictive models on top of that, you can build some systems that affect production on top of that…and I would say that a lot of the problems, like churn for example, you can solve some of these problems with very cheap heuristics.
Part of it is, can we improve this over time, can I solve churn with a simple heuristic and can get 80% of the lift that a ML would do, and you actually can. And then when you get more sophisticated, you can try to tackle that next 20%…
…we’re not dealing with image recognition, or speech-to-text, so we were dealing with problems in the business world that have been tackled historically with simpler regressions or heuristics, and those can get you pretty far. I think it’s important to always question the ROI of building these models…