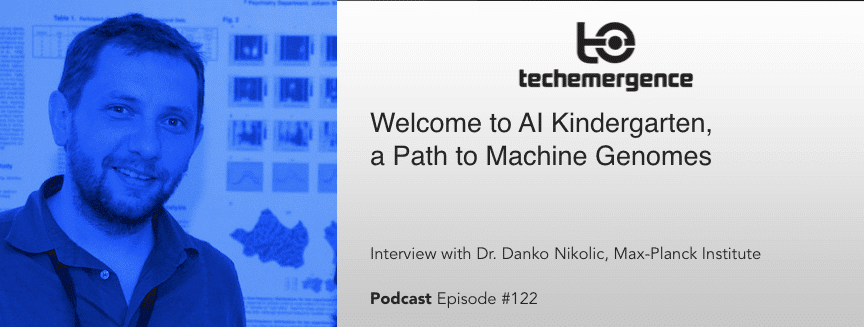
Episode Summary: Dr. Danko Nikolic, a scientist at the Max-Planck Institute for Brain Research, asks and works to answer questions about how our physical neuronal connections create the mind’s perceptions. In the realm of AI, Danko zones in on learning in a newborn human and compares that to a robot. He asks how we can take human lessons, what’s built into our genome, and apply that to construct a more generally intelligent AI, in a way that is not being done today.
Guest: Danko Nikolic
Expertise: Neuroscience and Artificial Intelligence
Recognition in Brief: Nikolic’s work in brain physiology has led to the creation of two theories, ideasthesia (sensing concepts) and practopoiesis (creation of actions). His ideas and work have been featured in TechCrunch, TED, the Guardian, New Scientist, Nature, and others. He has published numerous articles in the areas of neuro-, cognitive, and behavioral science.
Current Affiliations: Max-Planck Institute for Brain Research, Ernst Strüngmann Institute, Research Fellow at Frankfurt Institute for Advanced Studies, and Professor at University of Zagreb
Back to Basics
It’s often a good idea to start with the basics. When defining the differences in learning between a child and a robot, Dr. Danko Nikolic remarks that the biggest difference is that biological children come born with a large set of knowledge. Children seem to know what to learn, where to pay attention, and their gene-programmed instincts help them through their developmental stages. Robots, on the other hand, come as a tabula rasa.
Building one that is similarly intelligent to humans, that is “born” with the same type of low-level knowledge, requires more than programming – it requires a robot with a set of basic knowledge, in much the same way as an infant. In fact, Danko believes that – at least at present – programming this type of primitive knowledge into robots is not possible.
“It’s too complex for a human mind to understand…we don’t even know everything that’s playing a role…so how do we do that? I think we can by using AI Kindergarten, and then we can basically interact with robots in a similar way that we do with children in real Kindergarten, but make robots learn at a lower level…at a level we call machine genome.”
Nikolic envisions creating a robot that would have this type of reflexive knowledge pre-programmed, which you could then treat as you do a child. “We are basically at a point where evolutions was billions of years ago ,” says Danko, “to accelerate this process…I think what we can do with AI Kindergarten is…steal this knowledge, take this hard work that evolution has done through eons, and have it translated into our robots, into their genomes.”
Nikolic notes that this process should, in principle, be possible.” He compares this transfer to the way we pass down our civilization rules, laws, and culture to the next generations of human beings. We don’t, as a whole, build an entirely new culture from scratch, and what took 1,000 years to create can be done in the span of a couple of decades.
Scaffolded Learning
One of the primary obstacles is that we don’t really understand the processes behind this theory yet, of how knowledge at the level of genomes is created and stored. What types of experiments and research pathways should we be following in order to shape this reality?
For starters, Nikolic thinks we should do something quite different from the standard programming approach. Instead of giving exact rules, Danko broadly suggests that we give examples of what should be done, give machines our own intuition in what is the right thing to do – machines would have to figure out rules on their own, which is exactly what kids do.
In the beginning stages, this teaching process would still look much different than it does with children, and we would have to start much earlier with robots, at a stage when kids would already know behavior basics. Danko suggests teaching a robot behaviors that you would teach a bug or worm to do, primitive and reflexive and instinctual behaviors at the level of genes. From there, robots could gradually improve to more complex behavior, more complex artificial brains, and eventually develop what we call “strong” or “general” intelligence.
Could this type of approach be achieved in a decade? Predicting is risky, but it’s always an interesting mind exploration. Danko poses that a baby with prebuilt learning mechanisms takes about 20 years (there are exceptions) to achieve adult-level intelligence. “I don’t know how we could hope to get there quicker, I think it wouldn’t be possible based on that argument,” he suggests.
Yet if researchers were to pursue the approach of a AI Kindergarten and put in enough resources, Nikolic believes we could develop robots that are a “biological-like” robots. These robots would still not be close to human AI, but you might think of them as quite a smart animal, in terms of how they behave.
Animals, even simple ones, solve basic problems in the face of novel situations, and have a certain level of flexibility and adaptability – and this is much more than we have with robots today. “Take any of today’s robots, leave them in the jungle, come two years later, (and) what you will find is just a bunch of rust; take any mice, leave them in the jungle, come two years later and what you will find is probably just many more of the same mice,” explains Danko.
His mission is to build these more adaptive robots based on the nature of life’s biological systems, a different way to organize AI systems than programming endless bits of complex information.