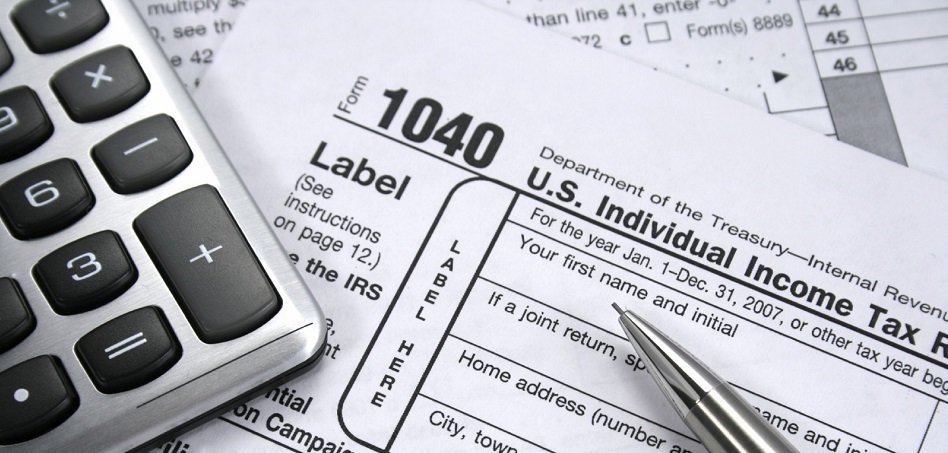
Episode Summary: Many machine learning applications in business can be boiled down to some form of decision support. There are big decisions like deciding whether or not to merge or acquire another company, and there might be smaller decisions like whether or not a tumor has enough traits that make it seem like it’s worth a surgical procedure or if it’s worth leaving alone.
In this particular interview, we talk about the domain of decision support, specifically in tax and accounting. There are few firms that know more about tax and accounting than Ernst & Young, and there are few people at Ernst & Young who know more about artificial intelligence than Sharda Cherwoo. Cherwoo is a partner at EY, and she is also the Intelligent Automation Leader for the Americas division of its tax practice.
Cherwoo talks about where decision support is being influenced by machine learning in accounting and tax today, the initial experimentation, traction, and results. She also paints a picture of bigger decisions that might be automatable by machine learning software. The focus of this episode may be on tax and accounting, but here are transferable lessons for business leaders in all industries that revolve around how machine learning can help inform decisions made by human experts.
Subscribe to our AI in Industry Podcast with your favorite podcast service:
Guest: Sharda Cherwoo, Partner, Americas Tax – Intelligent Automation Leader – Ernst & Young
Expertise: enterprise adoption of AI
Brief Recognition: Cherwoo has been with EY for over 36 years. She became a partner in 1991 and was CEO of the company’s Global Shared Service Center company in India between 2001 and 2004.
Interview Highlights
(03:00) Obviously, tax and accounting is your world. Where do you see machine learning fitting in?
Sharda Cherwoo: So today we’re seeing machine learning in several areas. I wouldn’t say it’s been industrialized to the “n”th degree, but we’re seeing spotty usages of machine learning. One that comes to mind is fixed assets. One of the big challenges that companies have is when they have a large fleet of assets, desks, chairs, laptops, and everything. There’s a lot of accounting and depreciation and disposition and gains or losses on all that stuff that needs to be recorded, right?
And every so often you have misclassifications. Something that might be classified as five-year depreciable assets might be put into ten-year depreciable assets. There’s a lot of stuff that goes on that isn’t quite what it needs to be. Today what we see machine learning applications … That’s one use case that we see that’s emerging.
I wouldn’t say most companies are sort of are on that path, but certainly we’ve seen companies, and we’re working with companies, who actually are using machine learning to kind of straighten out, making sure that if every month someone’s recording something as a desk and it really should be a chair, through the data that’s ingested by the machine learning tool, it sort of corrects. It auto-corrects and gets it into the right category just by looking at the data. Every time something looks like “this,” it’s an invoice here, it says “this,” it really should be a desk, it shouldn’t be a chair. Right? That kind of stuff.
So we’re seeing machine learning in that application. We’re seeing a lot in the application of expenses, correct categorization of expenses where whether something is travel versus a meal or a hotel bill that needs to be split into different pieces. It’s relevant because travel gets deducted differently than meals do, right? That type of stuff.
So there’s a lot of point solutions for specific problems that are fairly narrow at this point: correcting stuff that’s routine, that’s automatic, that might be caused because of human error, or just misclassifications. Invoices are a great example also. I’ve seen lots of invoices come into organizations to get paid, and there’s a lot of manual time today that’s spent on, “Well this invoice might sound like this, but it’s really for paper and not something else.” So auto-correcting, ingesting tons of invoicing. Every time it’s from company “A” and it says something like “this,” it’s really for paper and someone might have classified it as something else. So, a lot of invoice machine learning. [Another example is] tax rates being corrected. If you’re always in a certain jurisdiction, there are local taxes and whatever else, and someone just put the wrong tax rate in, right?
(06:45) [Is historical data for anomaly detection the biggest use case] or is there a little bit more on the front end in terms of programming and instrumenting that we need to do?
SC: I think there’s a little bit more. It’s not really the anomaly cases, it’s finding the errors, maybe some are bad, and also then finding patterns of something that might have happened. So it is finding the anomalies, but it is also auto-correcting and them making sure it gets into the right category. I think what the beauty about this stuff is that if the machine can’t figure it out, it gives you the confidence level: “You know I think I’m right, but I think I might be 80 percent right, there is a 20% chance of error.” So throwing out these sort of confidence level to apply human judgment is also what you end up sort of seeing through machine learning, it’s just ingesting huge amounts of data and doing it much quicker than human beings.
[Maybe] there’s an anomaly if the goods were shipped “X” place, and the sales tax rate should have been 7%, and the local flat rate should have been 2 percent, and somehow it sees an anomaly that it’s only 5 percent or knows local rates apply just from kicking those out as well. So, it sort of, again, like connecting the dots between those parameters saying, “This is what I think it should be, but it’s this.”
(11:30) When you guys look ahead at where accounting and tax might be, are there some exciting vistas of capability that maybe are not there now, but we’d really like to get to…does anything jump to mind that you are excited about?
SC: I think what is going to be really exciting is there is a lot of rules and thoughts and experience that’s built into our brains, especially over the universe. Maybe 80 percent of this rule base, but there is a 20 percent judgment element, right, or based upon experience. Where there is a gray decision being made to one versus the other.
I think what’s gonna be very interesting in the tax field or obviously on taxes where I typically spend most of my time in, is just the ability to take a bunch of information and being able to conclude, with a fair amount of confidence that when you read this kind of stuff, and if you see these 16 facts, and if you see these, that this is what’s relevant, this is what the answer probably should be, and human beings take a look at it, so a lot more in terms of cognitive decision making based on old cases, a lot of what gets done in tax planning, just looking at tax, really being able to take voluminous amounts of tax cases, et cetera, and being able to find them and conclude, and really put together the summary of what might be the answer. Is something that’ll I think help.
Just like it helps surgeons and the medical field. I think it’s the same concept, where you see a lot more advances of that in the future then we have today. I think what is going to be very interesting, is that I love the idea of, if you had 20 very smart brains, or even 100, or whatever, take 20 to start, at a practice in a certain field, right, it is virtually impossible, especially in the gray areas, to get the same answer if you talk to 20 different people.
I think what is going to be very exciting is to be able to have a world where you actually have consistency because my brain through all these examples concludes “X,” your brain concludes “Y,” and then you might have 10 other answers, but then being able to discover what those answers might be. Being able to then make sense of this and maybe get a better answer or the more popular answer today or over time. I think that is a pretty interesting way to apply this because today when you give a case defined for lawyers, they might come up with five different versions of what the answer might be, or accountants.
Today we don’t know how our brains think, so what this is going to help us do is to have that transparency across whatever domain. Are 10 people concluding differently on the same thing?
(16:00) So layering that expertise into a network to flag that for human experts by baking it in the front end. Am I following you correctly?
SC: Yes, in addition to that what you also start seeing is experts, maybe at times don’t even agree, you almost start seeing why they don’t agree with something. I may look at a document and conclude this fact is more important than that facct as I am training the machine to learn what I know, and someone else might do something a little different because they find something else more important, but today I think a lot of that is done involuntarily.
It just happens and you really don’t understand the logic as much, as clearly. What this can also allow you to do is see the dimensions of the conclusions and why people think differently. Then think about what might be a better answer. It is almost like a best-agreed answer because I might hone into one fact that’s more important to me when I am reading something, so I tag that in the machine. You might hone into something else that you find is one that I didn’t find. So now what you really come up with is a sort of a best-to-read. It is almost like getting nine smart brains together and combining them and getting a perfect brain. So I think that the ability to take each experts’ brains and create the best brain available is a pretty interesting thought.
Header Image Credit: Freedomworks