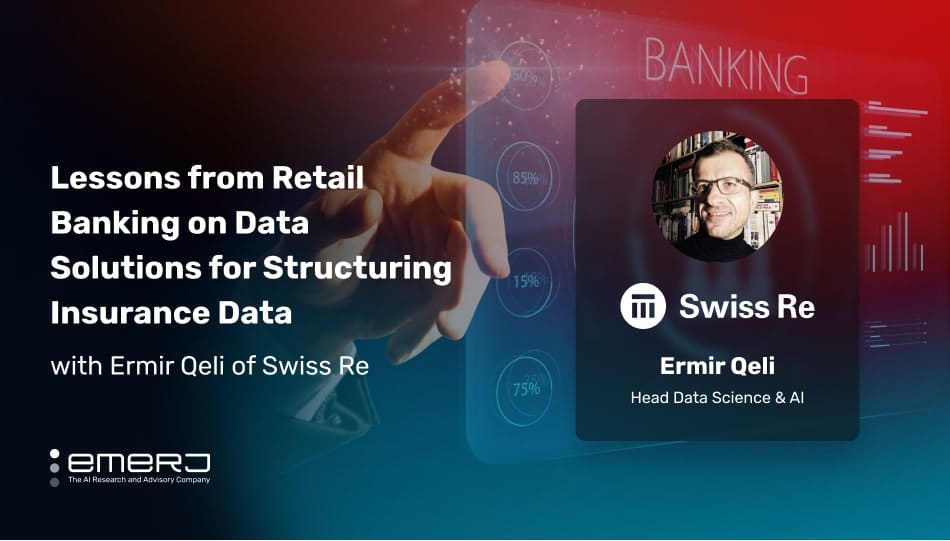
Like much of the financial services space broadly, the insurance industry is growing interested in adopting AI. The technology holds immense potential to revolutionize insurance, enhancing efficiency and accuracy across various processes such as claims and underwriting. However, concerns surrounding data privacy and integration complexities have prompted a measured approach among industry leaders.
Delaying AI adoption could perpetuate inefficiencies, escalating costs, and hinder competitiveness in an increasingly digital landscape. Arguably, the biggest challenge in insurance right now is the wealth of data available, yet leading studies and thought leadership have found time and time again that poor quality and inaccuracies can lead to a significant lack of trust.
A study by MIT Sloan found that “estimate[s] the cost of bad data to be 15% to 25% of revenue for most companies.” This trend holds especially true for the insurance industry, as underwriters need reliable data for risk assessments, and carriers must meet strict compliance requirements and regulations.
How can insurance leaders balance the challenges of adoption while effectively extracting the value of AI technologies? By leveraging proven strategies from other sectors, such as retail banking, and adopting AI incrementally, they can avoid potential pitfalls and maximize the benefits.
To gain a greater perspective on the most valuable approaches for AI adoption in insurance, Emerj Senior Editor Matthew DeMello recently chatted with Ermir Qeli, Head of Data Science & AI at Swiss Re. They discussed how AI is transforming the insurance industry and what insurance leaders can learn from the banking sector’s experience with data solutions.
The following analysis will focus on two critical insights from their conversation:
- Utilizing generative AI (GenAI) to systematically organize unstructured data: Focusing GenAI initiatives to solve inefficiencies in data collection in the insurance and reinsurance industries, leading to more streamlined datasets.
- Streamlining data flows to assess risk and prevent fraud accurately: Combining machine learning and new generative capabilities to analyze these improved data sets to spend less time processing submissions and more time processing claims, ultimately delivering more value to the consumer.
Listen to the full episode below:
Guest: Ermir Qeli, Head of Data Science & AI at Swiss Re
Expertise: GenAI, Large Language Models, Data Engineering, Digital Transformation, Data Science, Machine Learning
Brief Recognition: Qeli, named Head of Data Science & AI at Swiss Re in June, has worked in various roles over his 10 years at the company to solve business problems related to data science and bring analytics to the heart of the business. Prior to Swiss Re, he held several positions focused on data analytics, visualization, and software engineering, with extensive experience in the retail banking space. He received a degree in Computer Science in Tirana, Albania, and a PhD in Computer Science at the University of Marburg, Germany.
Utilizing GenAI to Organize Unstructured Data Systematically
The interview opens with a discussion of the differences between the banking and insurance industries regarding data utilization. Ermir Qeli highlights that, historically, insurance relied heavily on unstructured data, introducing inefficiencies in their systems. Unlike banking, which has seen significant advances in data structuring and analysis, the insurance sector often needs help with complex IT landscapes that make data analysis challenging.
Qeli explains that unstructured data complicates data analysis and is challenging to integrate across various systems. These obstacles lead to a fragmented approach to data management. To address this, Qeli suggests that AI technologies, particularly GenAI, which excels in analyzing unstructured data, can be of particular use to the insurance industry.
He notes that GenAI has significantly lowered the barrier to tapping into advanced technologies and performing data analysis. Large language models (LLMs), the core of GenAI, are particularly effective in processing unstructured data, making them an excellent starting point for insurance companies beginning their AI journey.
GenAI automates data analytics by using natural language processing to extract relevant information from text-heavy documents, categorize data into meaningful segments, and identify patterns that would be difficult or time-consuming for humans to detect manually. These automations allow insurers to process vast amounts of data more efficiently, reduce manual errors, and quickly gain insights that inform better decision-making.
Examples of applications:
- Natural Language Processing (NLP) for policy analysis and interpretation.
- Sentiment analysis for customer feedback and claims handling.
- Predictive analytics for customer segmentation and personalized pricing.
- Image recognition for assessing property damage claims.
However, Qeli emphasizes that robust data foundations are crucial for leveraging AI at scale and creating a competitive moat (a unique competitive advantage that a company accrues through its AI technologies) for the company. Robust data foundations involve ensuring data quality, consistency, and accessibility across the organization.
To accomplish effective data hygiene in the insurance enterprise, Qeli emphasizes that insurance leaders will need to invest in data governance frameworks, data cleaning processes, and data integration tools that standardize and harmonize data from diverse sources.
These foundations are necessary for even the most advanced AI systems to be able to deliver accurate and actionable insights. Qeli stresses that building these foundations is not just a technical challenge but also an organizational one, requiring a cultural shift towards data-driven decision-making and continuous improvement in data management practices.
Streamlining Data Flows to Assess Risk and Prevent Fraud Accurately
Pulling extensively from his background in retail banking, Qeli discusses the step process for AI adoption, particularly for traditional insurance organizations burdened with legacy systems and unstructured data. He recommends a pragmatic approach, starting with small, manageable projects that demonstrate value to business leaders. This approach helps secure buy-in and resources for more significant initiatives.
One compelling example Qeli provides is the use of AI to triage and process large volumes of documents, such as insurance submissions and claims. By using LLMs to summarize and extract critical features from documents, companies can optimize workflows and allocate resources more effectively. Leveraging LLMs for document processing not only improves operational efficiency but also enhances customer service by speeding up claims processing and providing more accurate assessments.
In addition to streamlining data processing, AI is also being used to prevent insurance fraud. Qeli explains how machine learning algorithms can detect patterns and anomalies in claims data that might indicate fraudulent activity.
For example, AI can analyze historical claims data to identify unusual patterns, such as multiple claims filed from the exact location within a short time frame or claims that deviate significantly from established norms. By flagging these suspicious claims for further investigation, AI helps insurers reduce fraud-related losses and ensure that legitimate claims are processed quickly.
Qeli also highlights innovative applications of AI in the insurance industry, such as parametric insurance. Unlike traditional indemnity-based insurance, which requires manual assessment of damages, parametric insurance relies on predefined triggers (e.g., weather indices) to automatically pay out claims. The approach is data-driven end-to-end, requiring high-quality data for both pricing and settlement.
Examples of additional applications:
- Predictive modeling for risk assessment and pricing accuracy.
- Robotic Process Automation (RPA) for automating routine tasks in claims processing.
- Telematics data analysis for usage-based insurance models.