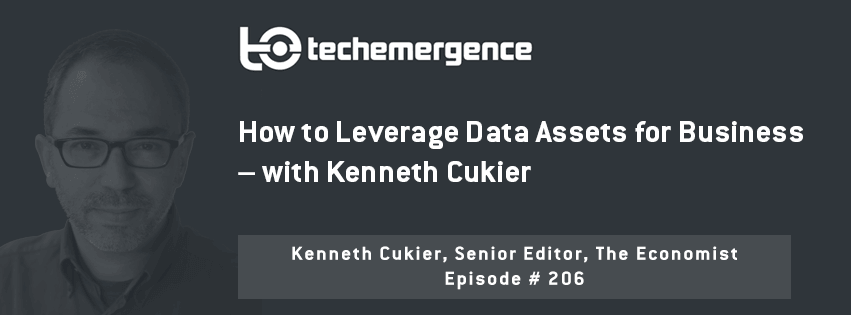
Episode Summary: In this episode, we speak with Senior Editor for The Economist in digital and data products and Co-author of “Big Data: A Revolution that Will Transform How We Work, Live and Think”, Kenneth Cukier, who speaks on the technologies that underlie big data and make it what it is today. Cukier addresses common misconceptions about machine learning and dives into how companies can catch up with this technology by thinking through, assessing ROI, and making sense of the dynamics of data assets for business. Listen for Cukier’s apt analogy in comparing machine learning technology to the dynamics of computing from decades ago.
Expertise: Data analytics and digital product development
Brief Recognition: Kenneth Cukier is the Data Editor of The Economist in London and the co-author of the award-winning book “Big Data: A Revolution That Will Transform How We Live, Work, and Think” with Viktor Mayer-Schönberger in 2013, a New York Times Bestseller translated into 20 languages. He is a regular commentator on BBC, CNN, and NPR, and a member of the World Economic Forum’s council on data-driven development. In 2002-04, Mr. Cukier was a research fellow at Harvard’s Kennedy School of Government.
Current Affiliations: Senior Editor of Digital Products for The Economist; Board Director of International Bridges to Justice and Member of the Council on Foreign Relations
Interview Highlights:
The following is a condensed version of the full audio interview, which is available in the above links on Emerj’s SoundCloud and iTunes stations.
1:34 – What do you consider to be the technologies that are really supporting big data and allowing for meaningful applications in this space?
Kenneth Cukier: “It’s been used in lots of different ways and misused as well…sadly, the term big data has been sort of diluted, to basically mean everything from an excel spreadsheet to Hadoop, but there is a usefulness to it, and it’s going to describe something that is still very useful for everyone…
…we are applying traditional statistics to problems that never had a quantitative bent before, we’re taking the scientific method in a robust, muscular sense of how to take data, collect it, analyze it, and report it back using rather sophisticated statistical techniques, but we’ve democratized it because it’s now based on software on our computers, and we can now do it for marketing campaigns, and for human resources, and a host of other areas where before it was only applied to physics; and so it’s still a win for society and for everyone…but at its core, big data is just a rebranding of the term machine learning”
10:32 – What do you see as some of the biggest misconceptions about these chronologies around big data that you see in the business world?
KC: “What I’ve observed is that interestingly the biggest obstacle is that there is not a conception about it, for must business people, they really don’t understand, they don’t even think about it…so the first thing we need to do is educate them…now most people are catching on to the idea that there is something about data…the first misconception is that it’s not ready yet, and the second misconception is that it’s ready to go, and the truth is it’s somewhere in the middle…”
16:36 – Machine learning still seems very wizard-like in terms of some of its applications…would you liken that analogy, that maybe that’s part of why it’s not ready yet, or do you think there’s other reasons?
KC: “There’s multiple reasons… in the case of classical machine learning, I would argue it’s simply statistical inference at a Moore’s law scale, but you need a lot of data to get the inferences very good because you’re dealing with subtle probabilities, so an example of that would be language translation…
…deep learning, which is considerably different than classical machine learning…there’s a lot more black magic to get going, and there’s really not that many people in the world who know how to do it – there, it looks more like nuclear physics in the 1930s and 1940s…people are making a good valiant effort at it but not really getting it to work…for most companies, you don’t stand a chance to apply deep learning, you’re going to have to wait 10 or 15 years, I would guess, before it becomes a bit more democratized…”
22:23 – How are smart companies making decisions among vendors and navigating this landscape?
KC: “It’s still pretty early, companies are experimenting, they’re doing internal things, but there’s not many vendors…there’s certainly several that do data processing…but that will come in time as the tools become more sophisticated, and more importantly as companies get the data in better order – it’s cleaner, it’s labeled, they can do more things with it; they have to upgrade their infrastructure to allow this to take place…”
Big Ideas:
1 – Big data, though now a buzz word, is something revolutionary and still new in business and AI. Within a decade or so, overarching attention will be focused less on how the technology works – that will become a bedrock, much like ours computers – and more on product and how service is delivered to increase value.
2 – Misconceptions about big data persist because there is still lack of trust by some business people and regulators. This mistrust is in large part due to lost causality; people want the ability to understand why an algorithm got to a decision, and until a computer is able to explain its decision, these misunderstandings are likely to persist.