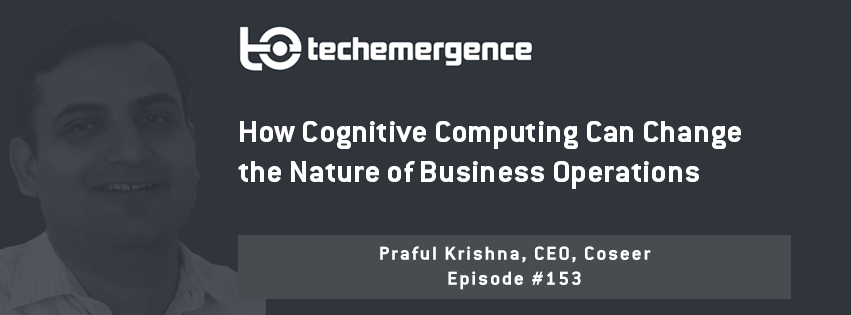
Episode Summary: When you go to Harvard Business School and then to McKinsey company to work in private equity, there’s really only one thing left to do – go to Silicon Valley and launch an AI startup. At least, this is exactly what CEO Praful Krishna did when he moved to San Francisco to start Coseer, an AI company focused on understanding natural language and unstructured data. In this week’s episode, we speak about where unstructured data lives in a business, and how a business can be changed if the right data is unlocked. Krishna also discusses his experience in how executives are making decisions around how, or how not to, leverage AI in their companies.
Expertise: Machine learning and data science
Recognition in Brief: Praful Krishna is a Harvard Business School (HBS), Indian Institute of Technology (IIT), and McKinsey alum. Prior to founding Coseer, he invested in technology companies across asset classes with ValueAct Capital, a hedge fund in San Francisco. He also ran a distressed company to achieve a successful exit. He is an avid coder and builds robots in free time.
Current Affiliations: Founder and CEO at Coseer
The Dawn of Understanding Big Data
At the most fundamental levels of reality, unstructured data is everywhere. Human beings interact with unstructured data on a daily basis; our senses, for example – we speak in language, see objects around us, hear sounds, have a sense of beauty, develop likes and dislikes. All of this information, which we often take for granted, is unstructured; there are no 1s and 0s underlying our most immediate realities. By its very nature, unstructured information is subject to subjective context.
Take our choice of words, for example; Krishna illustrates this point by sharing one of his favorite local examples: “In San Francisco when we say a good day, we mean 72 degrees fahrenheit…but if you took yourself to some station in Antarctica, 72 degrees basically means end of world,” he jests. In the world of business, most decisions fall into this same unstructured category. It’s not just about getting from point a to b, explains Praful, but how to use a specific strategy or approach to arrive at a more abstract solution. Though data science has improved how we capture vast amounts of data, we’re at a precipice in knowing how to process all of that collected, unstructured data.
“The problem is we’re trying to take unstructured and nebulous and subjective information and trying to impose quantified binary decision science on it…this is inherently the problem AI is trying to solve,” says Krishna.
Is it possible to quantify such information and if so, how far are we from being able to do it? Praful answers this in part by pointing out that data science lies on a continuum; on one end is decision science (the 1s and 0s bit) and on the other is pure thought process based on instincts. Human beings have the unique capability of rationalizing many decisions, but the first impulse is always instinct. Praful believes we’re taking the first few steps in the continuum in making machines ‘sentient’, or rational entities, and this involves trying to quantify more information along the continuum and scale up decision science; however, there’s a second step that can’t be ignored, and that’s adding context.
“Unstructured data is really more powerful if you have the right context…a simple word which is almost unspeakable today would be part of normal vocabulary a hundred years ago – today, you might call that word a racist word…doing sentiment analysis without context, it may have gone out of phrase, so that’s the next step,” says Krishna. He posits that today’s brightest minds have given up on trying to formulate causality and have accepted this point as an important reality. In contrast, the discussion by data scientists in the 1980s and 1990s around AI was focused on making a model or formula that could predict everything, though this conception of AI grossly failed.
“Now with the ability to capture nuances in big data…we can now say that ‘whenever X 90% corresponds to Y – we don’t know, but let’s take it as a given’,” says Krishna. In essence, though we still don’t understand the fundamental workings or underlying processes behind much of these correlations, we’re able to recognize the profound nature of a machine that can make sensible correlations out of previously untouchable masses of data. One day, we may reach a point where we do mostly understand how these processes work at a fundamental level. “The truth is our brains are 1s and 0s, electronic bolts in massive systems, and we can take multiple steps towards understanding (how that works).”
What’s in Cognitive Computing for Businesses?
Making sense of patterns clearly has an abstract sense of value, but what is the tangible benefit or yield of using cognitive technologies to help make better business decisions? Most of the time, says Krishna, people get into a debate over whether AI is just a productivity tool, whether the direction that data science is moving is really just taking what humans do and handing it to machines. But the kind of projects that Coseer is working on, for example, have the potential to fundamentally “change the way business is done” at client organizations.
For example, Coseer worked with a healthcare organization that deals with 10 million SKUs for a range of medical equipment and devices. Each SKU in turn had several attached and in-depth informational elements, such as brochures, white papers, and surgical protocols. “None of that (information) is structured, it’s not even all in English, but technology like ours allows us to help them say things like ‘Hey, SKU 256 is the same as SKU 375 but it’s 20% cheaper’; having that type of information transforms how you do business in that niche.”
Another example involves going into and opening an account with a bank. Sounds simple enough, but if you’re a small business owner who has ever tried setting up a 401K for yourself and your employees, says Krishna, then you know what a nightmare this process can be due to the amount of data required to be ingested. “It’s not because banks don’t like you or they’re being too selfish, it’s because there are so many regulations and forms to fill out and data points to be collected that even the bank representative doesn’t know everything,” explains Krishna.
What Coseer or other similar data analysis technology can now offer is a machine-learning based system that has the capability to read through hundreds or thousands of technical forms, become ‘smart’ by getting trained on past interactions involving those forms, and then leverage superhuman powers of analysis to make sense of and translate that information in a way that is efficiently digestible by humans. Coseer and others are developing streamlined, user-friendly interfaces, where such information is collected and can then (for example) be put into legal forms that have been converted into simple yes or no questions.
To wrap up this idea, the ultimate goal of pattern recognition and data analysis is to take a complex situation and simplify it, delivering a seamless process for the provider and the user — whether that be a bank helping a client navigate a complex account landscape or a corporation determining whether a potential acquisition is a lucrative opportunity.
Of course, knowing how to navigate and choose a provider for these nascent services – not to mention how to succinctly use this technology for achieving direct results – is a challenge for any business, great or small.
“Hundreds of billions of dollars will exchange hands trying to answer those questions…the fundamental truth is that unlike any other branch of discipline of computer science, cognitive computing is inherently probabilistic, which means that when someone like me comes and solves problem and says the answer is 42, what I really mean is it’s somewhere between 41 and 45,” says Praful.
Many of today’s successful CEOs and COOs have built careers on a foundation of deterministic decision-making, and using data analysis and related machine learning technology requires a big shift in mindset. “Really what we are delivering is some kind of gooey stuff, which may bake into a good cake, but it cannot get there on day one,” says Krishna.
It may take a generation to become comfortable with the inherent error that comes with this type of technology, or it may be that newer positions, such as virtual CIOs, will have the patience to make it through the learning curve until businesses start to see the advantages of AI in the context of their specific processes.
Image credit: LinkedIn