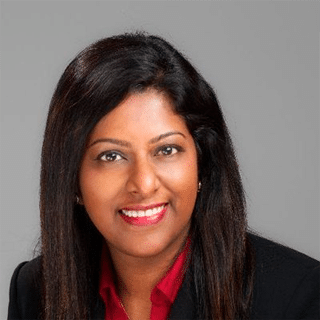
Episode summary: In this episode we speak with Sangeeta Chakraborty Chief Customer Officer at Ayasdi and discuss the applications of data science and AI in healthcare, what hospitals and healthcare systems are doing to adopt this kind of technology, and how this approach can be extended to other forms of enterprise. This interview was conducted in-person at BoostrapLabs annual AI conference in SF.
It’s easy to see how Facebook and Google or the best-funded Bay Area start-up are leveraging artificial intelligence, but what about industries that are often resistant to cutting edge technology? How are they adjusting the ways they are doing business to stay competitive with data science and AI, particularly in areas where sooner or later they will really have to adapt in order to stay in business? That’s what we set out to ask in this week’s exclusive interview.
(Readers with a particular interest in currently in-use applications in healthcare might like to read our recent article comparing the AI applications and initiatives at the 5 most prestigious hospitals in the USA).
Guest: Sangeeta Chakraborty, Chief Customer Officer at Ayasdi
Expertise: Entrepreneurial management, integrating AI systems.
Brief Recognition: Sangeeta Chakraborty has a Bachelor of Science, majoring in Computer Science, from the National Institute of Technology, India and an MBA, from the Walter A Haas School of Business, UC Berkeley. She is currently employed as the Chief Customer Officer at Ayasdi.
Current affiliations: Board Member, California Alumni Association, University of California at Berkeley, CA, Board Member, South Asian MBA Association, Chair, Women’s Leadership Network, South Asian MBA Association. Member of Haas Diversity Council.
Big idea:
“In large, established businesses, AI adoption often must start from the top”
What does it take to get older and more reluctant enterprises to want to make the move to adopt AI and use it to enhance their enterprise? In spite of many companies’ resistance to the introduction of AI, the most powerful factor in encouraging and readying a business or enterprise to make this move ahead of the curve is strong leadership from the top. This means having ability to think five to ten years ahead and to assess both what is going to be competitive and how they can improve quality. This is also combined with a willingness to adapt to new things.
Turning Insight into Action: Here are some actionable insights for older companies and industries that may be sluggish when it comes to adopting AI technologies.
- Look forward: Think about how implementing this type of technology can help you become more competitive and provide higher quality service 5-10 years down the road.
- Don’t be afraid of change: Be willing to adopt new technologies and strategies for the sake of growing revenues and cutting costs.
- Don’t wait until your data is perfect: Use the data you have available, this will grow as the technology is further implemented.
- Create a center of excellence: This will give you a centralized platform from which you can leverage data, drive your business and increase ROI potential.
Interview Highlights with AYASDI’s Sangeeta Chakraborty
The following is a condensed version of the full audio interview, which is available in the above links on Emerj’s SoundCloud and iTunes stations.
(2.28) We’ve done our best to cover some of the most prominent applications of machine learning in healthcare, but I know you’ve been seeing this work up close. From your perspective, what are some of the core issues in healthcare where machine learning can make a difference?
Sangeeta Chakraborty: There is a convergence happening and the divide that used to be there with the payers on one side and the providers on one side, that has converted into a focus on quality from both sides. They are getting incentivized to obtain higher quality results.
That means lower readmissions, faster recoveries and preventing people from getting sick. As a platform Ayasdi allows hospitals to build intelligent applications. One of the applications is provided by Ayasdi…is around the area of care variation management. The problem we’re addressing is that when a patient walks into a hospital for any designated surgery, they could be treated in any number of ways depending on the learning that the physician has had, the current journal they have read…it may not be the best outcome for the patient.
So now, with the advent of so much data and the prevalence of electronic medical systems…we have the data and the machine learning capabilities to actually understand what the ideal treatment is. What we do is expose that. It is the physician’s decision at the end of the day, but we expose the finding and say this group of patients had this ideal outcome…we can predict on whichever outcome you want to look at. Then we give you the characteristics that define that group…That gets manifested in the form of order sets and the physician can choose to use it or not.
(5.23) So much information has been and is being collected…if that information is here, we should use that. Have enough patterns emerged where we can come up with a viable action and should physicians use that as part of their decision making process?
Sangeeta Chakraborty: To an extent. It’s not just about different types of surgery but that literally the same thing could be treated differently depending on the knowledge that the physician has and what they are prone to doing. Our premise is why don’t we have the data exposed that seems to be giving the best outcome for patients? Then look at the variations and say maybe this is the best option.
(7.50) What are the challenges of getting AI adopted, and what are the commonalities of the crowd who are ready to adopt it? What makes them ready to step ahead?
Sangeeta Chakraborty: The industry is going to have them go in that direction because they’re focusing on the quality…so it is inevitable. Some of them are ahead of the curse where they have EMRs in place where they can access data in terms of all the clinical and operational things that have happened to a patient.
They have a system where they can set it up and they’re willing to look at this and talk to their physicians and say by no means is that replacing anybody. We are only here to augment the physician. This is all data for them to look at, make sure they have confidence because we ensure there is transparency in everything we provide. Then they can say “I’m interested to use this because this is new learning.”
Back in the day, learning was magazines and conferences, but now here’s new learning. It’s one more avenue for them to consume. It seems to be the hospitals that are more ready to embrace this new shift in the dynamic are the ones that are ahead of the curve.
(10.13) They’re going to have to have the budget in place…and the data available, are they also going to have to have someone at the top, maybe a CEO, to say that quality is what’s ultimately to drive our financial results and let us serve patients? Does it take gusto from the top to get it to trickle down?
Sangeeta Chakraborty: Yes, it definitely takes leadership thinking in the right direction five to ten years down the line. What do we need to do to compete and grow in our business? Every one of these organizations that we speak with has very strong executive leadership thinking along these strategic lines.
(For readers with a deeper interest in AI implementation, see our robust article called “How to Apply AI to Business Problems“, which features quotes and insights from many of our AI executive and researcher guests.)
(11.49) Are there any other commonalities about the way these adopters might be organized (within the medical field)?
Sangeeta Chakraborty: It doesn’t seem to be different from one type of specialty to another, but what I do see is that there is difference in the culture of being ready to adapt to new things.
(12.44) Are these people that are already a little bit ahead…not just in AI but in other sectors?
Sangeeta Chakraborty: They are the ones that realize they need an EMR, for example, they have gone ahead and got that done. That is an investment and now this is the next step of leveraging that. Typically those are the ones, they have data in place, they have infrastructure that is able to handle this.
(13.53): In terms of data science and people who are just getting used to bringing this in to healthcare or whatever other space that is not quite used to that, how do you guide other executives in that transition?
Sangeeta Chakraborty: Think about his not as data and machine learning as such, but this is a platform that allows you to build intelligent applications that allow you to discover things from the data, make prediction, justify the predictions and then act and learn. What you would need to do is first of all, don’t be afraid to start, if you haven’t started, you’re not making progress anyway…bring your data out into the business because it is all about solving a business problem.
You may not think the data is perfect but you may be surprised at some findings in the data you may have. Be prepared for organizational change, because you are going to act and that is going to lead to downstream changes that should give you more revenue or less cost. Finally, start a center of excellence around this because you’re going to learn and you need a centralized approach for leveraging and growing that learning.
(16.27) What does the implementation of that center of excellence look like?
Sangeeta Chakraborty: Think of it as a factory, and the factory is moving along the conveyor belt different use cases from conception where the business days “I have a problem,” and odes a series of tests to see if they can actually fix it by machine learning or whatever platform you’re working on, and then moving it to an implementation stage. Then it gets into deployment and it provides you with value. That’s the life cycle…The center of excellence centers around supporting that conveyor belt.
(18.14) Do you see the future of this technology as becoming more accessible to analyst level folks?
Sangeeta Chakraborty: Accessibility is the core of our strategy…We are building and application framework and it is purpose-built for the end user. It’s all about accessibility. (Users) should not need to have data science knowledge.. but know how to use the information because that’s (their) job.