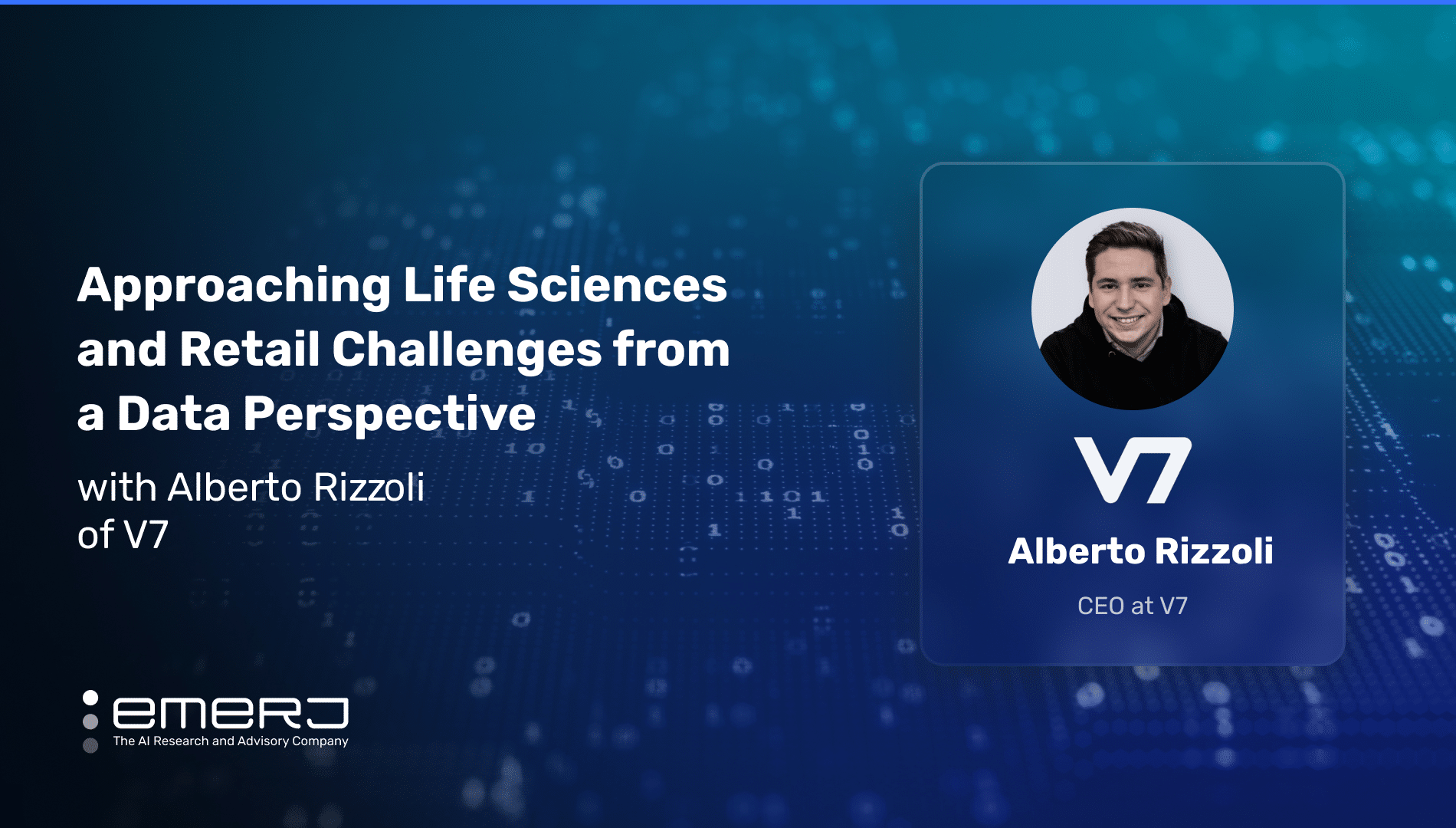
Problems in different industries can look quite similar when looked at from the perspective of data. Though diverse business problems originate from distinct domains and needs, they often share a common thread and solution.
Take, for instance, computer vision. Research published since the end of the pandemic consistently found that in both retail environments, computer vision can measure and observe customer behavior in the shopping aisles or notify empty shelves. Meanwhile, the same technology can be deployed on the factory floor to improve predictive maintenance processes.
As becomes evident when these problems are viewed from a data perspective, the same data solutions – in these cases, computer vision — can operate in service to different business goals and environments, bringing out varying results depending on needs, function and training.
Emerj Senior Editor Matthew DeMello recently spoke with Alberto Rizzoli, Co-Founder & CEO, V7 Labs, on the ‘AI in Business podcast to to look at how new generative AI and NLP-based tools can be deployed in contexts across life sciences and retail to handle different problems that look much more similar from a data-based perspective.
The following analysis of their conversation examines two key insights relevant to leaders across sectors:
- Making AI self-reliant for data labeling: Deploying self-learning AI algorithms in independent product labeling, reducing the need for extensive manual labeling.
- Tailoring AI solutions to the unique needs of the retail: Customizing off-the-shelf AI products to tune to the specific data and appearances of the retailer’s products.
Listen to the full episode below:
Guest: Alberto Rizzoli, Co-Founder & CEO, V7 Labs
Expertise: AI, deep learning and computer vision
Brief Recognition: Alberto is an Italian-born entrepreneur and the CEO and Co-founder of V7. He has a BSc in Management from Cass Business School, and in 2015, he joined Singularity University’s Graduate Studies Program at the NASA Ames Research Park under a Google-funded scholarship. In March 2016, Alberto was awarded by the President of Italy, Sergio Mattarella, for advancing artificial intelligence for a good cause.
Making AI Self-Reliant for Data Labeling
Alberto begins by establishing that the life sciences sector relies heavily on imagery for drug discovery and scientific research tasks. However, the images in these fields are complex and numerous. He elaborates on the challenge of dealing with large numbers of cells in digital pathology slides. There can be thousands of cells in these slides, but only a tiny subset might be cancerous or exhibit distinct characteristics.
Alberto then shifts to discussing the applications of large models in radiology and microscopy. Significant work is done in segmenting cells and detecting their changes over time in these areas. He mentions 3D microscopy as an example. He suggests foundation models can automatically segment cells in 3D images. This process involves identifying cells within complex microscopy data.
Robert then discusses the concept of data labeling in AI systems. When data is labeled, it’s like adding pieces of information to images, documents, or any other medium the AI consumes. As the AI “eats” more of this labeled data, it can perform tasks encoded within the patterns it learns from the data.
He points out that AI systems have become more self-supervised, meaning they can figure out labels independently to some extent, reducing the need for extensive manual labeling.
Alberto then goes on to explain how AI can watch a YouTube video and extract labels from the subtitles. By connecting the labels to images, the AI starts to understand the relationship between the content and the labels. This self-supervised learning is similar to how early image generation models used captions to understand the content of images.
Alberto also notes that self-labeling or self-supervision becomes valuable when there is a need to gather a large volume of data quickly, often from the internet or a data repository. It is beneficial when there isn’t enough time to manually label all the data, especially when the output isn’t critically important.
An example he provides is the initial creation of large language models, which involved scraping a significant portion of the internet’s text data. However, he points out that creating these models is more than solely reliant on self-supervised learning; extensive human involvement is necessary on the other side to ensure the models don’t generate harmful or inappropriate content.
In summary, Alberto references the challenge of maintaining high labeling standards to achieve the desired accuracy.
While self-supervision can provide a foundation, the ultimate goal is to guide the AI away from its initial “feral state” acquired from raw internet data and train it to imitate tasks skilled humans perform. The aim is to refine the AI’s capabilities to a level where it can produce outputs that align with the quality of work performed by human experts.
Alberto concludes by emphasizing that while expensive, this investment in high-quality human feedback ultimately enhances the accuracy and reliability of the AI model.
Tailoring AI Solutions to the Unique Needs of the Retail
He points out that the retail industry presents a distinctive set of challenges due to the vast number of products available, each with its appearance and variations. Unlike other computer vision applications where identifying general categories (like cars or motorcycles) suffices, retail demands more specific identification. For instance, it’s not enough to recognize any soft drink; retail leaders might need to identify a particular stock-keeping unit (SKU), such as a limited edition Christmas version of that soft drink.
Alberto then highlights that the abundance of data in retail is substantial and can pose infrastructure challenges. Therefore, having a robust data infrastructure is crucial, including proper tooling and storage methods to handle and manage this vast amount of data effectively.
The V7 CEO also emphasizes that more than traditional classification techniques might be required in retail scenarios due to the sheer diversity of products. Instead, he suggests leveraging techniques like retrieval, where the AI system retrieves specific items based on similarity or relevance rather than assigning them to predefined categories.
Alberto also advises businesses to consider which AI use cases they want to implement in retail. While there might be off-the-shelf solutions available, these solutions often need to be customized and tuned to the specific data and appearances of the retailer’s products. This customization is necessary whether the application involves autonomous checkout, out-of-stock detection or enhancing the online retail experience by recommending products to users.
Robert draws a parallel between warehouses and retail stores. He mentions that the fundamental principles governing the technology used in these environments are quite similar- both involve managing and tracking objects, whether in a store or a warehouse setting.
He points out that the technology powering autonomous checkout in retail is closely aligned with the warehouse systems. For instance, many companies are introducing robotic solutions capable of picking items in warehouses, and there’s a growing trend of guiding human workers about where to locate specific items.
Additionally, there’s a focus on enhancing warehouse safety by integrating autonomous navigation features into devices like forklifts. It is particularly crucial in environments where accidents can have severe consequences due to the presence of heavy objects and tall shelves.
Furthermore, he emphasizes that warehouses also offer potential for automation in smaller tasks beyond their primary function of storing and retrieving items. These tasks include palletization, where items are arranged on pallets for transport, and routine scanning of objects as they move in and out of the warehouse. These processes can be streamlined by using cameras or nearby robots, reducing the manual effort required and enhancing efficiency.
Robert lastly discusses the significance of infrastructure and its upkeep. AI technology could be applied to monitor and inspect infrastructure, such as spotting cracks in concrete or worn-out patches. Quadrupedal robots like Boston Dynamics’ Spot robot check energy infrastructure and send data for AI analysis.
Similarly, AI algorithms within a central hub can identify anomalies in infrastructure data, resembling radiologists detecting medical issues. AI leverages large models to generate comprehensive Health Reports for infrastructure.