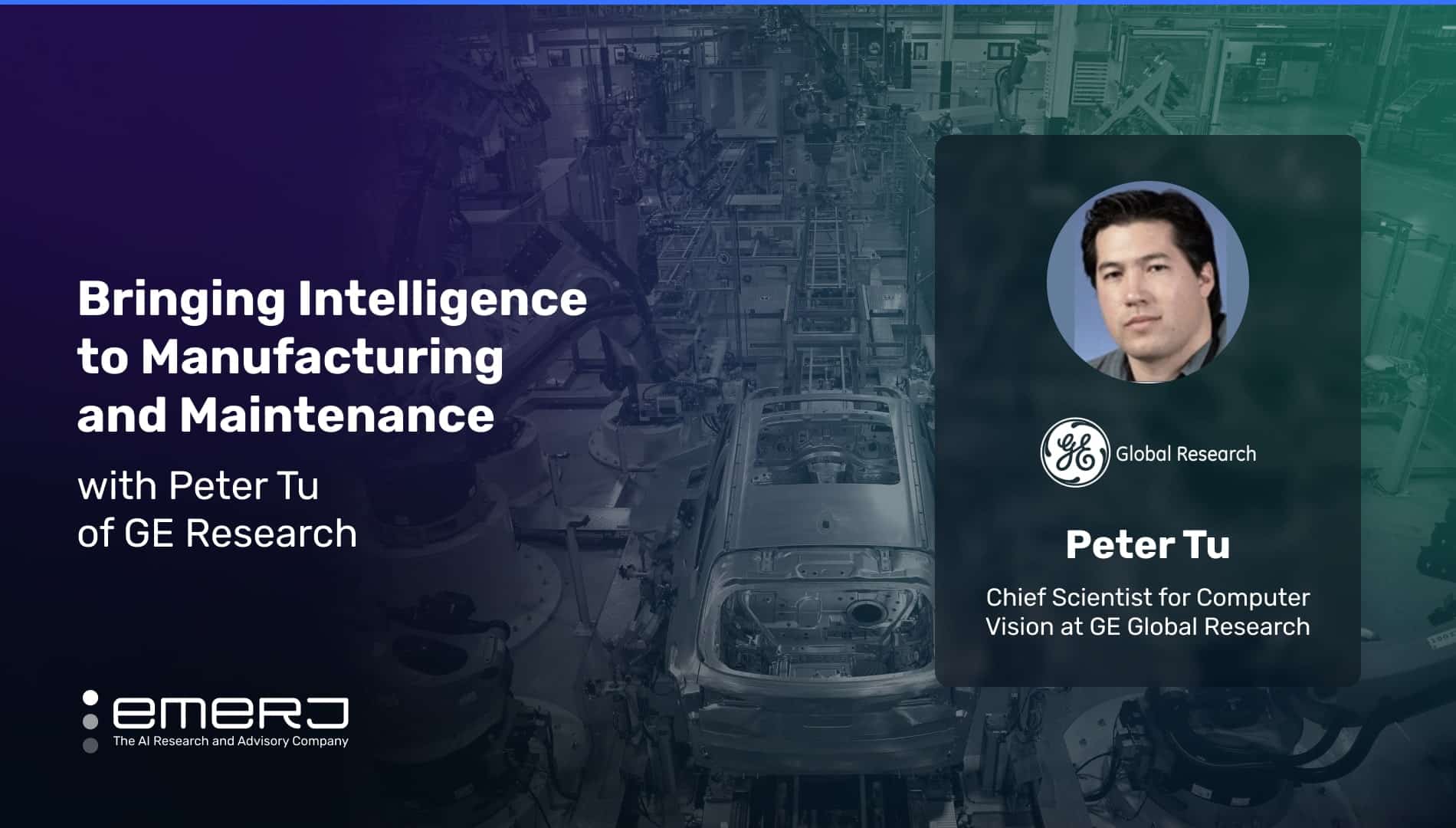
A paradigm shift is happening in the manufacturing industry. Advancement in big data and machine learning is changing traditional manufacturing processes into the era of intelligent manufacturing. The concept of what gets called “industry 4.0” encourages the use of smart sensors, devices, and machines – going beyond the motives of collecting data about production.
One of the most important goals of manufacturing companies is to produce more at minimum costs. That goal is also one that these new technologies are distinctly designed to deliver. Emerj CEO and Head of Research Daniel Faggella recently spoke with Peter Tu of GE Research on the AI in Business podcast about what these developments mean for leaders across all sectors.
In the following analysis of their conversation, we examine two key insights from their conversation:
- Automating physics considerations: Lowering costs and data collection needs by delegating engineering decisions to algorithms trained in physics rules in congruence with business goals.
- Building explainability into AI systems: Making systems explainable from their origins to help humans understand the reason behind decisions made with AI capabilities.
Listen to the full episode below:
Guest: Peter Tu, Chief Scientist for Artificial Intelligence, GE Global Research
Expertise: Video analytics, computer vision, face expression analytics, articulated motion analysis
Brief Recognition: Dr. Tu has helped to develop a large number of analytic capabilities, including person detection from fixed and moving platforms, crowd segmentation, multi-view tracking, person reacquisition, face modeling, face expression analysis, face recognition at a distance, face verification from photo IDs and articulated motion analysis. He has over 50 peer-reviewed publications and has filed above 50 US patents.
Automating Physics Considerations
AI and machine learning algorithms in 2023 can compute data at hyper-scaled speed. While the advanced technology models outperform the human brain in computing data and providing deeper insights, Peter Tu tells Emerj, it will never be able to provide the insights that come with subjective experiences of events.
In order to bridge the gap in providing meaningful data points from subjective perceptions only humans can provide, Peter says that businesses need to infuse business and physics rules into the algorithms to replicate what these systems will feel like to human end users:
“I don’t want to suggest that everything is data-driven. The physics itself is also important. So the understanding of the physics of thermodynamics, of friction of chemical processes material properties, the more and more that we’ve been able to inject that innate scientific knowledge we’ve had into these data-driven models, I think, has given us a significant boost in terms of their robustness.”
– Chief Scientist for Artificial Intelligence, GE Global Research, Peter Tu
Peter also explains that if models are trained in the rules of physics, they require less data to help models understand whatever physical phenomenon they’re trying to detect. Conversely, if we don’t embed systems with knowledge of physics rules, the more data will have to make up for the gap in understanding.
As an extension of this transitive property of data, Peter explains that the more we couple AI capabilities with real-world experiences, the more AI will understand the nuances associated with the experience – even the rules by which the experience occurs in nature. These capabilities have evolved to the point, Peter tells Emerj, that today’s AI-driven manufacturing processes take more responsibility behind engineering decisions across manufacturing organizations:
“Modern and smart sensors control the processes on a shop floor. Machines today have achieved statistical inference and can predict the downtime and tell us how to accommodate the possible downtimes and, further, what to do about the same.”
– Chief Scientist for Artificial Intelligence, GE Global Research, Peter Tu
Peter talks to Emerj about AI’s advancements in the manufacturing industry. He breaks these down into four main categories as follows:
- Visual inspection: When a product is made, it has to satisfy certain specifications in size, weight, and shape, and AI techniques can tell us about the product’s defects, pits, or cracks.
- Service inspection: When heavy products like aircraft go for service, the systems have the potential to detect what is wrong in which part. If there is damage — it needs to be replaced, fixed or serviced.
- Predictive maintenance: Sensors embedded in parts of machines can indicate if the device can go for 20 more cycles or 50. So devices with high confidence can keep working, but the ones with less time to fail need to be serviced immediately.
- Saving costs: Predicting service times saves costs for airlines, for example. Taking an aircraft for service is a loss of revenue for the company.
Predictive maintenance is an approach that aims to improve the manufacturing process’s performance and efficiency by predicting the machine’s downtime or failure.
By using machine learning techniques, predictive maintenance tries to learn from historical data and uses the live data to match and identify specific patterns of system failure. The basics of the approach mean analyzing the live data to find the correlation between parameters that can help predict equipment downtime on the shop floor.
Behind all four categories are AI algorithms and models detecting pivotal metrics and KPIs for every major function of a machine. For instance, when a device like an aircraft engine blade is observed for data collection, the sensor will take images from different modalities, angles and pixel bases.
The pictures and visual data processed will lead to a report outlining whether the criteria of size, weight, and other dimensions of the blade were met. It will also note if those images have changed since the last inspection, if there are any dents or cracks, how severe the damage is and if it needs to be fixed immediately.
Peter points out that – while subjective human observations are essential to data collection – their limited senses mean the data points they influence should be viewed with appropriate skepticism. Often this means drastic differences in how “objective” data points influenced by humans really are, as they are influenced by subjective factors such as the level of skill of the subject matter expert and their interest in the job.
In discussing where AI is headed in the future, Peter tells Emerj that the next phase of these technologies will be marked by “AI in the wild,” meaning algorithms that can make decisions when unanticipated situations arise. He offers the following vision of what that might look like:
“If an aircraft is flown through a volcano, it will be exposed to ashes. It is a very unlikely situation; hence the machine may not be prepared for it. But now that it is happening, what will AI do? Will it understand what it means chemically, and how will the ashes impact the aircraft”
– Chief Scientist for Artificial Intelligence at GE Global Research, Peter Tu
These questions will challenge even humans and pilots in this case. However, humans can think and react effectively in situations they have not faced before. The question Peter asks here is: will AI eventually be able to do the same?
He tells the podcast audience that, in continuously emphasizing the fusion of both physics and business principles in algorithmic models, he finds he and his colleagues are frequently asking themselves, “Can we ground AI in these concepts sufficiently that it understands what is important in the present time and makes all the right decisions as a human would?”
Building Explainability into AI Systems
Peter emphasizes the need for explainable systems in manufacturing. Citing an example from the aviation sector, he says an engineer should know why a specific device in the engine was turned off automatically — they would like to see the explanation of the decision a machine made.
“If your AI is a black box and makes a decision that is not explainable, then there is a significant risk in putting those models into production. Because, as the output or the result of the black box, lives could be lost and assets could be destroyed.”
– Chief Scientist for Artificial Intelligence, GE Global Research, Peter Tu
Black box systems are closed systems that receive input and produce an output but do not explain the reason behind the output. Since self-learning systems learn from the surrounding environment and past mistakes, programmers struggle to understand intelligent machines’ internal logic and decision-making.
Explainable AI is essential to:
- Generate trust and transparency
- Mitigate risk
- Ensure compliance and regulations
- Generate accountable and reliable justifications
- Minimize bias, unfairness, and misinterpretation
The fundamental reason for explainable AI is to have machine learning and AI systems make decisions that humans can easily understand and track back to their origins. The goal of explainable AI as a business value is to make systems that are persuasive as a consequence of end users understanding how AI works, the mistakes it can make, and the safety measures around it.
The objective of having an explainable system is to have transparent, confident, and trustworthy AI. A company may have a great AI model at its disposal, but if it is a black box, it is useless, Peter tells Emerj.
As noted in a recent research report by Deloitte, implementing and building explainable AI is a multifaceted process that requires changes in data sources, model development, and governance processes.
Peter tells the Emerj podcast audience explainability is especially important for critical systems carrying life-or-death implications — like aircraft. If an engine automatically turns off on its own in inappropriate circumstances, the implications of AI capabilities being involved are especially serious and easy to overlook through the lens of black box considerations.
Another example Peter cites comes from an inspection point of view. When the aircraft go for servicing, the sensors tell the engineers if a particular part has to be replaced or serviced. In these circumstances, the algorithm must be easily understandable enough to convince the engineer that the detection outcome is authentic.