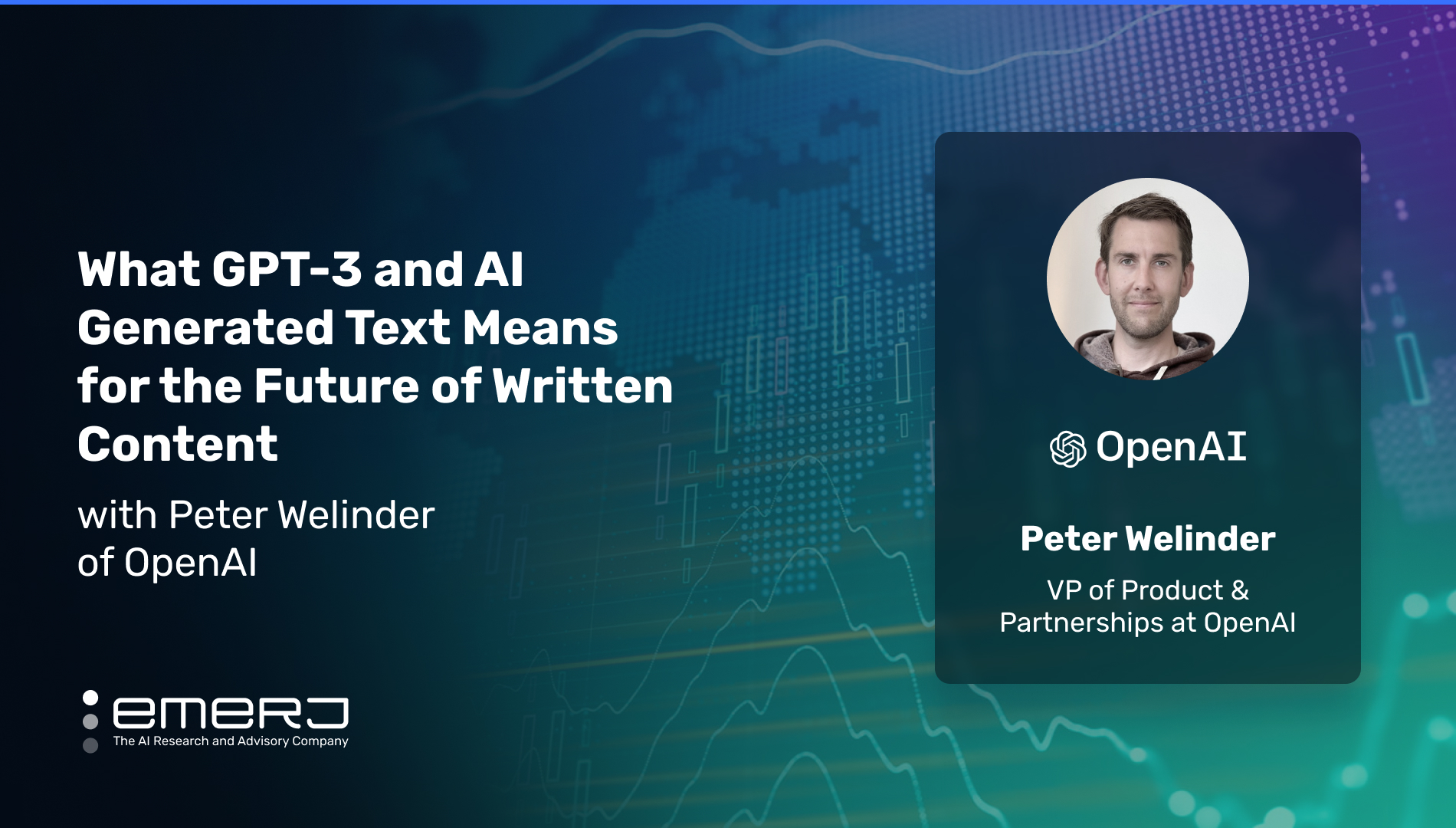
It is not hyperbolic to state that the autoregressive language model known as GPT-3 (short for ‘Generative Pre-trained Transformer 3’) is an unparalleled evolutionary step in content creation. In fact, the potential outputs the model can produce are so advanced that many academics have cited GPT-3 as Exhibit A in calling universities to reconsider what constitutes academic plagiarism.
In the business world, enterprises all over the globe are using GPT-3’s platform to design innovative products and solutions, most notably content creation applications. Example applications of the technology include: automated customer service, report generation, sentiment analysis, knowledge management, and machine translation.
Peter Welinder is OpenAI’s VP of Product and Partnerships – and one of the principal designers of GPT-3. Peter recently sat down with Emerj CEO Daniel Faggella on the AI in Business podcast to discuss three ways that GPT-3 is changing written content creation and consequent effects:
- Expanding content creation possibilities: From summarizing large pieces of text into question and answers to writing product descriptions, GPT-3’s potential content creation uses cases are extensive.
- Demonstrating that elaborate models can be simple in design: While the hardware engineering of GPT-3 was complex, the underlying model became simpler as time passed and as the machine became adept at predicting user actions
- Enhancing educational delivery: Advanced language models like GPT-3 are delivering more personalized, effective educational solutions to both enterprises and individuals, with the potential to change the very framework of education delivery and consumption.
Listen to the full episode below:
Guest: Peter Welinder, VP of Products & Partnerships, OpenAI
Expertise: Machine learning, computer vision, robotics, software engineering
Brief Recognition: Before becoming OpenAI’s VP of Product & Partnerships, Peter founded the robotic research department at OpenAI while leading the product, engineering, and research teams GPT-3 AI from the prototype phase to the product launch. Peter also served as the Head of Machine Learning at Dropbox for over two years and led the computer vision and Dropbox Photos infrastructure teams. He holds a Ph.D. in Computation and Neural Systems from Caltech.
Expanding Content Creation Possibilities
In terms of use cases, GPT can take on more diverse and complex tasks than many — if not all — language models. Peter gives real-life use case examples, including:
- Translating a sentence into French,
- Asking about the sentiment of a movie review
- Extracting questions and answers from articles.
In each case, GPT’s model can perform the task quickly and accurately after being prompted.
”What became really amazing is that you could also apply [the model] to other use cases that just didn’t exist … We’ve seen our users apply it to things like extracting questions and answers from articles for summarizing pieces of text or doing semantic search in large document collections to doing things like writing great product descriptions or even like writing assistance or coding assistance.”
– VP of Products & Partnerships at OpenAI, Peter Welinder
Another use case of GPT-3 that Peter mentions is summarizing customer feedback. Here, Peter provides an example of a concrete use case at Viable, which automates the analysis of qualitative customer feedback. In this case, the company uses GPT-3 as its platform’s centerpiece for processing thousands of pieces of feedback. The result was a model that improved the accuracy and quality of customer feedback summaries.
Throughout the conversation, Peter discusses the importance of customizing and refining the use case model and increasing the flexibility of potential use cases. He explains how he compares the potential use cases with classification models, “While we found that GPT-3 is extremely good at classification tasks, it’s better once you start customizing the model.”
Yet where GPT-3 really demonstrates its prowess in Peter’s view is in the text generation realm and particularly in summarization. He cites the following use cases:
- Creating copywriting applications
- Writing product descriptions
- Providing content ideas for writing
- Summarizing large content pieces
However, the model is capable of far more than generating content. A good example is a use case where Peter describes how GPT-3 models are embedded into a product at Keeper Tax, a San Francisco-based tax filing software company:
”They declassify financial transactions that you have on your credit cards, and so on, and help you find things like write-offs. At the beginning, GPT-3 was maybe 85% accurate. Over time, they added another percentage point every week by having the model do some classification, have some humans correct, then feed that [data] into the model to do another customization with the new data. And then it gets better. The model is pushed to a level where it makes very few mistakes.”
– VP of Products & Partnerships at OpenAI, Peter Welinder
Demonstrating that Elaborate Models Can be Simple in Design
Interestingly, as the number of use cases for GPT-3 continued to grow, the model itself became simpler, Peter explains. While the more complex use cases required additional training data, thus increasing the model’s size, the underlying engine did not change:
“So, when I talked about these models before, like the translation models or the sentiment classification models, they were specialized down to how they were engineered for that particular task,” Peter tells Emerj. In contrast with GPT-3, they were “trained in a really simple way, where we basically collect a lot of text on the internet … and then the model is a big neural network.”
Of course, as the number of users and use cases expand, so does the underlying data corpus in which the model is trained. Starting with the scraping of “enormous amounts of text that we found on the internet,” says Peter. Over millions of CPU hours, naturally, the model improves its ability to predict the next user step.
The result is that GPT-3 grows in its ability to predict what to do next without manual intervention or additional engineering. The model was “rewarded” and, in that way, learned what to do and not to do.
Yet despite the resulting simplicity, in no way was the GPT-3 initially easy to design – requiring thousands of CPUs and “a ton of engineering.”
Despite the facility of user design, Peter cautions users in how they instruct the model to do something, and cites using the model to come up with a recipe as an example: “You [would] write it in the same format as you would expect it [to be written], and then the model would pick up on it and kind of do it.”
In other words, “be careful what you wish for” – as the saying goes, or in the case of generative AI: users need to be very careful of what they’re putting in the text prompts to avoid unwanted outcomes.
Further output refinement requires additional stores of training data and fine-tuning of the particular model.
“I think when you do these kinds of generative use cases, oftentimes, in order to get it to the right tone that you want, customization is really useful for adding more data, adding more examples of the sort of time you want,” he notes, elaborating on the importance of customization for text summarization use cases.
Enhancing Educational Delivery
The podcast moves forward with Peter and Daniel discussing OpenAI’s and GPT-3’s educational capacities. Peter mentions the Swedish education company Sana Labs, which uses and fine-tunes GPT-3’s customized models to develop education material.
More specifically, Sana Labs uses a GPT-3 model to read the content of various learning material and then generate questions and answers based on this material. In this way, students are not forced to read through copious amounts of superfluous information and can be tested on the main tenets of the material – which is already happening anyway.
Peter elaborates on where he thinks GPT-3 and other AI technologies can make a positive impact in education. One area is giving each students their own individual AI teacher and how this personalized delivery can lead to better student outcomes.
He references Bloom’s 2 sigma problem to provide some context on his opinion:
“[Bloom’s two sigma problem] was a really interesting study … it is this idea that if you have a class of students and a teacher that teaches them, you will get a certain average score of the people in the class. But if you have each student a personalized tutor and teach the same subject, then they will all end up at like the 90th percentile in terms of scores. You know, of course, we cannot give a teacher to any person on the planet. Well, with AI, we have that opportunity. I think it’s so exciting.”
– VP of Products & Partnerships at OpenAI, Peter Welinder