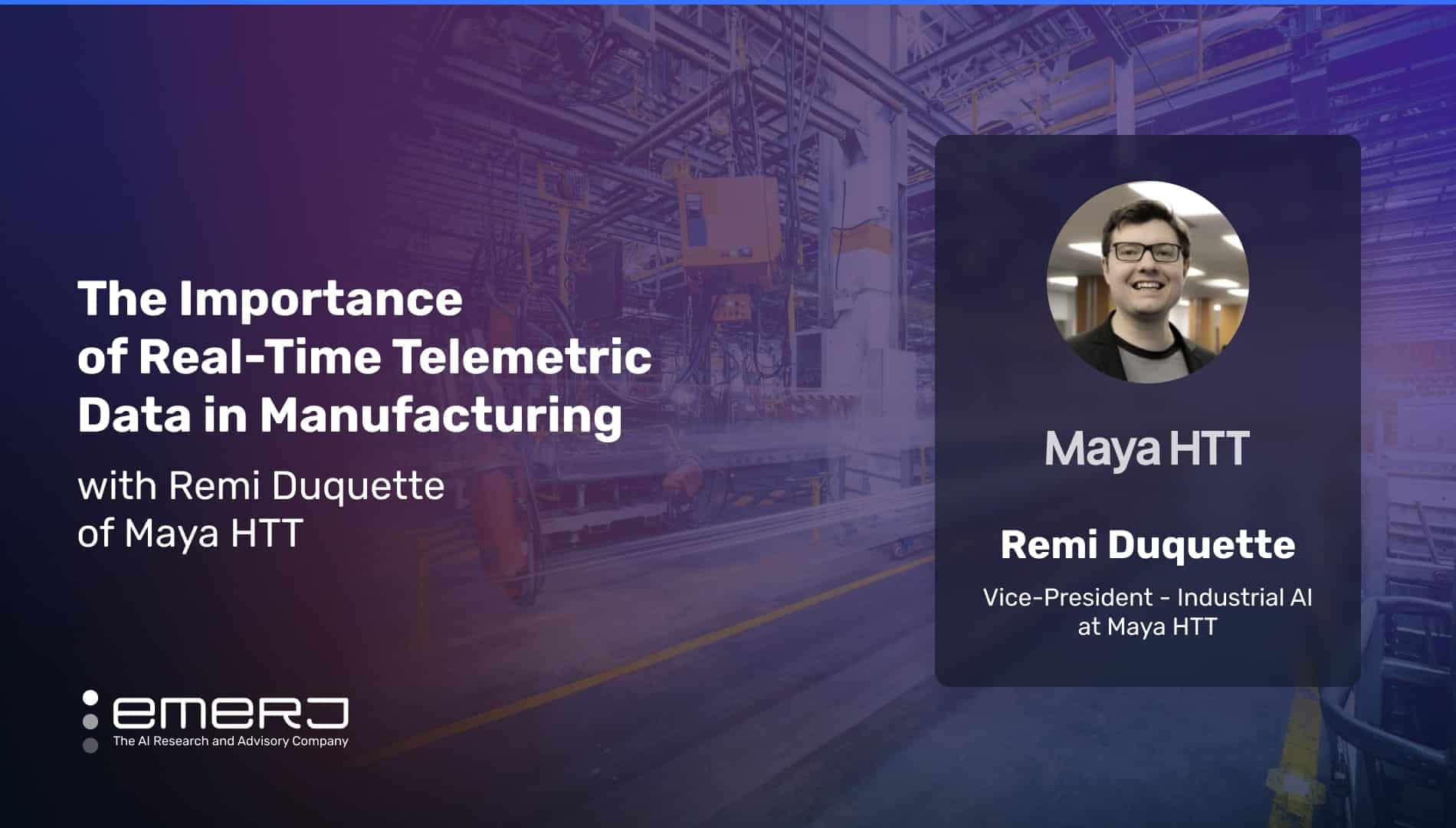
The manufacturing industry has changed in recent years. Humans on the shop floor have always used their senses and experience to anticipate machine failure before it occurs. Now, AI can be used in conjunction with human expertise.
Safety is also an important consideration in the heavy industry space; the shop floor has new hazards that didn’t exist a decade ago including robots and semi-automated and fully-automated moving equipment. AI has the potential to minimize or eliminate the risks posed by these machines.
According to the Harvard Business Review, one way to improve AI – especially in heavy industry – is to augment data with human intuition and insight.
Where does that needed data come from? We will address that question in this article. For Maya HTT, that data can be derived from both human and machine sources and combined in an approach known as sensor fusion, which we expand upon below.
For a deep-dive into using computer vision and machine listening in manufacturing, we spoke on the AI in Business podcast with Remi Duquette, Artificial Intelligence Lead at Maya HTT, an AI services firm.
In our 27-minute interview, we explore how AI can augment human capabilities in both forecasting machine failures and keeping workers safe on the shop floor. We also explore how to determine high-ROI opportunities from sensor fusion.
This article will highlight and examine three key insights from our conversation with Remi that are relevant to business leaders implementing AI in manufacturing:
- Forecasting shop-floor failure: Using machine listening and vision to detect subtle shifts in sound pressures, temperatures, and frequencies in active equipment.
- Improving worker safety: Computer vision can identify potentially unsafe work zones and send alert notifications to workers.
- Criteria for pursuing ROI from sensor fusion: Along with traditional considerations of cost and impact, safety considerations are essential to identifying reliable ROI opportunities from sensor fusion but often overlooked.
Listen to the full episode below:
Guest: Remi Duquette, Vice President of Innovation and Industrial AI, Maya HTT
Expertise: Sensor fusion, machine learning, deep learning, machine vision, machine listening
Brief Recognition: Previously, Remi was a Structural FE Analyst at EMS Technologies. He has been with Maya HTT for over 20 years and VP for the last 6 years.
Forecasting Shop-Floor Failure
Machine vision and machine listening allow companies to go beyond human senses and improve predictive maintenance and quality in the manufacturing process.
Machine vision comes from three different sources collectively called “video as a sensor”. These sources include:
- 2D cameras that produce streaming images.
- Stereographic cameras that add depth to the pixel.
- Thermal imaging cameras containing sensors that capture temperature distribution.
Remi elaborated, “Separately, you have a thermal imaging camera. So, if you put a thermal filter in there with a nice little sensor, you can get the temperature distribution of what you’re looking at. So there are really visual inputs.. We call them video as a sensor in this context.”
Machine listening involves leveraging sounds to identify machine misbehavior or early signs of machine failure. Shop floor workers often rely on the vibration coming from a machine to recognize that the machine is about to fail.
The issue with relying on human senses is that by the time you get to a point where the machine has a change in vibration, it’s too late by that point; failure is imminent. “Once you get to a vibration of a machine that’s a pretty good indication that the hard failure is about to happen at that time,” explained Remi.
Machine listening can augment those human capabilities by detecting early signs of failure onset. AI can detect subtle shifts in sound pressures and frequencies that are not detectable to the human ear. While forecasting failure can improve machine longevity, it can also improve worker safety because a worker won’t have to speedily repair a machine that has already failed.
In addition to sound, computer vision – including thermal imaging – can also help to forecast machine failure. Electric failure in a piece of equipment can cause the machine to heat up beyond a normal threshold. With proper sensors, the machine can be repaired before the failure.
To understand the new capabilities that emerge from the fusion of different data sources Remi explains:
“The integration of all those sources of interesting data, once we’ve cleaned them up, of course, in a reliable way can definitely solve a lot of complex problems that humans may have been able to solve in some ways, but not completely. And the new ones clearly are adding value here to the shop-floor workers.”
– Vice President of Innovation and Industrial AI at Maya HTT, Remi Duqette
Improving Worker Safety
Remi emphasizes that there are numerous hazards on the shop floor that simply weren’t present a decade ago. Robots used in manufacturing are commonly present on shop floors, and they present a risk to humans nearby.
Companies can use AI when looking at a specific field of view to track the humans in the scene and ensure that they are not in areas where they could get hurt. “So having that kind of camera that will kind of depict those and send safety notifications to shop-floor workers can become a very interesting safety and security use case that we’ve seen happen,” Remi tells Emerj.
Computer vision in manufacturing goes well beyond what humans can easily see with the naked eye. The two areas in manufacturing that people usually look for are:
- If a part is falling off
- If there is a crack present
In the manufacturing industry, computer vision can be used to see “heat.” In this way, computer vision can also be used to help ensure worker safety.
Through the use of thermal imaging cameras, AI can detect a small electrical fault in a piece of equipment. As Remi describes:,
“So it could be like a small electrical fault in a piece of equipment that is heating up the side of a panel. Of course, you’re not seeing that inside the machine that some electrical failure is happening and it’s heating up that corner of a panel and if you were to touch it with your human hand you would probably burn yourself.”
– Vice President of Innovation and Industrial AI at Maya HTT, Remi Duquette
Criteria for Pursuing ROI from Sensor Fusion
It’s common to look at how humans solve problems and try to replicate that with AI. However, as Remi explains, untapped potential emerges when we use input that machines can detect:.
“Sensor fusion is exactly what it sounds like. It’s combining various sensor inputs, whether it’s the video inputs with a real time telemetry input, and an audio sensor that will give you the sound of what’s happening in this environment. And you combine them in a logical fashion, so that you know that the sum of the three parts are actually getting you to a decision that you would not otherwise be able to take with, you know, the individual parts.”
– Vice President of Innovation and Industrial AI at Maya HTT, Remi Duqette
Remi tells Emerj from his experience that use cases have to be evaluated to determine which ones are candidates for applying AI. The commoditization of sensors has opened up the possibilities in the last four years.
“So it’s very interesting,” He Remi offers on the podcast. “Because it’s becoming easier and lower cost [sic-ed.] to deploy additional sensors in the world right now with mesh networks and other things that you can put on top fairly next, inexpensively in your shop floor.”
Thermal imaging cameras have come down in price as well. Even lower-cost thermal imaging cameras that range in price from several hundred to several thousand dollars can be calibrated to be used safely.
Remi emphasizes that AI can be used to improve worker safety, but worker safety can be the deciding factor in nudging a project forward that wouldn’t otherwise qualify based on ROI.
He tells Emerj that Maya HTT starts by creating a roadmap for clients and looking at costs, grouping it into small, medium, and large. ROI can also be grouped into green, yellow, and red.
In addition to the cost and revenue ratio, safety and security is considered as a third dimension. Adding in safety and security concerns increases the opportunity for sensor fusion in cases where the business ROI wasn’t high enough to move a project forward.
Safety and security might be intangible and not easy to quantify but are extremely important because human lives are at stake.
Thermal imaging points and feeds can be added to both 2D and 3D cameras that have been deployed. “And now you have, you know, amazing new capabilities based on saving people’s lives or at least not getting them injured,” according to Remi.