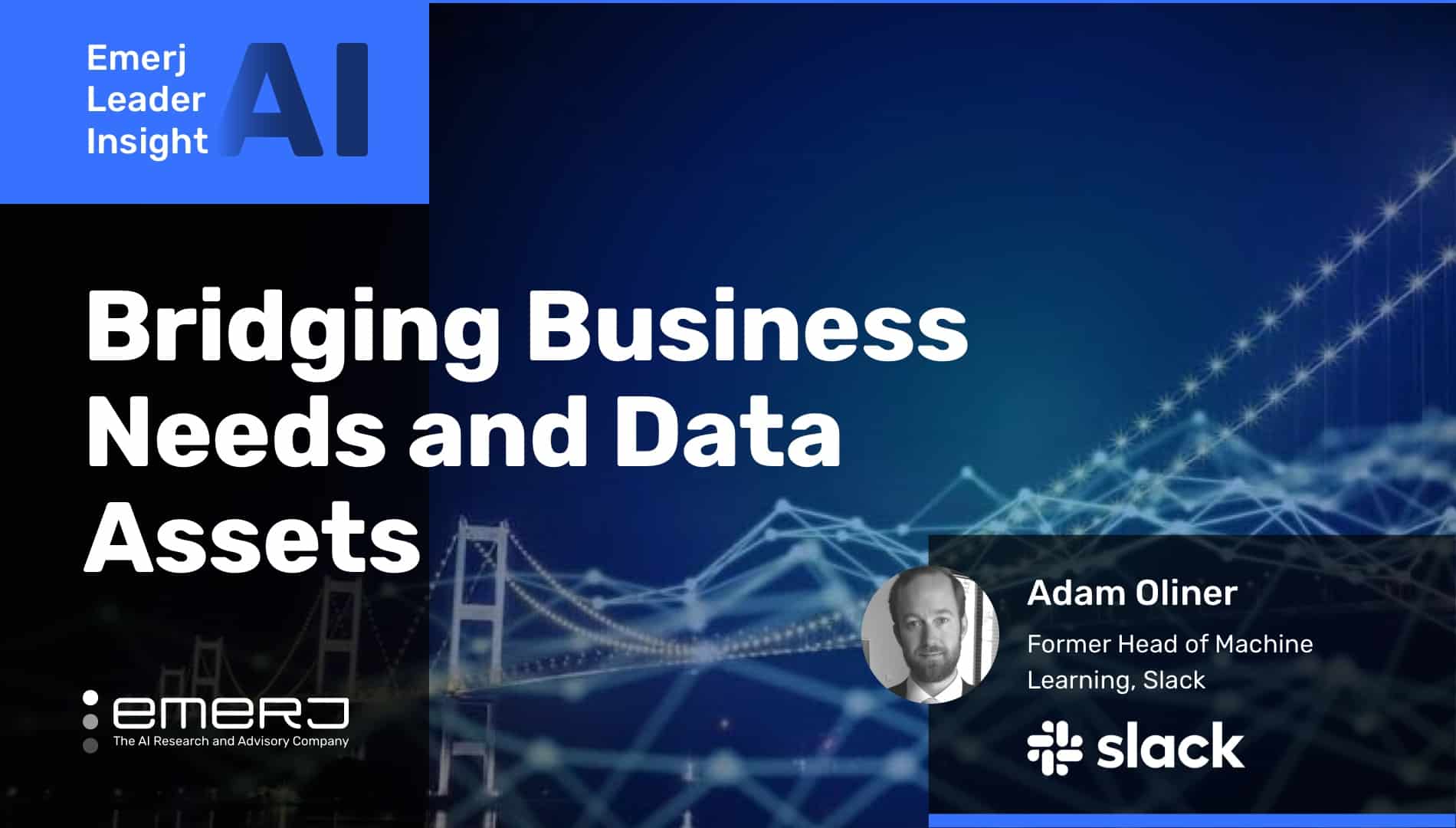
In the vast land of opportunities that AI creates, how do we select the projects that will generate ROI? Do we gain inspiration from reading AI use-cases relevant to our industries? Do we search through our own lists of existing priorities and hope the applications for AI will become clear?
In May, we sat down with Adam Oliner, Slack’s then-Head of Machine Learning. An industry expert who ran ML for one of Silicon Valley’s most successful start-ups, Oliner took us through the process to discover AI opportunities by bridging business needs to data assets. He then gave us five steps to help us navigate our own unique universes of potential applications for AI and form the connections between our business problems and the galaxies of data scattered among them.
“I think about it as a constellation of potential business problems that you would want to attack with AI on the one hand and a bunch of data on the other hand. And the process of assessing those business problems, assessing the data, and then assessing the potential bridges between the two,” says Oliner.
This article summarizes those five key takeaways that help business leaders identify their best AI projects based on what they need, what they have, and the tech that’s available to them.
1. List Well-Defined Business Projects
In the vast constellation of potential AI projects, look for well-formed problems with measurable impacts, advises Olin. An AI project created to ‘understand the business better’ might gain some traction in the boardroom. Down the road, however, questions will emerge:
- How will you define your project?
- Which measurements will you use?
- How will you define success?
- What will the end-state look like?
A better AI project might target reducing the time it takes for a worker to complete a process. AI excels when it looks for user friction and dead ends. It can spot repetitive tasks more effectively than humans.
When you identify initial projects, look for potential AI applications within your workflows that frustrate employees and end-users. Start small and simple. Improving an incremental repetitive workflow will be easier to accomplish (and get traction for) than launching an entirely new project or feature.
2. Determine Data Needs
Do you have the data you need? Once you’ve defined your potential business projects, compare the data you have with the data you need. Repetitive workflows generate structured user data. When you evaluate that data, ask if it is:
- Clean
- Reliable
- Timely
If you’ve got clean, reliable, and timely data, you’re in great shape to proceed. Careful, however: choosing an AI project just because you have ample data might lead to the low-hanging-fruit variety of business insights … those that don’t yield much business value.
Advances in technology have made it easier to work through data shortages. Even though modern ML no longer requires a table, the further you get from this ideal state, the harder your project will be. You can also sometimes log and collect what you need—even relying on services like Mechanical Turk.
3. Determine Bridges Between Data and Business Needs
With your list of projects and your data assessed, it’s time to build the connections between the two. We build the strongest bridges when a project has:
- High business value
- A well-defined problem
- Available, clean data
Beyond that, we also need infrastructure and expertise. Your project team will need a firm understanding of the ML methods needed to get the job done.
ML applications break down into two buckets: predictions and clustering. These two categories cover the vast majority of machine learning applications.
“You can be led to believe that ML has to be really complicated or difficult,” says Oliner, “but, at its base, there’s a small number of fundamental capabilities and if you understand those you’re off to a great start.”
4. Assess Relative Costs and Requirements
When you compare your well-defined business projects against your data needs and strengths, every project on that list can look like an ROI winner.
But, they’re not all winners. You still have to assess the costs and requirements of each … and test the strength of that bridge linking your business needs to your data assets. Among the costs you will want to consider:
- Overhauling data architecture
- Physical hardware and software
- Purchasing third-party data
- Talent acquisition, onboarding, training
When you evaluate the costs of potential AI projects, consider that you can reuse AI components and save money. “Some people have hooked together forecasting and clustering and prediction in complicated ways where now you have self-driving cars or something like that, but a lot of the time, those solutions are reusable,” says Oliner.
For example, a project leader downloading a pre-trained object recognition model can then just show it objects—without incurring the cost of rebuilding it with their own time and resources. As another example, if you already have a system that predicts which option a user is likely to choose from a pull-down menu, the cost of an initiative incorporating that AI will be substantially lower. Oliner advises typing a concept called a feature store into your search engine as just one way to see the breadth of ML features available today.
5. Rank AI Opportunities
In this last step of the process, rank your AI opportunities. Consider costs and timelines, resources you will need, and your in-house expertise. Through this process, determine which projects move to the front of the line and get presented to your decisionmakers. The others remain on the list for later. Or they drop away from consideration.
When you rank your AI opportunities, consider economies of scale. Which common ML capabilities exist across different business problems? If you have a class of business problems, that might be a bridge worth building. While you’re building your list of business problems that AI might solve, if you see many that involve “recommendations,” for example, you might want to invest in a general baseline capability that can be used in different parts of the business.
Conclusion
Throughout the process, you need a cross-functional group of people in the room with you so you can drive towards well-informed decisions that reflect the experience and knowledge of the business and not just one key employee.
For example, with data, “Someone like me is not sufficient (by myself),” says Oliner. “Even with someone who is an absolute expert in all of this will not necessarily be able to tell you exactly what data is required for one of these problems.
“For team composition,” he continues, “I think it’s more about skillsets than roles. You need folks who understand the business, the subject matter, the data, and the ML capabilities.”
As you move through the process to discover AI project opportunities within your own company, make sure you have not only smart SMEs in the room, but also business people to opine on financial implications and data scientists to explain the depth and breadth of data available in an organization.
“Start with the business problem,” Oliner advises, “and then think about what are the possible bridges to get you there, and what data do those bridges need to get started.”