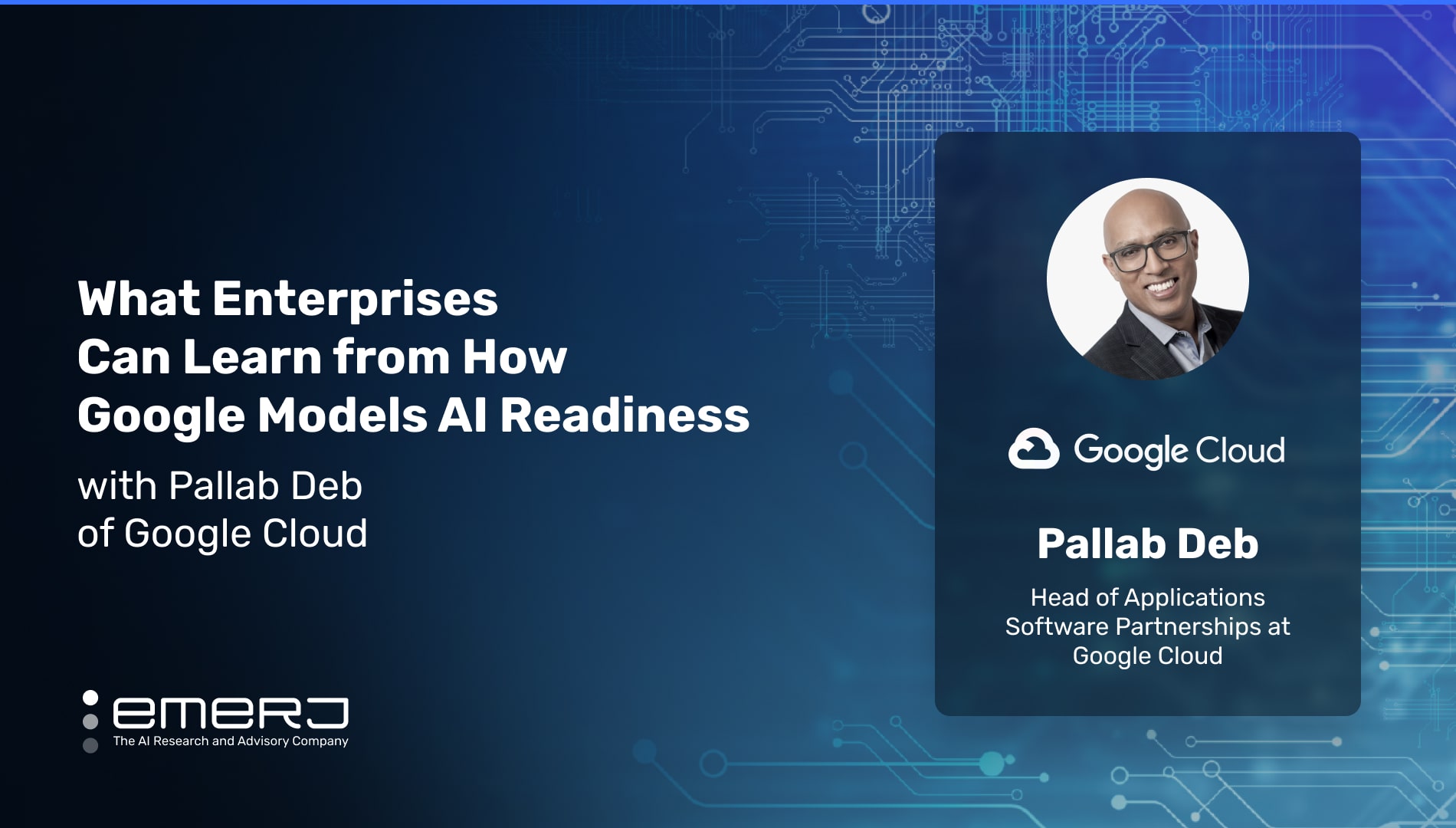
It is exceptionally difficult to get started, much less succeed, in AI adoption if one does not have at least a foundational education, particularly in those “modules” pertaining to AI capabilities, requirements, and organizational readiness. A business wanting to implement AI must understand the current state of adoption and how this current state matches up against AI readiness requirements.
This isn’t easy – and the more sizable and complex one’s organization is, the more difficult it is to ascertain AI readiness. So, the question is, “How do I get started?” A perfectly rational query – and one that’s perfectly suited to Google’s Palleb Deb, a recent guest on the AI in Business podcast.
Pallab is the Director of Partner Solutions & AI Partnerships at Google Cloud. In this role, Pallab is responsible for leading a global AI business development team on strategic partnerships and new business models for leading telecom companies, banks, and industrial automation enterprises. In his role, Pallab’s team consults with enterprises every day about implementing AI into its current operations.
Google is a corporation that has managed to frame and adopt using a simple yet comprehensive framework despite its large size. We spoke with Pallab on the AI in Business podcast about how a leader could apply a similar AI maturity model to their own company’s AI strategy.
In this article, we analyze two key insights from our conversation with Pallab:
- Using an AI maturity scale to assess readiness: Using a framework that measures organizational preparedness to adopt AI through the lenses of personnel, management strategies, access to data, data security, and automation policies.
- Transitioning between maturity phases: Organizations will begin AI projects in a period of short-term solutions for narrow before they can provide sustainable – or even transformative – value for the business.
In each section, we will provide concrete documentation and summarization for both Google’s AI adoption framework and the three phases of AI maturity Pallab explains on the podcast.
Listen to the full episode below:
Guest: Pallab Deb, Head of Partner Solutions and AI Partnerships, Google Cloud
Expertise: IT strategy, enterprise software, cloud computing, SaaS, business intelligence
Brief Recognition: Prior to moving through the upper ranks at Google Cloud, Pallab was the VP and Head of Data, Analytics, and AI at Wipro.
Using an AI Maturity Scale to Assess Readiness
Pallab begins explaining Google’s AI maturity assessment framework with the maxim you should start with the end in mind and calibrate your approach from there. Accomplishing this requires an understanding of where your company is in terms of AI maturity.
An essential first step along the AI maturity path is bringing any groups working on “stray” AI projects to the table. Pallab emphsizes that gathering a team of early AI adoption champions and key stakeholders is needed to ensure all necessary areas of the business are on the same page, to bring some organization to future processes. Moreover, these individuals may have some valuable insights and lessons to share regarding AI learning and execution.
For an idea of who these individuals might be in the organization and how to hire or recruit for them, refer to Emerj’s recently published AI executive guide, “Seven Non-Technical Enterprise AI Career Paths”.
Pallab shares the AI maturity tool that Google uses to assess readiness. The AI Adoption Framework tool includes the four pillars of people, process, technology, and data.
Each of the abovementioned four elements produce a comprehensive yet concise and easy-to-follow readiness instrument. Pallab states that there are two good, practical uses for the instrument: assessing project readiness and calibrating performance against an existing project.
Below are the five elements and the elemental questions to be answered (please see the above-cited PDF for more specifics on how to use the model):
- Learning:
- What data and machine learning skill sets are required?
- What data science and engineering roles should be hired?
- To what degree do learning programs mirror business needs?
- Leading:
- To what degree are your data science teams collaborative, cross-functional, and self-motivated?
- Do your data science teams have executive-level empowerment and sponsorship?
- How are AI dollars allotted?
- How are projects assessed and governed?
- Access to data:
- How is your dataset annotated, created, and curated?
- Who owns the dataset? Is it discoverable and usable?
- Are trained models, notebooks, and other machine learning components and solutions sharable, reusable, and expandable
- Data security:
- What are the data controls in place?
- What are the governing strategies?
- How do AI capabilities establish organizational confidence to be leveraged to drive business value?
- Automate:
- What triggers a process?
- How is data lineage tracked?
- Are data pipelines fault-tolerant and resumable?
- How are logging, monitoring, and notifications managed?
Progressing Along the AI Maturity Roadmap
To understand some of the terminologies Pallab uses, we must quickly explain how Google Cloud defines the levels of AI maturity. There are three phases: Tactical, Strategic, and Transformational – described in-depth below.
Tactical:
- Simple use cases are found but are typically short-term solutions with narrow applications.
- A comprehensive plan and strategy for scaling are likely absent.
- The organization typically focuses on easy adoption, minimal disruption, and quick wins in this phase.
Strategic:
- AI is being used to deliver sustainable business value; several machine learning systems are in place that leverage both out-of-box and custom modeling.
- A broader vision concerning AI adoption is found at this stage; perception is acute to the degree that hype is discarded, and the technology is evolving as a prominent business accelerator.
Transformational:
- AI is central to the organization because it stimulates innovations, supports business agility, and creates a culture of experimentation and learning.
- At this phase, machine learning expertise is dispersed across lines of business.
- A strategy is in place for scaling and promoting machine learning capacities throughout the organization.
Enterprise leaders might recognize the contours of these phases from Emerj’s recent article on the three phases of organizational transformation through conversational AI.
So, what does it take to evolve from a tactical to a strategic company? Pallab answers this question through the lens of the following:
- The type of leadership and vision that is needed
- The initiative’s scale and resultant human capital requirements
- Workforce education of the tech to be introduced
- Cultural and operational mindset changes
First, the company should have a dedicated executive role – e.g., Chief Data Officer or CDO – that determines the accessibility and operation of AI and data assets within the firm.
Second, it is necessary to ask questions regarding the application(s) scope:
- How far-reaching is the application?
- Is the initiative on the departmental level? The organizational level?
Answers to these questions determine what Palleb calls the “talent imperative,” or the degree and diffusion of knowledge required to run and technically maintain the AI.
Next is ensuring data quality, security, and access. Palleb states that this is essential to refrain from investing time and money in a substandard solution that does not elevate human decision-making:
“I would say that the majority [of AI projects go] from popcorn projects to the strategic curriculum is about hardening your process; harden the way you look at it, how you’re going to use your technology, how you’re going to enable your people, [and] how you’re going to use leadership to drive that as a key priority for the company. And then, of course, use as much tech as possible. So people are doing valuable stuff, and you’re automating the mundane.”
– Head of Partner Solutions and AI Partnerships at Google Cloud, Pallab Deb
What about the leap from the strategic phase to transformational? That’s an entirely different ballgame. “This is literally about stepping back from what you are doing today,” says Palleb.
At the transformational level, decisions directly impact both customer experience and competitive advantage. Palleb provides a hypothetical example of a digital native company with AI-powered inventory capable of tracking its supply of colored pencils down to individual red, blue, and green colors. The company’s competitors, meanwhile, monitor inventory level by pallet, and thus have yet to determine whether what they have in stock is red, green, or yellow.
The digital native company has a distinct competitive advantage in that it can:
- Offer the specific product the customer wants.
- Guarantee that it is in stock.
- Tell the customer when they will get the product.
“So, your organization would have built all its systems thus far to ship products, buy pallets, track the movement, and measure the inventory by pallets. Now, in the e-commerce world, you are trying to give people the ability to order red pencils and know that you have that in inventory. Completely different mindset, right? And this is where I think data nd AI can drive that transformational change. But that is not just tech change. It’s a culture change, it’s an operating model change, it’s a business model change…”
– Head of Partner Solutions and AI Partnerships at Google Cloud, Pallab Deb