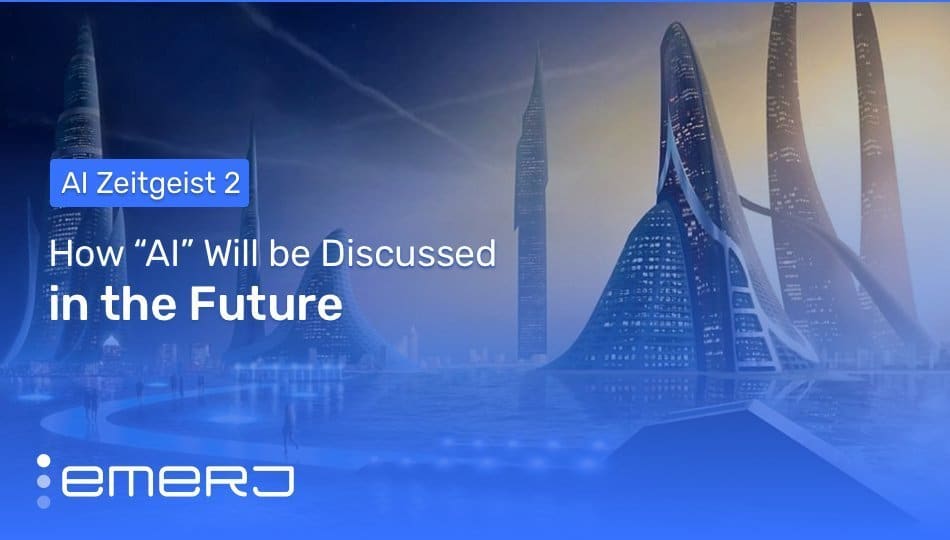
This article is part 2 of a 7-part series called "AI Zeitgeist," where we'll be mapping out the details of AI adoption over the next 10 years and explore the critical changes in the AI ecosystem that business leaders need to understand.
In this installment of the Zeitgeist series, we'll be exploring the ways that the terms "AI" and "machine learning" will be used and discussed over time—from the buzzword of today (in the "Emergence" phase) to the invisible tech layer of a decade from now (in the "Dispersion" phase).
(Note: If you haven't read the first article in the AI Zeitgeist series, you should familiarize yourself with it first. In that article, I explain what the 3 phases of "Emergence," "Adoption," and "Dispersion" actually mean).
We'll start off by listing a few company names that probably sound funny to you:
“International Business Machines”
“Apple Computer Company”
You don’t know those names instinctively, but you know what the companies are called today:
“IBM”
“Apple”
Wh...
You've landed on exclusive content for Emerj Plus Members
Emerj Plus Membership
In-Depth Analysis
Consistent coverage of emerging AI capabilities across sectors.
Exclusive AI Capabilities Matrix
An explorable, visual map of AI applications across sectors.
Exclusive AI White Paper Library
Every Emerj online AI resource downloadable in one-click
Best Practices and executive guides
Generate AI ROI with frameworks and guides to AI application