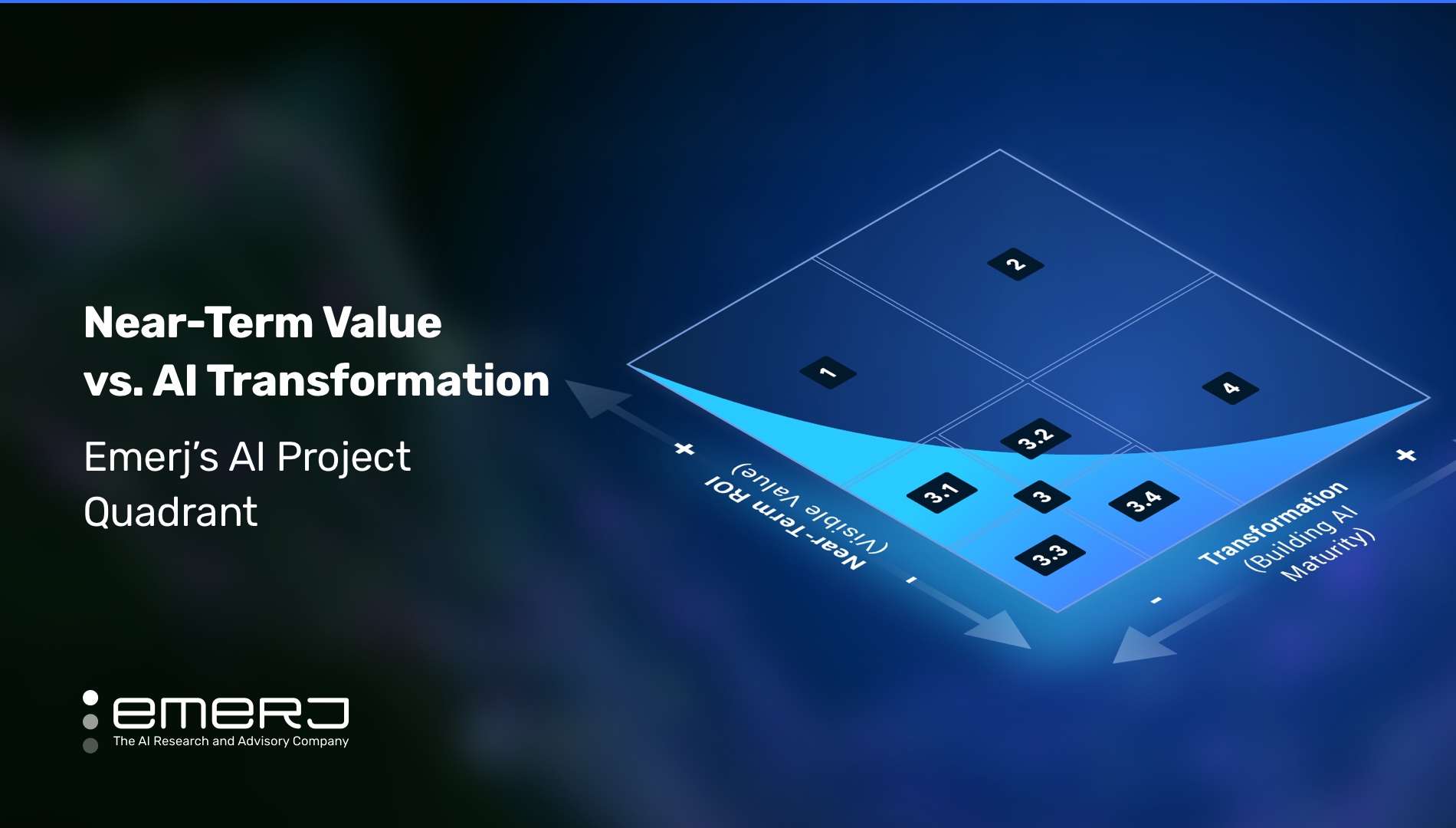
You can invest in AI maturity and future capability – or you can have “quick wins” with surface-level AI applications that have relatively short-term, narrow ROI.
But you can’t have very much of both at once.
For better or worse (often the latter), AI solutions are sold by promising near-term value – which is almost never in line with the kind of core AI maturity development that companies need to actually enable AI broadly.
Today, the elements of AI maturity (i.e. data infrastructure, developing a culture of innovation, developing in-house data science talent, etc) are often framed merely as adoption barriers, as hurdles, or challenges. Done right, however, the lessons learned and maturity built from AI projects become a new layer of capability for the organization writ large – and investment in unlocking future AI value.
We created the Emerj AI Project Quadrant to help leaders maximize near and long-term value, and communicate the delicate balance between the two to their own stakeholders or clients – which is a critical aspect of expectation-setting for AI projects.
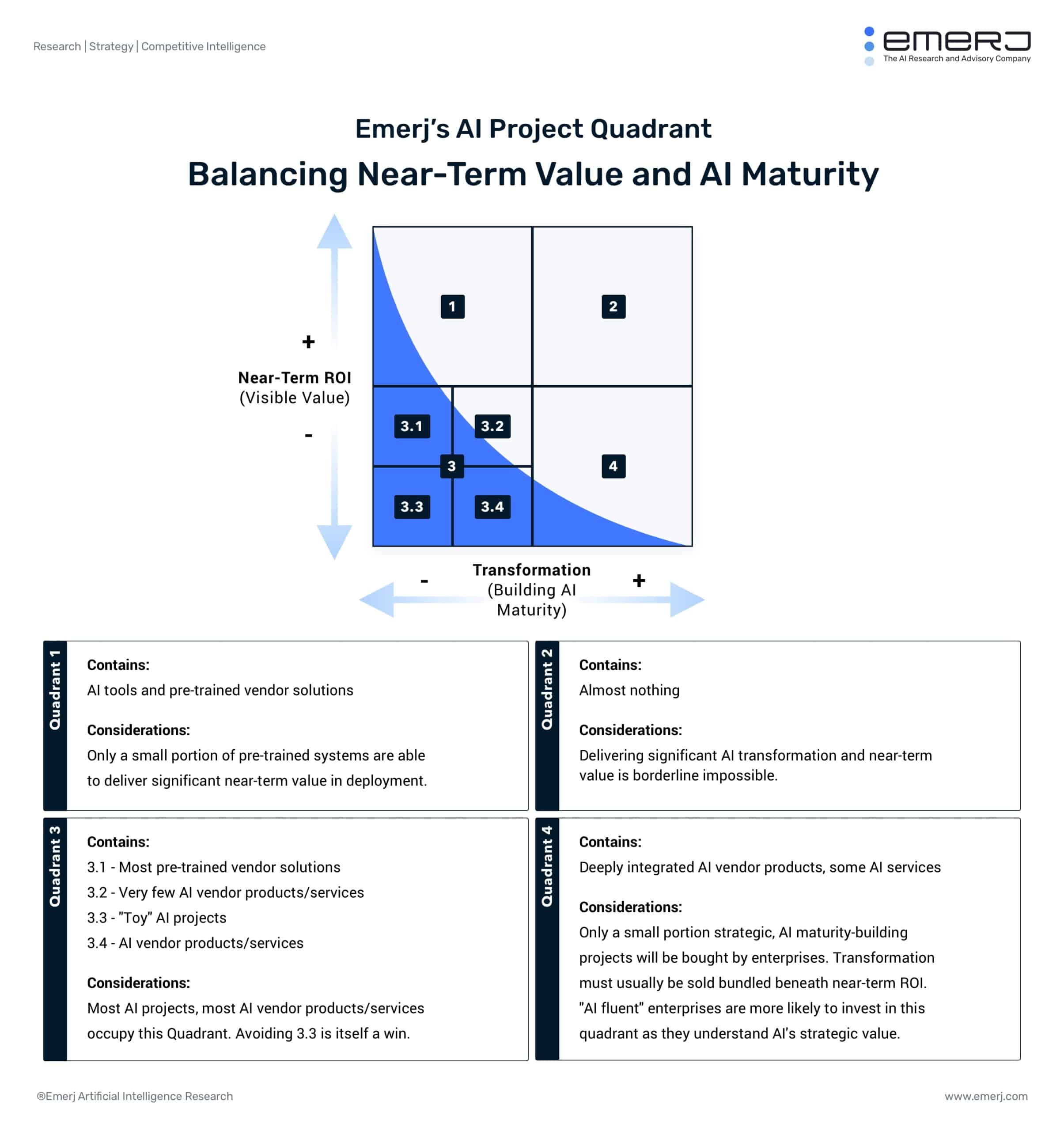
The Emerj AI Project Quadrant – Overview
The Y-axis is the near-term ROI, which is basically the visible value of an AI project. The X-axis is transformation, or building critical capabilities to achieve AI maturity and unlock future AI value.
The blue-shaded area of the chart represents all possible AI projects. Notice that this blue space includes only part of quadrants 1, 3, and 4, and none of quadrant 2. This is because any one AI initiative is unable to generate both (a) massive improvements in AI maturity, and (b) significant near-term ROI. The two are inherently opposed in many respects.
Near-term ROI requires a focus on surface-level issues with relatively immediate AI-based solution. It is often about avoiding transformation and AI maturity.
Transformation requires a focus on building new capabilities (not simply “spinning up” a solution that may become technical debt). It is about moving beyond surface-level applications and setting up a firm for long-term AI advantage.
The four quadrants represent classifications or categories of AI projects that have a specific mix of near-term value and AI maturity. Below, we’ll explore each of the quadrants in depth.
Quadrant 1 – Plug-and-Play
Contains pre-trained (or mostly pre-trained) AI vendor solutions and API-able AI tools.
The vast majority of AI projects are not plug-and-play or IT-like. The vast majority of AI applications involve the use of our own proprietary data, training models off of information unique to our own business. Most of the time, buyers who hear “plug-and-play” should be extremely skeptical.
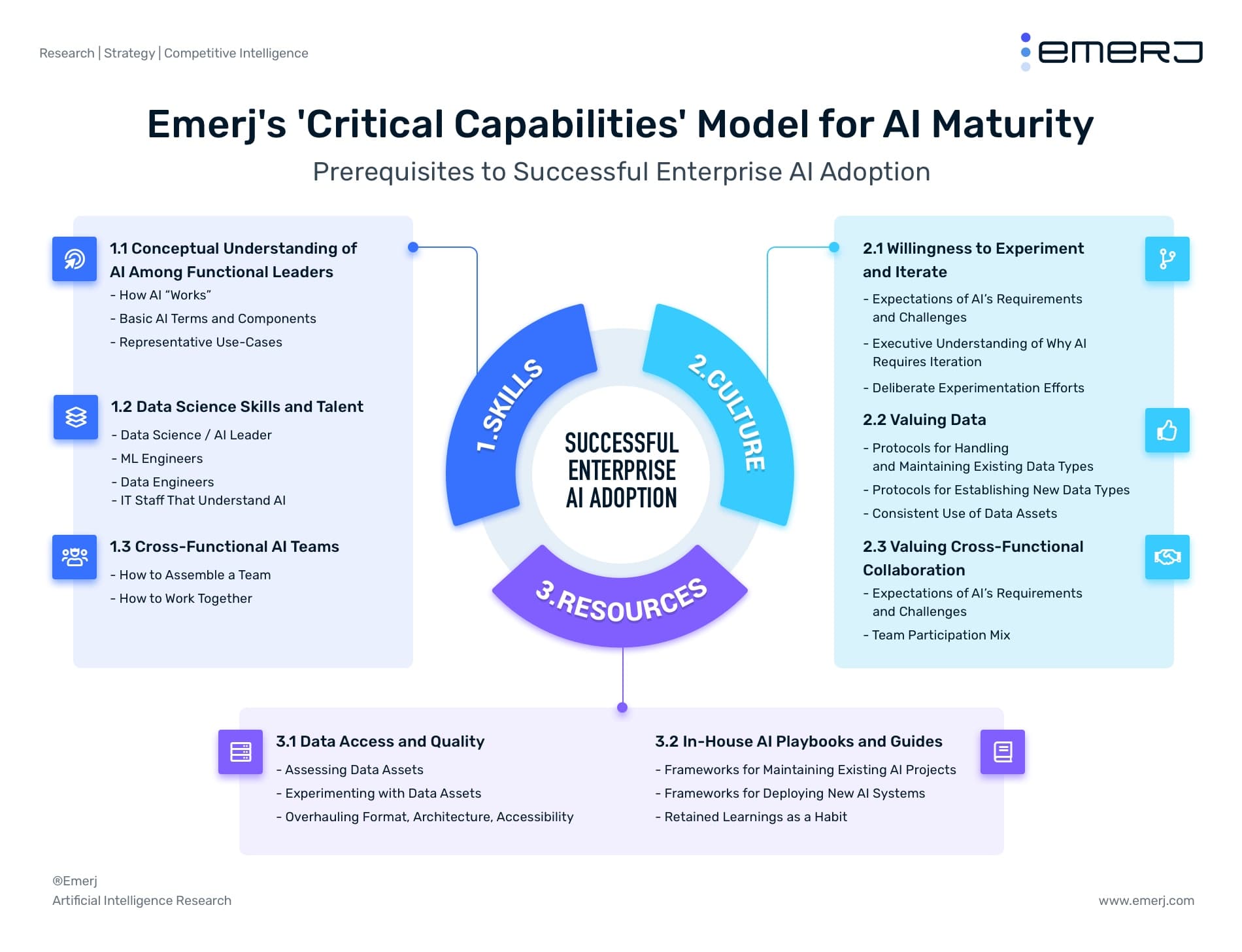
There are, however, classes of problems that work well with minimal training, or nearly no training at all. For example:
- AIDoc.com develops healthcare AI-based decision support software. When someone goes in for a chest X-ray, the company pulls the image up into the cloud and uses a pre-trained computer vision algorithm to detect if there are any anomalies such as cancerous growths. The company then sends back a new image with highlighted areas down into the workflow of the radiologist, who will then interpret the images.
- Amazon’s NLP API Comprehend for scoring sentiment on customer service messaging is also another example. There is no genuine transformation happening with its use, no real development of AI maturity. By simply hooking up the Amazon APIs to the existing data flows, end users can determine the sentiment or entities in a given corpus of text data.
A manufacturing company aiming to use computer vision to detect defects in very specialized metal parts will not be able to use off-the-shelf solutions, but will need to customize a vendor solution by training it on their own data – or build an entire solution from scratch.
Plug-and-play solutions offer a wonderful opportunity to shortcut the “hard parts” of AI adoption – but they offer none of the learning and competency-building benefits of more robust AI deployments. As such, selecting surface-level plug-and-play tools should only be done when (a) the problem suits pre-trained systems, and (b) the problem does not warrant more robust long-term investment in data infrastructure and AI maturity.
One of our AI market research advisors and podcast guests, Nishant Chandra, refers to plug-and-play projects are like throwing another “topping” on top of a technology “pizza.” They simply play at the surface. For transformation to take place, and for firms to genuinely become more AI-enabled, they need to think about baking AI into their tech stack and product stack, more like a “lasagna.”
Nishant’s very popular first episode on our AI in Financial Services Podcast breaks down some key distinctions around surface-level versus transformative AI adoption:
When to Pursue Quadrant 1 Projects
- Your problem is a commodity and doesn’t require the use of your own proprietary data. For example, detecting people or vehicles in your security footage is not a unique challenge to your security cameras, and many vendors have already trained models to solve versions of this problem.
- There is little or no benefit to developing data infrastructure to solve this particular problem (see security camera example, or AIDoc example above).
Quadrant 2 – Snake Oil
Contains almost nothing. Almost no single AI initiative is able to deliver both immediate near-term AI ROI and robust development of AI maturity and transformation.
With the right AI roadmap and AI transformation vision, it is possible for an enterprise to select AI projects that
It is not possible to gain both the immediate value of plug-and-play API tools with the robust transformation benefits that come from deeper AI integrations – the two simply don’t come along in the same package. Quadrant three (below) offers a realistic balance between the two, but quadrant two is essentially impossible.
Most vendor firms won’t even attempt to frame themselves in this second quadrant, because most vendors who exaggerate the ease and benefit of their solutions emphasize only the near-term financial benefits – neglecting the reality of strategic and AI maturity benefits altogether. That said, firms who do promise both the building of AI capabilities and near-immediate financial ROI are safely in the Snake Oil Quadrant and aren’t to be trusted.
It is the job of an AI catalyst – whether an outside AI consultant or an internal project leader – to be honest and frank about the results of a project, and to communicate the dynamics of AI adoption to ensure aligned expectations with stakeholders and budget providers.
When to Pursue Quadrant 2 Projects
- Don’t. Instead, build a strong AI roadmap with both near-term ROI, and long-term capabilities – and structure individual projects with realistic expectations along that trajectory. Avoid ever overselling the varied benefits of any single initiative, and do not expect any immediate, surface-level application to yield long-term transformational value by itself.
Quadrant 3 – Balanced
Contains most projects involving AI vendor platforms and solutions. Typically requires the use of one’s own data, and some level of integration and workflow change.
The bulk of AI initiatives should fit somewhere in the third, or Balanced, quadrant. That means that these projects are unlikely to create an immediate financial return, and will involve the iteration, and R&D approach that is always needed to coax forth new functionality and value from unexplored data.
At the same time, projects in this quadrant are also likely to have the potential upside of creating AI maturity by improving the skills, culture, and resources a firm will need in order to make the most of future AI initiatives. For example:
- A bank working to detect money laundering might leverage a vendor or internally built AI solution to detect patterns of laundering from a variety of sources of information across many banking IT systems. While this is a strong opportunity for AI and there are a variety of seemingly successful use-cases of AI for anti-money laundering (AML), there would still be a reasonable amount of iteration and experimentation in order to detect the fraudulent patterns in the bank’s unique set of data sources – and there is no guarantee that this experimentation will yield results. If executed well, even a pilot AI AML project may lead to new insights about our data silos and features, and new ways for SMEs and data scientists to work together.
- A jewelry eCommerce firm may decide to leverage a recommendation engine to improve their on-site and in-email product exposure in order to win a higher LTV on their existing customer base. This project, again, would involve iteration in order to combine data sources (CRM data, email response data, on-site analytics, etc) to determine the best way to recommend products. Again, no great improvement is guaranteed in this R&D effort, but there is rife opportunity to develop elements of AI maturity.
The Sub-Quadrants of Quadrant 3
The reality is most projects are going to be in one, three, and four quadrants, and actually a majority are going to be in three. Since there are so many AI projects that fit into the Balanced Quadrant, it is necessary to classify them according to varying proportions of near-term value and AI transformation. Below we’ll break down the four sub-quadrants that can be seen in the Emerj AI Project Quadrant graphic above.
- Sub-quadrant 3.1: Pre-trained vendor solutions, or solutions that involve relatively modest data access, algorithm training, and AI maturity requirements. This is essentially similar to quadrant 1.
- Sub-quadrant 3.2: We will see very few AI vendor products/services that can deliver near-term ROI and AI transformation at the same time. The only way to get there is when the solution is a strong, precise fit for the client’s needs and the client has to be willing to invest in capability as part of the process. In other words, the client must have a perspective on the long term, in addition to the near-term value. Projects in 3.2 are “as good as it gets” for AI projects to achieve both kinds of value.
- Sub-quadrant 3.3: AI projects with no to low near-term value and no to low AI transformation, and we call these “toy projects.” Toy AI projects do not deliver near-term value nor build transformation. They are often spurred on by misguided fear of missing out (FOMO), or by novelty alone. This sub-quadrant is obviously to be avoided.
- Sub-quadrant 3.4: We will see projects that require communicating the value of AI maturity as a foundation for future value and deliver value reasonably in the near term. An example of this type of project might involve an AI and data platform like Sagemaker or DataRobot, which is acting more like an involved vendor than a service provider is because it requires transformation through moving data on a new platform, training people to interact with data in new ways, and forging cross-functional teams with the complete support of the client.
The Danger of Down-Playing AI Maturity in the Balanced Quadrant
The most common error of projects that should be in the Balanced Quadrant, is when salespeople or internal champions frame these projects as if they are in the Plug-and-Play Quadrant.
As an external vendor or internal AI project leader, it can be appealing to simply drum up the near-term financial return on investment of an AI project, allowing stakeholders and budget holders to remain ignorant as to the inherent challenges to AI adoption (iteration, data cleaning, team dynamics changes, more). This rush for near-term ROI emphasis can often get a pilot project approved, but it sets up projects to flop in terms of shared understanding with leadership.
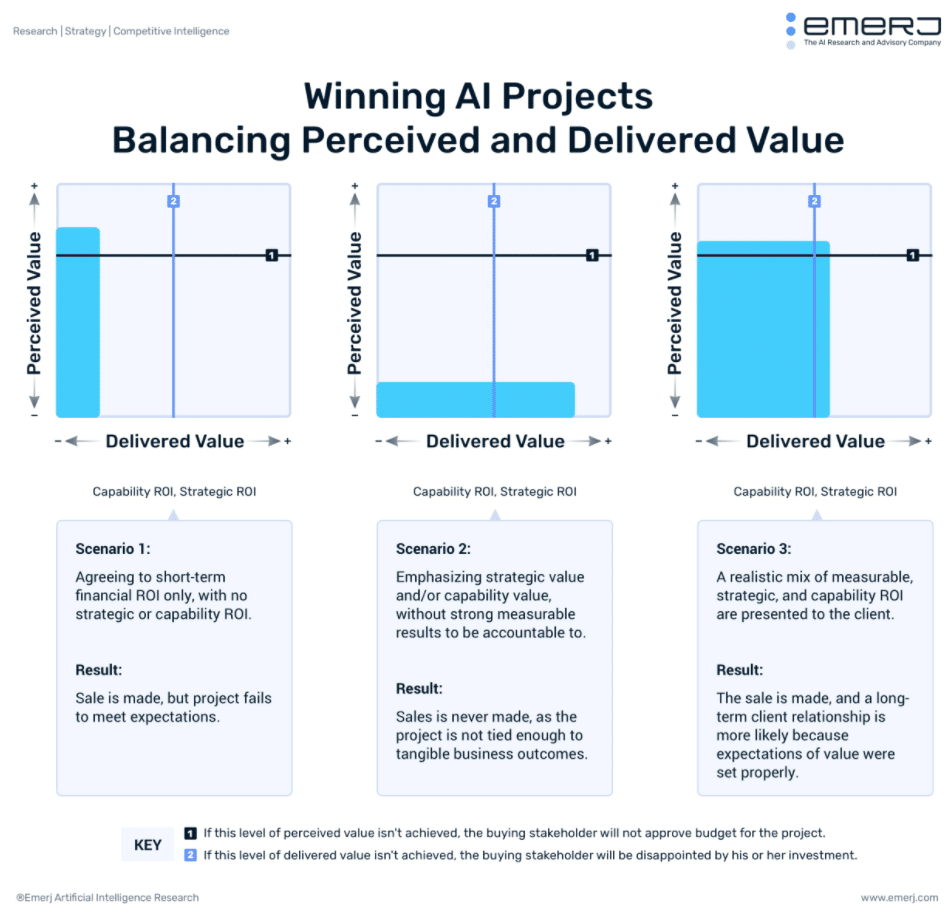
If done correctly, Quadrant 3 (and potentially Quadrant 1) projects can serve as early sources of momentum for more genuine investment on AI maturity – and so further expansions of general AI capability within the organization. Dataiku’s Chief Customer Office Kurt Muehmel refers to these project as “lighthouse projects” – though we’ve heard them referred to by many other names. Kurt’s interview on the AI in Business Podcast below is a good starting point for rolling out an AI adoption plan that accounts for both near and long-term ROI:
When to Pursue Quadrant 3 Projects
- Your problem is a commodity and doesn’t require the use of your own proprietary data. For example, detecting people or vehicles in your security footage is not a unique challenge to your security cameras, and many vendors have already trained models to solve versions of this problem.
- There is little or no benefit to developing data infrastructure to solve this particular problem (see security camera example, or AIDoc example above).
Quadrant 4 – New Foundations
Contains robust efforts to overhaul core elements of AI maturity, including data infrastructure or department/team structures.
We call quadrant four the New Foundations Quadrant because a basic requirement is communicating the value of AI maturity as the foundation for future value. These include deeply integrated AI vendor products and services that are longer-term roadmaps and transformation projects to build maturity and deliver on projects and are relatively low in near-term ROI. Often, these projects serve merely as foundations (hence the name) for other, more targeted near-term AI projects to build off of.
At present, projects in this quadrant are rare in the enterprise. First – because the general understanding of AI as a paradigm shift (what we call the executive AI fluency) of the firms is low, and so maturity is undervalued. Second – because most leadership is interested in seeing some kind of value from AI from its early projects before more robust and abstract investments in maturity are made.
For the first few years of an enterprise’s AI adoption efforts, the bulk of AI maturity is likely to be built through projects in Quadrant Three. Overly aggressive investments in “maturity” without a strong understanding of a firm’s AI roadmap or transformation vision can lead to substantial technical debt.
Examples of initiatives in this quadrant might involve:
- An overhaul of customer and marketing analytics data at a brick-and-mortal retail company, in order to match customer IDs and purchase behavior between online and offline purchases. This might involve efforts to identify known online users to in-store purchases – but would also likely involve significant overhauls to data storage, data features, and potential iteration to determine the best data formats to enable various AI use-cases. This new level of AI maturity could serve as a jumping-off point for many future projects.
When to Pursue Quadrant 4 Projects
- You have developed a strong AI roadmap with clear use-cases for near-term AI capabilities and ROI – and need to support that roadmap with robust investment in data infrastructure, team training, or other elements of AI maturity.