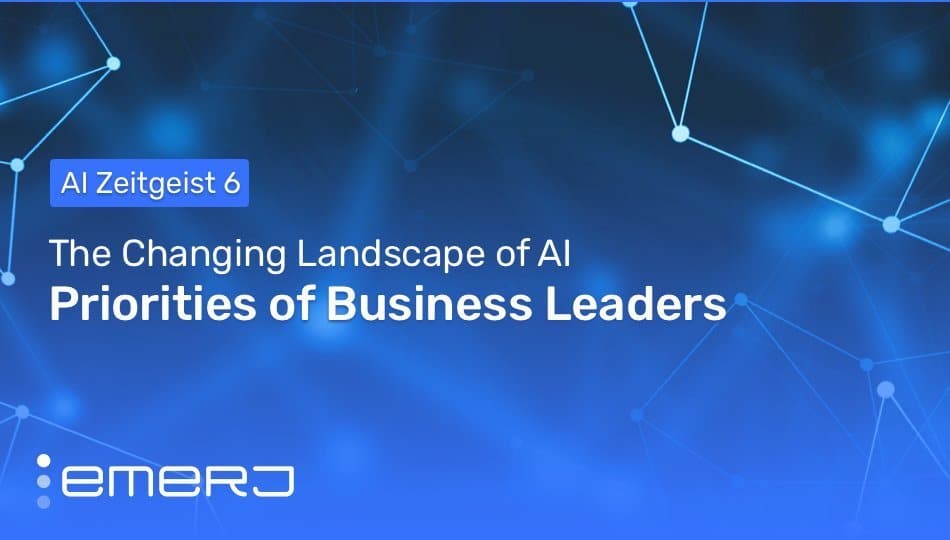
We come to the sixth installment in the "AI Zeitgeist" series, and this one is about the changing landscape of artificial intelligence priorities for business leaders.
We've covered all sorts of topics in the series, thus far. We've talked about the buying readiness of business leaders. We've talked about the AI talent landscape. We've talked about how people speak about artificial intelligence in the future, and how it won't always be a buzzword.
But what does all this mean for business leaders, practically speaking, as we go through these three phases of "Emergence," of "Adoption," of "Dispersion?"
How are the mindsets of leaders going to change around AI? How are their priorities going to alter as their industry and their business go through these three phases?
(Note: If you haven’t read the first article in the AI Zeitgeist series, you should familiarize yourself with it first. In that article, I explain what the 3 phases of “Emergence,” “Adoption,” and “Dispersion” actually mean)....
You've landed on exclusive content for Emerj Plus Members
Emerj Plus Membership
In-Depth Analysis
Consistent coverage of emerging AI capabilities across sectors.
Exclusive AI Capabilities Matrix
An explorable, visual map of AI applications across sectors.
Exclusive AI White Paper Library
Every Emerj online AI resource downloadable in one-click
Best Practices and executive guides
Generate AI ROI with frameworks and guides to AI application