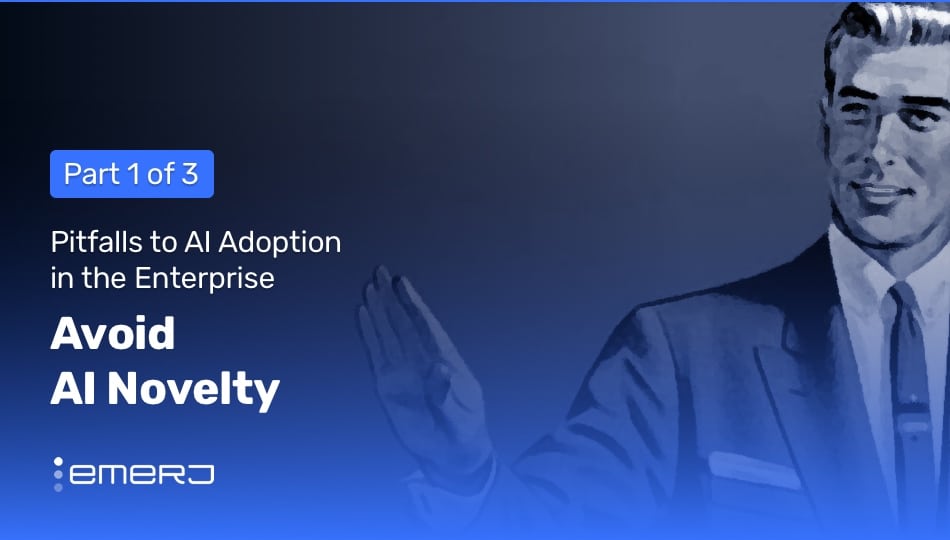
What's harder than training an algorithm to detect images or automate a process? Collecting and cleaning the data in the first place.
And what's even harder than that? Integrating AI systems into old technology, old processes, and old skillsets that exist in most enterprises and midsize businesses today.
This sort of cultural shift is arguably vastly more challenging than the technical problems of AI. Computer vision and different kinds of NLP are rather proven use cases. They provide great value when you have the right data and the right setup, but baking that into an existing business is where the challenges arise.
This is what the vendor landscape of AI companies are trying to solve. They're all trying to become more accessible.
Because of this accessibility issue, enterprises often jump the gun and make poor decisions, and there are factors that they simply don't consider or factors that they often over consider. We talked to vendors, we talked to consultants and we tal...
You've landed on exclusive content for Emerj Plus Members
Emerj Plus Membership
In-Depth Analysis
Consistent coverage of emerging AI capabilities across sectors.
Exclusive AI Capabilities Matrix
An explorable, visual map of AI applications across sectors.
Exclusive AI White Paper Library
Every Emerj online AI resource downloadable in one-click
Best Practices and executive guides
Generate AI ROI with frameworks and guides to AI application