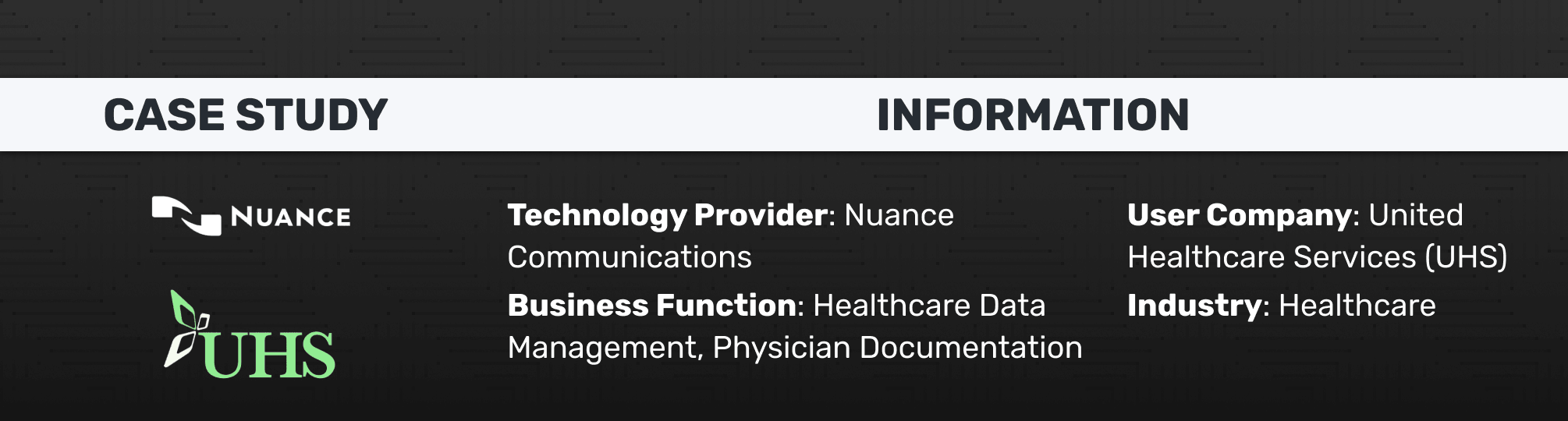
Technology Provider: Nuance Communications, an AI technology company that provides products in voice, natural language understanding, reasoning and systems integration.
User Company: Swedbank, a bank based in Sweden with branches in the U.S.
Industry: Banking
Application: Customer Service
Problem
Swedbank wanted its customer contact center agents to focus on sales rather than on service. The bank’s 700 contact center agents were handling 3.6 million annual customer interactions, which included more than two million basic transactional queries, 500,000 emails, and 10,000 social media interactions. The bank wanted to automate this process to achieve its goal.
According to Nuance, 60% of Swedbank’s customer base is already digital. The bank also reportedly estimated that, by 2018, digital channels, such as the website help chat bot, emails, and social media, will be its customers’ primary choice for contacting Swedbank. Therefore, the bank wanted to ensure that all customer queries could be handled (as much as possible) via swift, self-service options on said digital channels.
Actions Taken
Swedbank turned to Nuance’s conversational AI virtual assistant, Nina. The integration of Nina with Swedbank’s contact centers allowed the bank’s customers to search for information on Swedbank’s homepage search interface and get answers to basic transactional questions.
Nina answers the customers’ freeform questions in a conversational tone. For example, if a customer wants to order foreign currency, Nina either redirects the customer to a relevant web page or branch office, or asks clarifying questions such as which country’s currency the customer wants to order.
Apart from being the customers’ first point of contact, Nina also reportedly helps the bank’s contact center agents with quick information searches for answering customer queries.
Results
Nuance claims that, within three months of deployment, Nina handled over 30,000 conversations per month with a 78% “first-contact resolution.” Nuance claims that about 55% of these conversations did not require the customers to take further actions, such as calling the contact centers. According to Nuance, Nina can now handle 350 different and discreet customer questions and answers.
Transferable Lessons
Swedbank’s application of NLP for customer service mirrors the efforts made by many large firms. Namely, firms with a certain high volume of inbound inquiries are able to congeal their most common questions into a reliable set of query types – training a machine learning system on the various permutations of those individual demands. Company with millions of customers (like Swedbank) are able to train on huge volumes of data, giving them a strong ability to find all the permutations of individual queries.
For example, there might be hundreds of ways that customers might ask to see their bank balance (“What’s the balance in my 445668700 account today?”, “How much do I have?”, “What’s left in my savings account?”, etc), and these queries might be made in multiple languages through a dozen different channels (chat, phone, online FAQ, etc). The low-hanging fruit for companies today is to have machines that can handle simple and common requests, and accurately pass along more complex requests to humans who can handle the matter accurately.
Given the current state of machine learning, AI systems are often unable to answer uncommon questions, or to handle complex customer requests. Fundamental improvements in machine learning technologies may be required to cover this gap, and it’s safe to say that it’ll be years (as of this writing, February 2018) before customer service machine (whether via chat or voice) are able to replace human customer service workers while still maintaining service quality.
Fortunately, handling simple requests and routing complex requests is still valuable for enterprises with huge customer service costs, and there is hope that these systems will gradually become more capable over time.