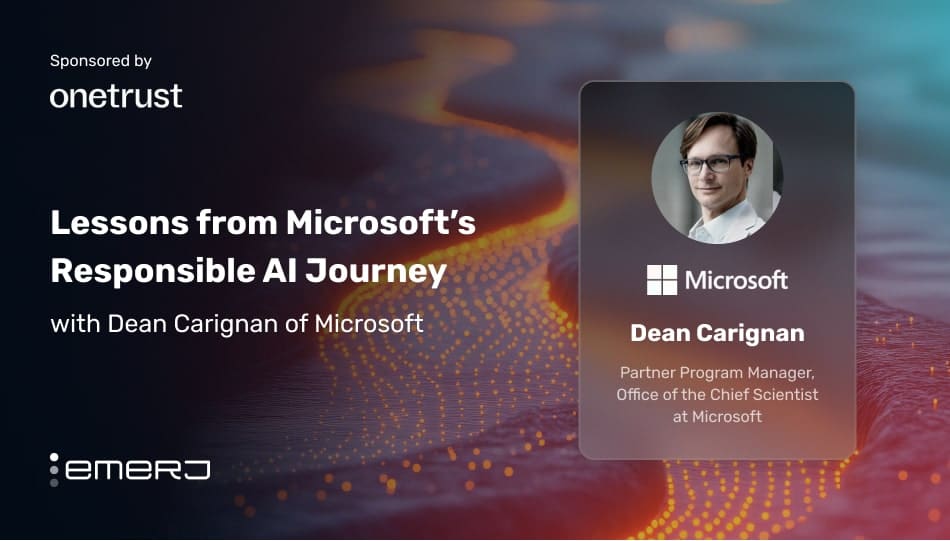
This interview analysis is sponsored by OneTrust and was written, edited, and published in alignment with our Emerj sponsored content guidelines. Learn more about our thought leadership and content creation services on our Emerj Media Services page.
The rapid integration of AI into various sectors has raised significant concerns regarding trust and ethical standards. In a blog authored by Kirk Stewart, Founder and CEO of KTStewart, the USC Annenberg School for Communication and Journalism explains that without proper attention to ethical and regulatory risks, AI systems can perpetuate biases, leading to unfair outcomes in critical areas like hiring, lending, and law enforcement.
For instance, AI algorithms may inadvertently discriminate against certain groups, resulting in unjust decisions that undermine public confidence.
A notable example of AI system failure due to trust issues is the discontinuation of IBM’s Watson for Oncology. According to a succinct post on Harvard University’s blog authored by numerous business leaders, including Jeffrey Saviano, Business AI Ethics Initiative Leader at Ernst & Young, what was initially hailed as a revolutionary tool for personalized cancer treatment faced setbacks due to inaccuracies and unsafe treatment recommendations.
Failures like that of Watson for Oncology underscore the critical importance of data quality and diversity in AI-driven healthcare solutions.
Recent research from the Centre for Computing and Social Responsibility at De Montfort University emphasizes the importance of integrating responsibility into AI systems to ensure they operate ethically and align with societal values. It proposes characteristics that an ecosystem must fulfill to be considered responsible, highlighting the need for AI to be developed and deployed with ethical considerations at the forefront.
Emerj Managing Editor Matthew DeMello recently sat with Dean Carignan, Partner Program Manager, Office of the Chief Scientist at Microsoft, to discuss Microsoft’s approach to responsible AI, including key principles, risk management strategies, and the integration of ethics into AI development.
This article examines two critical insights on responsible AI adoption strategies from their conversation:
- Adopting agile risk management to ensure AI safety and growth: Adopting a continuous risk management approach where research, policy development, and engineering work in tandem to identify new risks and swiftly update policies and tools.
- Leveraging responsible AI as a key product feature: Position responsible AI as a core feature of product lines to improve quality, reliability, and user trust, driving faster adoption, customer loyalty, and differentiation in a competitive market.
Listen to the full episode below:
Guest: Dean Carignan, Partner Program Manager, Office of the Chief Scientist, Microsoft
Expertise: Innovation management, Responsible AI, Research
Brief Recognition: Dean Carignan is the Partner Program Manager at Microsoft’s Office of the Chief Scientist, where he leads all program management efforts for the newly established organization focused on advancing scientific thinking both within Microsoft and society. Dean has been with Microsoft since 2004 and holds an MBA in Strategic Management from INSEAD.
Adopting Agile Risk Management to Ensure AI Safety and Growth
Dean opens the podcast by outlining Microsoft’s approach to Responsible AI, listing the 6 Key Principles of Responsible AI at Microsoft as outlined in the company’s new book:
- Fairness: AI systems should treat people equally and without bias.
- Reliability and Safety: Systems should be robust, avoid failures, and be easy to fix if issues arise.
- Inclusiveness: AI should work well for everyone, addressing diverse needs.
- Privacy and Security: Systems must adhere to stringent privacy and security standards.
- Accountability: Systems should allow for precise tracing of issues to address problems and assign responsibility.
- Transparency: Users should understand how AI systems are built, maintained, and effectively used.
Dean highlights Microsoft’s early commitment to Responsible AI, recognizing in 2016 that AI was moving from research into real-world applications. To address potential risks, the company founded Ether—AI Ethics and Effects in Research and Engineering—bringing together researchers, engineers, and policy experts to anticipate challenges and develop solutions.
This initiative became the foundation for a comprehensive, responsible AI framework, now supported by over 350 dedicated professionals, including more than 100 focused exclusively on ethical AI practices.
He believes the rapid pace of AI advancements presents unique challenges, as traditional risk management systems are not designed to adapt to new risk categories emerging every two to three months.
To address these challenges, Microsoft employs a triad of research, internal policy development, and engineering to create a “flywheel effect” that identifies future risks, updates policies, and builds tools to make AI systems safer and more reliable.
Dean emphasizes the importance of guiding principles as a “North Star” in the rapidly evolving AI landscape. He compares these principles to a constitution, providing clarity on what a company stands for, the boundaries it will not cross, and how it navigates day-to-day decision-making. With AI models advancing at an unprecedented pace, often monthly, such principles are essential for maintaining consistency and ensuring decisions align with the organization’s core values.
Leveraging Responsible AI as a Key Product Feature
Dean discusses red teaming as a critical approach to stress-test AI systems, ensuring they’re robust and safe before user exposure. Borrowed from Cold War-era military simulations, red teaming involves a team trained to identify vulnerabilities by simulating potential failures and misuse cases.
At Microsoft, this was incubated within Dean’s team and later integrated into the security organization. Effective red teaming requires a mix of machine learning expertise, user empathy, and engineering skills to explore edge cases AI systems might face at scale.
He also emphasizes adaptive policy-making as an agile process rather than a traditional waterfall approach. Policies are continuously updated with each new model to address emerging risks, forming a feedback loop: red teaming identifies harms, policies are revised, and engineering systems evolve to mitigate those risks.
Dean likens this to treating policy as dynamic code, with change logs and ongoing iterations reflecting technological advancements.
Lastly, Dean underscores the cultural shift towards viewing responsible AI as a feature, not merely a compliance obligation.
“The second really important thing we write about it in the book quite a bit, is we call it ‘feature-izing’ responsible AI. What this means is creating a culture in which people think of responsibility as a feature of the AI system, something that makes it better, as opposed to a compliance hoop that you have to jump through. And this is a journey we’re still on.
We’ve got a lot more progress that we can make, but we found that when we present responsible AI as something that customers want, that makes customers more willing to use our AI, allows them to adopt it faster, the mindset that it’s just a feature like quality, speed, and reliability that really resonates with people.”
– Dean Carignan, Partner Program Manager, Office of the Chief Scientist, Microsoft
For Emerj’s audience, Dean lists key steps for advancing responsible AI within an organization:
1. Define Core Principles: Start by identifying and documenting what the company stands for regarding responsible AI. Leverage existing corporate values to avoid starting from scratch and ensure these principles are widely communicated across the organization.
2. Assess and Plan with a Maturity Model: Use tools like Microsoft’s Responsible AI Maturity Model to evaluate your current practices and identify areas for growth. This model categorizes organizations into five phases of maturity, offering clear steps to advance capabilities.
3. Leverage External and Internal Resources: Recognize that responsible AI is a growing field with extensive resources available from companies, academia, governments, and nonprofits. Assign someone within your organization to study these resources and tailor them to your company’s needs.
4. Build a Passionate Team: Tap into employee enthusiasm for AI as a force for good. Microsoft initially relied on volunteer efforts and champions before formalizing roles in responsible AI in 2019. Identifying and empowering passionate individuals can catalyze momentum, enabling them to drive awareness, learning, and implementation efforts.