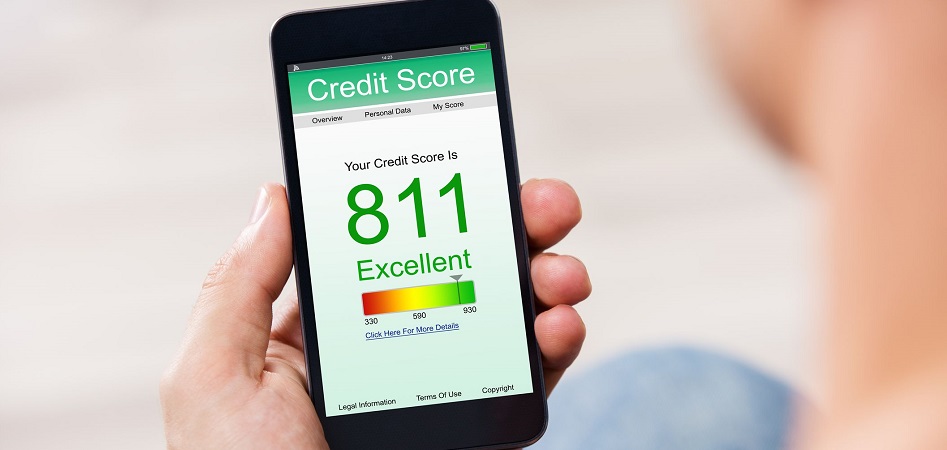
The advent of machine learning in finance ushered in a keen interest in using AI to automate processes from fraud detection to customer service. While some use-cases aren’t nearly as established as others, our research leads us to believe that in the coming five years, banks will continue to invest in machine learning for risk-related processes, including underwriting.
In an interview on the AI in Industry podcast, we spoke to Jay Budzik, CTO at ZestFinance, about the ways in which underwriters can use machine learning-based credit models to win more business and reduce risk by taking advantage of new sources of data that are now digitally available and ripe for feeding into a machine learning model.
These models are challenging traditional credit scoring techniques, including FICO scores and simple scorecards. In this article, we discuss the ways in which machine learning can expand a lender’s customer base to cover the so-called “credit invisible” (people with thin or no credit histories) and those whose credit scores are not accurate reflections of their risk.
We start with new sources of data: How FICO and traditional credit scores are too narrow in scope to serve key demographics that are often locked out of credit accounts altogether as a result.
Traditional Credit Score Variables Vs. New Data Sources
FICO Scores: An Overview
Over the last thirty years, the FICO score and similar credit scores have established themselves as the standard in credit modeling. FICO has allowed banks, credit card companies, and other lenders to objectively assess the creditworthiness of credit applicants. The score is calculated based on five factors, each of which is made up of several variables with varying weights and each of which makes up a percentage of the overall FICO score:
- Credit History (35%): One’s credit history is made up of the existence of blemishes and positive accounts on one’s credit report. These blemishes include late payments, bankruptcies, foreclosures, and similar instances which represent a person’s inability to pay their debt.
- Credit Utilization (30%): FICO scores factor in how much of a credit limit one uses in a given billing cycle, how many credit accounts one has open, and how much one’s down payment is on installment loans, among other variables.
- Length of Credit History (15%): The longer one holds open credit accounts (as long as they use them), the better their FICO score.
- Credit Types (10%): One’s FICO score is affected by how varied their lines of credit are. Types of credit include mortgages, auto loans, and credit cards.
- Recency (10%): FICO scores factor in how recently one applied for credit, paid off an account, or increased their balance, among other variables.
The Credit Invisible and The Catch-22 of Credit History
What all of these factors have in common is the necessity of previously acquired lines of credit. As a result, traditional credit scores are often barriers to entry for the “credit invisible.” According to the Consumer Financial Protection Bureau (CFPB), there were 26 million credit invisible Americans in 2015, nearly one in ten Americans. In addition, the CFPB found that “consumers in low-income neighborhoods are more likely to have no credit history or not enough current credit history to produce a credit score.”
These segments of the population are the most likely to need loans for big purchases, but their lack of credit history prevents them from getting approved for loans and credit lines when underwriters use traditional credit scores to assess them: it’s a catch-22.
There are also borrowers with credit scores that don’t accurately reflect the risk they pose to lenders. Experian found that millennials on average have credit scores around 638, less than the US national average and much less than previous generations. The company admits that this is partly due to the age of these borrowers; their credit histories are thin, and credit history makes up 30% of their FICO scores. As a result, lenders may not approve them for loans because their scores are too low when they may actually not pose that much risk; they’re just young.
While FICO and traditional credit scores proved useful for older generations of middle-class Americans, these scores may be less useful for millennials and low-income Americans that are used to making purchases with debit cards. These credit invisible borrowers are not necessarily risky, but lenders rarely approve them because without a credit score their risk is unclear.
The Challenge of “Change Over Time”
According to the company, FICO scores don’t change much over time. ZestFinance believes this can make it difficult for FICO scores to differentiate between the following two people:
- Someone with a few late payments from five years ago on their credit report but who hasn’t made a late payment since
- Someone who never had a late payment on their credit report until the last few months, during which they missed several payments in a row
FICO and traditional credit models may have trouble accounting for how the lives of these two borrowers changed over time and affected their ability to pay their debts. This might prove troublesome for young people in particular, many of whom are struggling with debt.
Experian reported on an Opploans survey that found that roughly one in four millennials feel they weren’t properly educated on how to build good credit. The same survey found 15% of millennials regularly miss credit card payments.
They may find their financial footing later in life, allowing them to easily make payments on time, but traditional credit scores aren’t going to reflect this immediately. These borrowers may struggle to get approved for a loan because of the poor credit history they built when they were younger, and the inability to open credit accounts will keep their scores low. Once again, it’s a catch-22.
New data sources may be the solution.
New Data Sources for Credit Scoring
Whereas a FICO score may incorporate a dozen or two variables into its score, according to Budzik:
The models we put into production for our customers tend to have hundreds or thousands of variables in them. We have one with 2200 variables that’s running an auto lending business.
More data means more nuanced credit models, and these models can give an underwriter a much more accurate picture of whether or not a loan applicant is a risk. New data sources might include:
- Public records of pending court cases
- The make and model of a car that an auto loan applicant is looking to buy
- Satellite images of a property for which a borrower is looking to take out a mortgage
- The kinds of products the borrower purchases on their credit card
These categories of data would in some way inform a loan applicant’s creditworthiness, but traditional credit models don’t take any of them into account.
The Advantage of Machine Learning
According to Budzik:
In order to be able to consider more variables, [lenders] need new algorithms that are able to handle them. Machine learning offers a way through that problem. ML can consider all those variables but not make mistakes. Traditional scoring techniques would get tripped up by things like correlations and limitations of the math.
With machine learning, the number of data sources that can factor into a credit model are theoretically infinite. There exist countless variables that might predict an applicant’s ability to pay back their loan, and machine learning is good at finding patterns within large data sets. ML-based credit models could factor in data points that are as of yet unknown to predict a borrower’s likelihood of paying back their loan.
For example, Zest worked with Discover to tap the credit card company’s trove of consumer spending data to build a new model for its $7.5 billion personal loans business. Zest claims the model assessed hundreds of applicant data points, up to 10 times more than Discover’s credit model had used before.
The modelers purportedly discovered that a history of discount-store shopping boosted an applicant’s chances of getting a personal loan, while an applicant writing the full legal name of an employer on a loan application would lower it.
Applicants who called Discover from a landline or cellphone, rather than Skype or other internet-phone services, were considered safer bets because they’re easier to trace back to an individual.
Furthermore, combinations of these sources themselves create their own data points. For example, the fact that a loan applicant purchases accessories for their car on occasion might not affect their ability to pay back their auto loan on its own.
But this combined with the make of the car for which the applicant wants to take out a loan might indicate a lower or higher likelihood the applicant will pay that loan back. These kinds of relationships are nearly impossible for underwriters to figure out, but they’re in large part the value of machine learning.
In addition, machine learning may be much more adaptable than traditional credit models. Developing a new credit model can take a year or more, which can hinder a bank’s ability to keep up with the changing economic landscape.
Customers and markets can change relatively quickly. Some machine learning software for credit underwriting come with automated risk management, which could allow lenders to refit models in under a month so it can adapt its underwriting as the economy evolves.
What ML-Based Credit Models Mean for Lenders
Machine learning could allow banks and other lenders to increase revenue by approving more credit invisible applicants and more applicants whose credit scores paint an incomplete picture of their creditworthiness. ZestFinance, for example, claims to have helped Prestige Financial Services increase loan approvals by 14% with an ML-based credit model.
At the same time, lenders may be able to increase revenue without also increasing risk. Underwriters can start rejecting loan applicants that are riskier than their credit scores imply. As a result, lenders can reduce the losses they incur from these borrowers.
Machine learning may also enable more accurate risk-based pricing. As previously discussed, ML-based credit models can factor in much more data than traditional models, allowing for a more nuanced picture of the applicant’s ability to pay. As a result, lenders can be much more granular with the interest rates they offer borrowers.
ML can pick up minute differences between two very similar borrowers, and these differences may be worth capitalizing on by offering one borrower a higher interest rate. This could increase the profit margin on each borrower without adding to an underwriter’s time scrutinizing a borrower’s application. As a result, at scale, lenders could see a significant boost in revenue.
What It Means for Consumers
Machine learning models that factor in new data sources can assess credit invisible applicants in a way traditional models that focus squarely on credit history cannot. As a result of machine learning-based credit models, applicants may find that lenders are approving them when they wouldn’t have before. Young people with thin credit histories may be able to start building their credit because lenders can start onboarding them.
Similarly, in the future, millennials might find that the credit blunders of their past don’t bar them from obtaining a loan for big purchases in the future when they’re more able to pay back their loans.
In addition, Budzik points out:
Instead of approving people that are going to default…creating a mess by offering credit to folks who aren’t going to be able to pay, lenders can avoid that and prevent that from happening to consumers
As previously discussed, loan applicants with good credit scores may pose more of a risk than their score reflects. An applicant with a score around 700 and in trouble with the law may be forced to pay a fine in installments over the course of a year. Such a judgment could impact the applicant’s ability to pay back their loan, affecting their credit score and causing them even more long-term damage to their future.
A machine learning-based credit model that factors in pending court cases might suggest an underwriter not approve the applicant altogether, even if their credit score would indicate that their worthy of a loan. Lenders can in essence hedge against riskier consumers defaulting on their loans by not approving them in the first place.
This article was sponsored by ZestFinance and was written, edited and published in alignment with our transparent Emerj sponsored content guidelines. Learn more about reaching our AI-focused executive audience on our Emerj advertising page.
Header Image Credit: The Balance