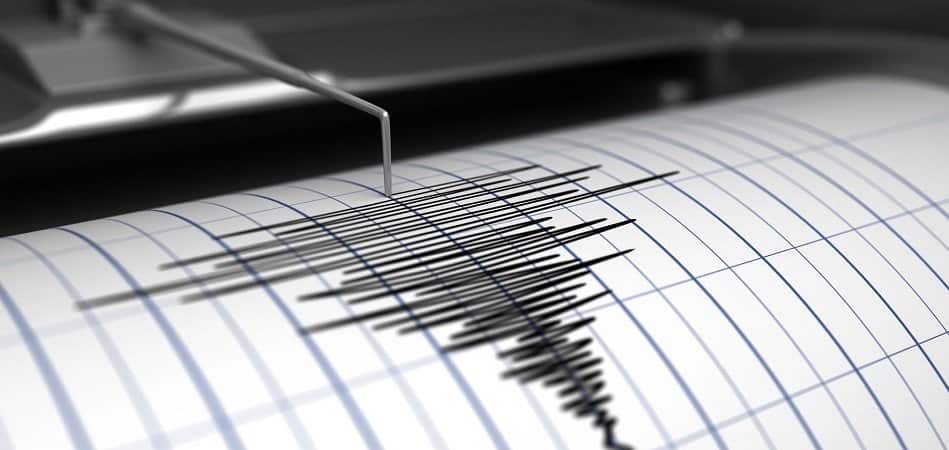
Oil and gas companies face many of the same challenges as large banks and established insurance firms when it comes to searching through their backlogs of documents. They want to use the data stored within these documents to make decisions on where to drill and determine whether or not they’re in compliance with laws and regulations.
The difficulty comes in that many of these documents are stored in physical formats: paper, physical maps, tapes. Oil and gas companies are very used to working with these physical formats, and this poses some challenges when it comes to integrating modern technology into workflows.
Predictive analytics applications and similar AI solutions require large volumes of organized digital data in order to generate insights and drive value for oil and gas companies.
For example, there are a number of AI vendors offering predictive maintenance applications to oil and gas companies specifically. These applications promise to alert personnel working on oil rigs and oil refineries when a machine or piece of equipment is in need of repair.
In doing so, repair crews can fix the machine or equipment before it breaks down and results in a significant drop in production or a serious injury that could cost the oil and gas company millions in legal fees and fines, as well as a sharp hit to their reputation.
Oil and gas companies will spend a lot more time on the initial setup of predictive maintenance applications if they’re storing notes on machine and equipment damage, downtimes, and repair frequency in the form of physical notes that maintenance crews might keep in their desks on site.
Digitizing Paper Documents at Oil and Gas Companies
In order to overcome this challenge and prepare for AI applications in the coming years, Oil and gas companies may benefit from AI-based document digitization software. Document digitization software most often involves machine vision, an AI approach that allows computers to “understand” what’s in a digital image or video.
As such, employees at an oil and gas company would be able to scan their physical documents and notes and upload them as PDFs or JPGs. The document digitization software could then transcribe the letters within those files into typed text that populates a word processor or digital form.
Once the documents are digitized, there are ways for oil and gas companies to extract information and insights from them with solutions that are much less resource-intensive to integrate than predictive maintenance applications.
Iron Mountain is one AI vendor that offers oil and gas companies document digitization and information extraction software, the latter of which runs on natural language processing (NLP) algorithms.
We spoke with Anke Conzelmann, Director of Product Management at Iron Mountain, about where AI-based information extraction and document search could be useful in the oil and gas industry. In this article, we discuss several use-cases for AI-based document digitization and information extraction in oil and gas, such as oil location and contract management.
For more on AI for information extraction in oil and gas, download Iron Mountain’s white paper on the topic.
Information Extraction for Finding Oil
According to Conzelmann:
Geoscientists are looking for geolocation data so they can access it by location. [Using AI], they can point to a spot on the map and get all the assets relevant to that location with a click, regardless of the type or format the information is in
Geoscientists at oil and gas companies spend a lot of time sifting through past drill and well log data and seismic data to discern where they might find more oil and the structures of the rocks in which it might be located. This work is time-consuming and involves a variety of geoscience specialists whose time is limited.
In many cases, these geoscientists are left to assume that they have all the information and data they need to make a decision about where to perform seismic testing or where to drill.
They’ll search through an often unorganized mix of physical and digital documents stored in different formats and in different locations.
An oil and gas company that can fully digitize this data and turn it into a digital, searchable database could not only save hundreds of thousands on each research session but also drive real revenue by discovering new oil fields faster.
A search function based on natural language processing and machine vision could make this possible. After the documents are digitized and organized in the digital database, AI-based Information extraction software could help geoscientists find new locations to drill based on past geolocation data the oil and gas company can access.
Natural Language Processing
For example, geoscientists may want to quickly review all of the well data from a specific location in order to determine if there are areas of similar geology nearby that might contain more oil. Right now, geoscientists need to search for individual documents, both physical and digital, and sometimes organize them based on location themselves. They don’t know if they’ve collected all of the documents that the company has on that specific well.
In addition, they may need to collect public data on the location that is stored in various locations across the web and public databases. With an NLP-based information extraction software, geoscientists could search within the database and across public databases for all of the documents and/or information relevant to a particular geolocation.
Depending on the breadth of integration, the software may be able to search for more granular information, specific types of data within those documents or data from a specific time period.
Theoretically, a geoscientist would be able to type in an iteration of “Well performance data from wells within ‘X’ region and around similar geological structures as ‘Y’ well” into the search function, and the software would pull up the desired data.
NLP Adoption Challenges in the Oil and Gas Industry
That said, building a natural language processing algorithm for the oil and gas industry comes with challenges, mostly due to the fact that the industry uses a lot of specific jargon not found outside of it. As such, NLP algorithms for use in the industry need to be trained to “understand” these words and phrases if specialized employees at oil and gas companies intend to use them for search.
Oil and gas companies would either need to make sure their subject-matter experts can speak what we call the “language of data science” so that they can convey to in-house data scientists what a useful NLP algorithm might look like in their industry.
Alternatively, the company could work with an AI vendor provided that vendor has robust experience working in the oil and gas industry. If they do, their own data scientists will likely be able to “talk shop” with the company’s subject-matter experts. They could then collaborate and build a useful NLP algorithm that allows company employees to search for information with the words and phrases they regularly use at work.
In some cases, AI vendors offering information extraction software to the oil and gas industry will come “pre-trained.” In other words, the vendor’s data scientists may only need to tweak the algorithm slightly in response to a little feedback from the client company’s subject-matter experts before the algorithm is ready to launch.
Machine Vision
Machine vision could also help geoscientists speed up the time they take to collect the information they want to analyze and ultimately the time they take to decide where to drill. The oil and gas company would of course want to their geoscientists to find as many rich petroleum reservoirs as they can before their competitors, and machine vision could allow them to select oil-rich areas for drilling more reliably than how they did traditionally.
Geoscientists may come across an image of a geological intrusion or a seismograph that in the past had lead to a rich oil reservoir underneath the surface.
An AI-based search function with machine vision capabilities could allow geoscientists to search for images similar to the ones they found that indicated rich oil reservoirs. As a result, they could discover new drilling locations that they had previously overlooked or that the company owns but have yet to explore.
Seismic Tapes
Oil and gas companies have been exploring the earth’s subsurface for over a century. As such, they have trillions of data points stored across a variety of different data storage units.
One of these is seismic data tapes, which store seismic recordings from surveys conducted by oil and gas companies to determine whether or not a geolocation is likely to harbor oil. Iron Mountain claims they can transfer the recordings on these tapes into digital formats before they are completely degraded, and they could be added to the company’s searchable database.
As a result, geoscientists could use an information extraction software with machine vision capabilities for seismographs from 40 years ago to better inform where to recommend their company drill next.
Harmonizing Metadata From Different Sources
Metadata, or data that describes other data, is in many cases essential for the development of NLP-based search functions because it often puts data into implicit categories that refine the results from search queries.
In other words, an NLP-based document search software in many cases uses metadata to organize documents and the information within them, thus speeding up the search process for geoscientists and other employees at the oil and gas company.
For example, the physical location of a high-performing well is one datapoint, but there exist millions of theoretical data points that could describe that physical location: data about its geology, the entity from which it was purchased, and its seismic activity, among others.
Much of this information is crucial for geoscientists when making an informed recommendation on where the oil and gas company should start drilling.
This metadata is often stored in a variety of locations, however, including within the documents (both digital and physical) at the oil and gas company. According to Conzelmann:
There is metadata available already. It’s important to synch that up with the digitized content. Being able to take what we generate from an ML perspective alongside the existing metadata and be[ing] able to create a relationship between them is where the power comes in of enabling those geoscientists to find all of that stuff and spend most of their time analyzing instead of finding the info they need.
Metadata is also often available for purchase from third-party data vendors or available for free from public databases. The latter is often the case for specific properties that the oil and gas company may have recently purchased.
Creating a database on which to run NLP-based information extraction software would require the labeling of company assets with all of this metadata, combining the metadata in a single location for the purposes of the AI use-case, a process known as data harmonization.
Integrating an AI solution like Iron Mountain’s would require a harmonization between the oil and gas company’s existing metadata, which they may not currently be aware of, metadata from purchases, and public metadata before any machine learning algorithm would be able to generate insights about a given property, well, or other asset, for example.
The Bottom Line – What Oil and Gas Companies Need to Know
Conzelmann gets to the heart of a key issue with geoscience work at oil and gas companies: geoscientists (and the companies that employ them) would rather spend their time analyzing well and seismic data to make decisions on where to drill; they don’t want most of their work day to go to searching for the information they want to analyze.
Few AI vendors cater to the oil and gas industry specifically, and many of them offer predictive analytics software that necessarily requires volumes of digitized data. Oil and gas companies may first want to focus on digitizing their paper documents, tapes, and other physical data storage units before embarking on lengthy AI projects.
In doing so, they have a better chance of avoiding a scenario in which they hire several data scientists who at various points during the development process go to subject-matter experts and ask them where they can find data that the company simply doesn’t have digitally available.
At the same time, determining which documents an oil and gas company should digitize is likely to be done through collaboration between data scientists and subject-matter experts at the company. After the digitization, however, an oil and gas company may want to work with an AI vendor offering NLP- and machine vision-enabled search software.
Conzelmann puts the potential value of AI-based search applications succinctly:
The power of machine learning and AI is that you can do this at scale across millions of documents even when you’re dealing with disparate, different-looking content.
Information extraction software could help oil and gas companies save on geoscience labor costs and drive new revenue in the form of more and higher-performing oil wells.
This article was sponsored by Iron Mountain, and was written, edited and published in alignment with our transparent Emerj sponsored content guidelines. Learn more about reaching our AI-focused executive audience on our Emerj advertising page.
Header image credit: Star.kiwi