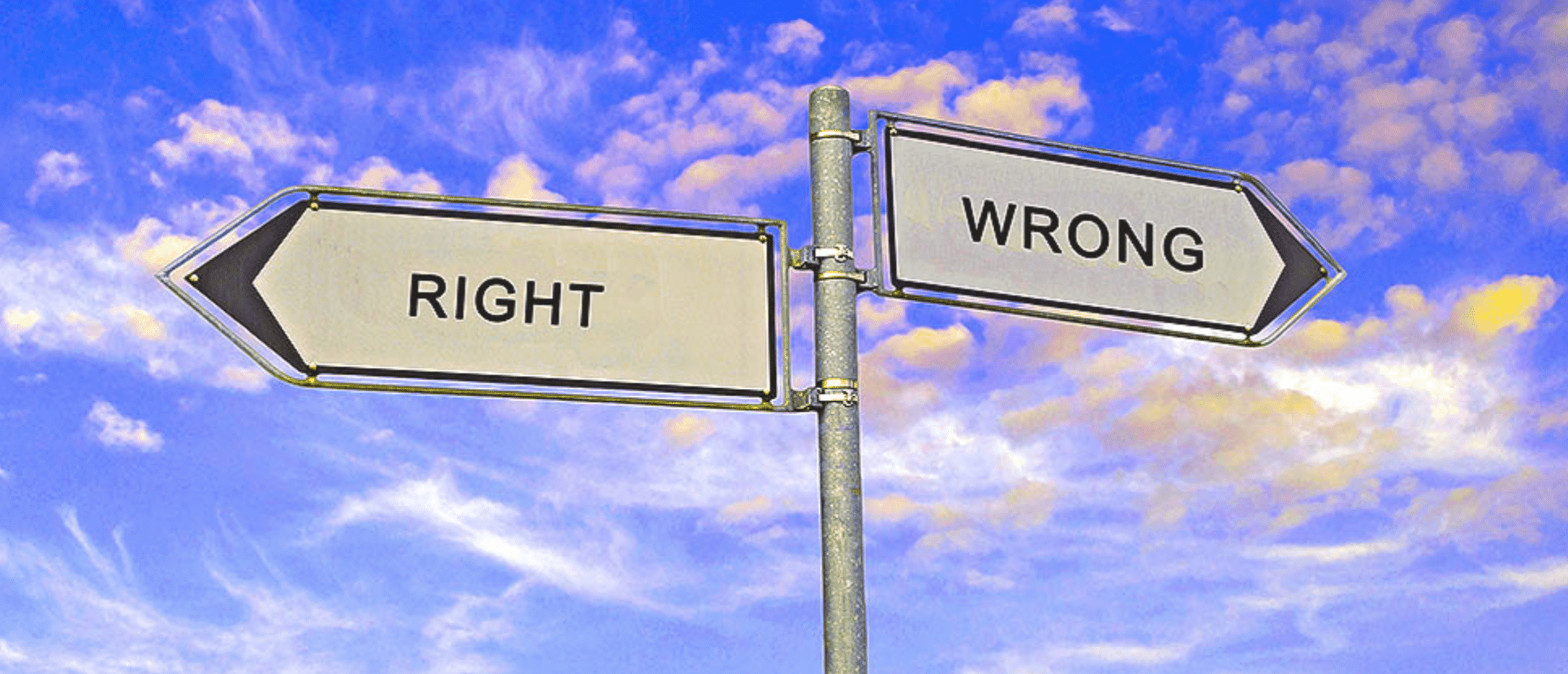
This week on AI in Industry, we are talking about the ethical consequences of AI in business. If a system were to train itself to act in unethical or legally reprehensible ways, it could take actions such as filtering or making decisions about people in regards to race or gender.
When machine learning is integrated into technology products, could a machine learning system put the company at financial and legal risk?
Our guest this week, Otto Berkes, Chief Technology Officer of New York-based CA Technologies, speaks to us about realistic changes in the technology planning and testing process that leaders need to consider. We discussed how businesses could integrate machine learning into the products and services, while still protecting themselves from potential legal downsides.
Otto Berkes and Dan Faggella will both be speaking at the AI event titled “Artificial Intelligence and Business Ethics: Friends or Foes?”, at the University of Notre Dame, Mendoza College of Business on September 19, 2018. Learn more about the event here.
Subscribe to our AI in Industry Podcast with your favorite podcast service:
Guest: Otto Berkes, Executive Vice President and Chief Technology Officer, CA Technologies
Expertise: technology vision, strategic research, cloud computing, mobile technology, software development
Brief Recognition: As CTO of CA Technologies, Otto Berkes is responsible for technical leadership and innovation, as well as ensuring that the company’s software strategy, architecture and partner relationships are aligned to deliver customer value.
His 25-year industry experience encompasses pioneering mobile computing and leading the development of touch-based technologies and designs. Prior to CA, Otto served as CTO at HBO.
In his prior 18 years at Microsoft, Otto was one of the leaders behind the Xbox and the Windows NT operating systems. He led Microsoft’s OpenGL and DirectX graphics development groups in early years of the modern GPU. He also served as a senior developer at Autodesk where he wrote the graphics engine and user interface for the first Windows-based version of AutoCAD.
Otto earned a bachelor’s degree in physics from Middlebury College in Vermont and a master’s degree in computer science and electrical engineering from the University of Vermont. He is co-inventor on and holds multiple patents spanning design, mobile device interaction and core computing technologies.
Interview Highlights
Daniel Faggella: There aren’t many concerns about the ethics of software, so what is the difference with artificial intelligence and why should business leaders care now? Besides job automation, what actually matters?
Otto Berkes: There is a real difference between how algorithms and programs have been built and how we think of programs using machine learning and machine intelligence algorithms. Previously, you could hardwire bias into software programs and algorithms but they were discoverable while the source code was being written.
Now we have systems that change their behavior over time or may have behavior that is only discovered when presented with certain conditions that were not anticipated. I would say it is the non-deterministic nature of machine learning and machine intelligence systems and their ability to change over time in ways that we do not anticipate that makes a big difference.
DF: It [machine learning] is their black box or evolving nature. If you design it with the system, like a set of buttons that is biasing people towards taking certain actions, you just redesign it and how it works. With machine learning, whether it is a recommendation engine or a fraud detection system, you don’t have that security now or in the future.
OB: It comes down to explainability and how your intelligent agent comes up with an answer. With hardwired algorithms, you can look at the code and know exactly how you got the answer. With intelligent systems, explaining the answer is a very different process.
DF: What are some examples of black box or machine learning scenarios that could pose legal and financial risk?
OB: One example is in the HR (human resources) space. We see tools for expense reporting starting to use machine learning and machine intelligence algorithms to drive greater efficiency and potentially prevent abuse. But there is also the potential to unfairly single out certain classes of people that was not intended. It makes a statistical decision without necessarily taking into context the bigger picture.
DF: That’s how human biases and stereotyping come about. How do you keep machines from making these biases even though the data shows it?
OB: We have to keep in mind that humans could be involved in the process of making sure that results are fair and ethical. There is a data science component here. The learning algorithms are only as good as the quality of the data they ingest. So make sure that the data itself is a fair representation of a body people or a certain problem. Active monitoring of these systems could ensure they are performing as intended, and detect anomalous behaviour that was not anticipated or does not represent the desired outcome.
DF: I imagine that a system for HR is periodically tested. It is fed a voluminous amount of data about different types of people. Are those bending ways that could be legally unacceptable? Would we hypothetically decide tactfully which data not to include in the system?
OB: It’s actually both. You bring up a good point to cover: testing. We’ve thought about testing in a rigid sense, in that it was geared deterministic hardwired algorithms. Now, we have to rethink what testing means. When algorithms themselves are dynamically changing their own behaviour, testing itself has to change and be rethought. Your point about data scrubbing and cleansing is spot on. It’s up to the humans to understand how certain data can inject biases into the system.
DF: You said testing needs to be rethought. What do you think other CTOs should consider and take action on?
OB: Testing in this new world will come back to data, making sure that you have the right mechanisms to harvest the right data. You cannot stress-test algorithms without known pools of data.
The other thing to keep in mind is that the behavior of these systems will change over time. Testing should be able to track changes in the answers that these machine learning algorithms are producing. You can’t test the systems to be a snapshot in time and have 100-percent confidence. You have to track the behavior of the system over time using data-intensive methodology so you can identify the drift, to see if there is some bias emerging.
DF: I would imagine an ongoing process of feeding new samples to the machine and getting responses. Do we have a system that tests for something that could be a legal risk or unethical?
OB: Absolutely. The potential reputational damage from unfairly singling out a class of people for example, in a payment security or payment fraud-related application, can be a mess. Something that the entire industry needs to guard against. It’s an ongoing challenge. You can’t just rest with a given solution and assume it will be acceptable.a month or a year from now. We need to evolve these solutions to stay ahead of the unintended consequences.
DF: I imagine a future where all kinds of machine learning-predicated products have an ongoing process where samples are programmatically generated and fed outside the operating system to test the decision-making process against known legal and ethical risks of that product. Do you see the same or a different future?
OB: That is absolutely right, and all the while maintaining privacy. The irony is that the more data that you have, the more precise you can be in having a representative set of data, but the greater the risk that you encroach on people’s privacy.
One of our research projects is tackling that problem. At a very simple level, it injects noise into personal data that anonymize people’s identities, while still retaining the valuable information to help program intelligent systems.
DF: You brought up two polar concerns. We have maximum amount and granularity of data so that we can train on as many features and deliver the best results for the business. On the other hand, we have privacy and security concerns. Considering the balance and ongoing testing to prevent legal and ethical risks can be a part of a CTO’s role in the near future.
OB: It will require us to rethink what privacy, bias and security mean. How well-intended intelligent systems could have massive, unintended consequences to businesses.
DF: Is it possible to have “unacceptable data” that could help a machine make better decisions even if it is socially uncouth and it may behoove companies to hide it. How will enterprises get around that?
OB: One aspect that I’d like to throw in here is the degree to which a machine learning algorithm is simply a data point for a human being to make a decision or whether the machine itself makes a decision without human intervention. That’s where we need to draw a very fine line.
Let’s assume data got into the system and makes a recommendation that includes the reason it voted against a person applying for a loan. The machine will allow a human to make a decision based on fuller context around each individual that the machine does not have.
DF: Can we create explainable systems that might take all the data, evaluate the considerations, make recommendations and allow humans to use their judgment to decide? For the tricky areas that require ethical responsibility, could we have a human in the final stage of the process?
OB: Absolutely and very well said. We have a research project around explainable AI. In examples where it is potentially prickly, the machine has to explain how it got the answer.
DF: Business and technology leaders will be thinking: Would there be some basis for the decisions in these applications? Or would the produced results be ethical, legal or financial risks? Can they think of ways to train and test these systems to prevent these risks?
OB: It is powerful technology. The confluence of powerful hardware and the ability to implement these software learning algorithms have great potential. But the onus is on us, the human beings, to supervise and monitor and evolve these systems and make them a positive part of our future.
Subscribe to our AI in Industry Podcast with your favorite podcast service:
This article was sponsored by the University of Notre Dame, and was written, edited and published in alignment with our transparent Emerj sponsored content guidelines. Learn more about reaching our AI-focused executive audience on our Emerj advertising page.
Header image credit: Book Business Magazine