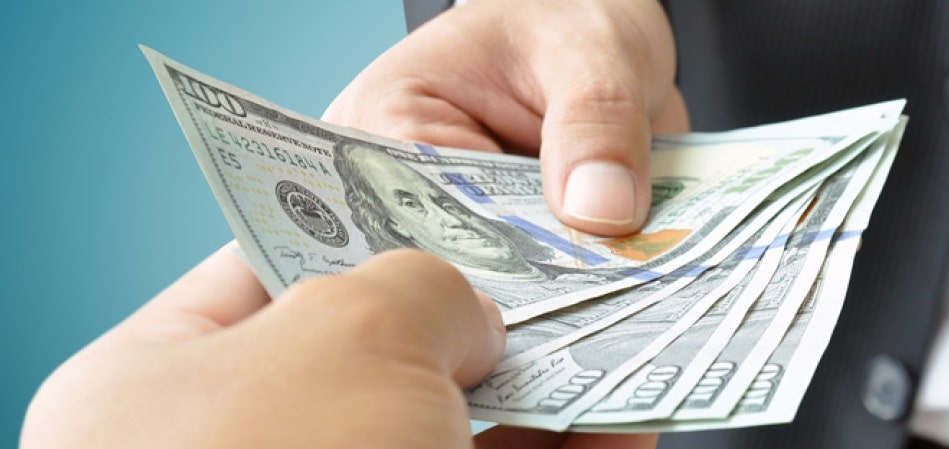
Automated loan processing and underwriting is not a new concept in the banking and financial services industry. Lenders have consistently faced pressure to reduce the costs and time associated with internal loans processing and turnaround.
Lenders have tried automating parts of the loan process including customer segmentation, loan approval, loan monitoring, and automatic loan pricing. These attempts were simple rules-based software programs that were set up based on indicators such as a credit score to make a decision on whether a loan would be approved or not.
These systems took in a small number of data variables (usually around 10-20) to make a decision on loan approval. In cases of ambiguity, the software automatically deferred to a human loan officer.
AI systems could help lenders identify and track more variables to build a more accurate picture of a customer’s financial state. Arguably having more information about the customer can help underwriters understand whether or not an applicant is likely to default on loan repayments.
AI systems can potentially be applied to lending to take advantage of the customer information now available to lenders. In an effort to understand how AI technologies are being deployed by leading lenders, we spoke with Anwar Ghauche and Carlos Pazos of SparkCognition.
According to Ghauche and Pazos, the processes involved in lending are interconnected, and there might be merit to automating the entire process from loan origination to monitoring of the repayments.
Even if a bank improves the turnaround time of the loan approval process, they will add value only when they reach the right customers, which in turn means their pricing needs to be optimized. Many lenders often have some of these processes partially automated while others are done manually.
Lenders are starting to use AI to improve the efficiency of their loan processes beyond what is possible with traditional software. Gauche relayed insight into where the areas of AI disruption are currently in automated loan processing and underwriting when he said:
Once an organization has developed an automated loan model (non-AI), what they need to do is monitor the loans given out and monitor the default and delinquency rates of those loans eventually overtime they would tweak those rules to reduce the amount of underwriting. Lender have identified 2 things that can be improved in this process
- There is a lot of external information that can be useful to assess the creditworthiness of an individual
- If you can connect the analysis of the automated loan performance to the system that approves the loans, you can optimize the system to give out more loans with fewer default rates.
In this article, we breakdown the advantages that AI systems might bring for lenders in terms of improving loan process and other common use-cases. We use the following structure based on our conversation with Ghauche and Pazos for that purpose:
- AI for Assessing Credit Worthiness
- AI for Overall Loan Process Automation
For more on how machine learning can automate the lending process, download SparkCognition’s white paper.
We begin with how AI can help add value to credit scoring efforts from vendors.
AI for Assessing Creditworthiness
AI can potentially help financial agencies determine the creditworthiness of borrowers and reduce loan defaults using predictive analytics and natural language processing.
While traditional software models can track a few variables to determine credit scores, machine learning models could be trained to create credit scores by using thousands of data variables from customer information such as social media posts, geolocations, browsing activities, and other data points. AI software could help lenders use all of this data to more effectively identify which data points and patterns in the data correlate to the types of borrowers that are most and least likely to pay back their loans.
In this way, lenders could predict the creditworthiness of customers who have little or no credit history, which may be difficult with rules-based engines. Since bankers are traditionally risk-averse, the rules in these engines are usually highly conservative, leaving out several potential customers:
Automated loan processing and underwriting has been around for a while now. It started with smaller loans, credit cards, and personal loans, but today we can even see them in auto loans and mortgages.
Now ML is coming in to replace those rigid legacy rules-based models because they are more scalable and able to be updated with more information. The system can get better over time by automatically tweaking the rules.
Although credit scores provide an objective way of determining someone’s creditworthiness, they may not help those without credit histories. AI could prove useful for allowing loan applicants to get approved for loans they wouldn’t normally have access to.
AI applications are being used by lenders to attract clients from groups with traditionally low credit histories, such as Millennials or customers in developing nations.
AI vendors offer products that can take into account a customer’s FICO score, level of education, SAT scores, GPA, field of study, and job history to use machine learning to predict an individual’s creditworthiness.
Underwriting loans involves a lot of data processing work, and AI software is starting to make inroads in applications such as automated document digitization. But most lenders today have only basic information verification systems, and human underwriters still review a majority of the loan applications.
Bankers might tend to try and control losses by tightening up the lending rules for approvals, but this also results in more false negatives where potential customers might be left out.
Alternative data sources could all help feed an AI system that can help automate the underwriting process more efficiently than traditional software.
AI for Overall Loan Process Automation
Lenders are looking to increase the number of loan approvals from incoming customer applications while reducing loan default risk or at least ensuring no significant change.
Machine learning solutions and automated machine learning platforms, such as SparkCognition’s Darwin, could be useful in developing self-learning systems to manage each part of the loan process more efficiently.
Sales and Marketing
AI systems could help banks identify the right customers that are likely to buy their products. Some software use unsupervised machine learning to cluster large amounts of customer information into meaningful segments that banks can use to target the right customers.
For instance, AI software can be fed with customer information who have repaid loans before and then trained to identify which new customers fit a profile that is similar.
Loan Approvals
Loan approval processes are usually the biggest roadblock for lenders looking to drive revenue. AI software and autoML software could allow for automatically reading through customer applications, generating their credit score, and prompting whether the loan should be approved or not. In cases where the software cannot make a decision, human loan officers are alerted.
Although this capability is not generated because of AI tools, the advantage of using AI is that the number of false-positive and false-negative cases could be reduced compared to traditional software.
The experts we spoke to also agreed that the process of tracking and monitoring loans for delinquency and default could benefit from AI upgrades. AI models could continuously track all the incoming payments and predict a loan default score that is updated over time.
If a customer has been identified as being at high risk of default, then the software alerts loan officers to enable communication with the customer to work out a different deal. Ghauche added the following insight regarding how AI may help with loan performance monitoring:
Lenders that realize they have cost pressure, they don’t want to have large call centers and large teams for manual underwriting, and they need to reduce their number of calls. This is where automated underwriting using AI is coming into place.
Using autoML those companies can track the performance of loan to better inform and lower the bar for automated loan processing.
Given enough historical loan performance and customer data, AI software could also predict what the most optimized interest might be for each customer. This information could also be used to track which groups of customers seem to have the lowest default rates or which groups are at high risk of default.
Customer Experience
One other added benefit from using AI technologies for automated loan processing and underwriting might be improved customer experiences. While AI software can help reduce the time taken for loan processing and suggest a price that is most convenient to that particular customer, the customers are gaining from not having to wait for long before their loan is approved, which in some cases can be almost instantaneous.
Concluding Thoughts
The advantage of machine learning systems might be significant in the long term for the early adopters of the technology. Immediate returns might still be limited since the software learns to accomplish tasks better over time. Automating the complete loan process can potentially lead to compounded cost and time benefits for lenders, which is an advantage that might help them stay competitive in the future.
We expect that machine learning will be applied to credit scoring processes more frequently among banks and financial institutions in the future.
This is largely due to the fact that banks with conservative rules-based loan approval systems have not tapped into the significant pool of potential customers for mortgages, payment plans for products, and credit cards, among those that don’t have any real credit histories.
Although AI systems are now starting to be deployed by lenders, this use-case is still in its nascency. These types of AI projects require time, data science resources, and lots of capital to integrate, and the systems are usually only as good as the people who set it up. Readers may want to note that these AI applications are new and still haven’t been fully tested.
In some cases, AI algorithms can be trained to give an output result that is desirable without actually having the software understand the underlying logic in loan processing. This might lead to banks working with vendors to deploy a solution that does not help identify the right kind of customers.
Banks might find even when using machine learning that feeding off of customer digital footprints (purchase history, app use, search history, social media activity, etc), loan approvals might not be significantly higher than traditional software immediately. Machine learning integrations require maintenance and upkeep in the form of fine-tuning algorithms to account for new data.
Artificial intelligence has the potential to disrupt lending, allowing those companies that leverage it effectively to offer risk-based pricing, increase approvals, and reduce risk. Adoption in the lending space is relatively slow, but risk-focused leaders in banking and other lending-related industries are likely to focus their innovation wings on automating risk-related processes, loans and lending included.
This article was sponsored by SparkCognition and was written, edited and published in alignment with our transparent Emerj sponsored content guidelines. Learn more about reaching our AI-focused executive audience on our Emerj advertising page.
Header Image Credit: FinTech News