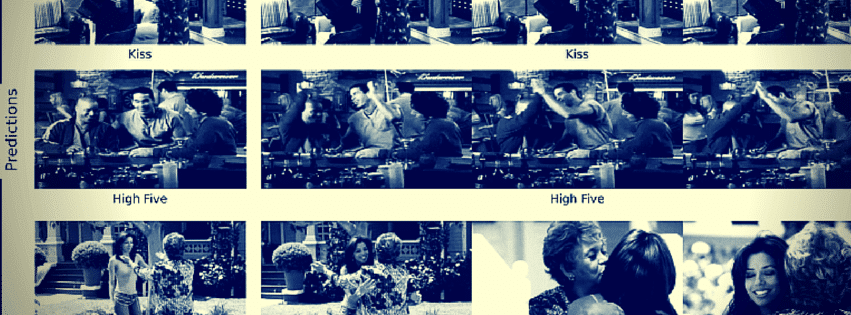
1 – Sucking Robot Arm Wins Amazon Picking Challenge
A Dutch robotics team was awarded the top prize in Amazon’s most recent warehouse bot competition in Germany. Team Delft’s bot excelled in two tasks: selecting and picking products from a container and putting them on a shelf, and performing those actions in reverse. Teams were given a computer file, five minutes before the start of the competition, that detailed the range of objects involved and instructions for moving each. Once the task began, the robots acted autonomously .The competition is held to with the ultimate goal of furthering collaboration between humans and robots in Amazon’s warehouses. At present, robots move items around the building, but humans are still relied upon to stock shelves.
(Read the full article on BBC News)
2 – Google’s New NHS Deal is Start of Machine Learning Marketplace
DeepMind, in partnership with Moorfields Eye Hospital in London, is training neural nets to spot patterns associated with two common eye diseases – age-related macular degeneration and diabetic retinopathy. Over 100 million people worldwide suffer from one of these diseases, and the resulting damage (at present) is irreversible. Neural nets have the potential to spot subtle patterns that hint at oncoming diseases before a human clinician. The experiments are not guaranteed, but Mustafa Salesman, DeepMind’s director of its healthcare research team, believes the potential to glean valuable insights (and at no cost to taxpayers) is worth the airing out of data that would otherwise be locked up and away from the eyes of clinicians.
(Read the full article on The New Scientist)
3 – Microsoft Now Helps Businesses Use the Data that Powers Bing Predicts
On Thursday, Microsoft released a version of its Cortana intelligent assistant with the integrated power of its Bing Predicts service, which combines machine learning algorithms with big data from social, search and the web and has the potential to provide businesses with more accurate information on which to base solutions. This technology is being sold as an end-to-end consulting service and is available (for an unpublicized price). Users will work with a Microsoft research team to help interpret the data gleaned, characteristic of a current consultative industry trend for businesses who have limited resources for building up a full in-house data science team. On a related note, Microsoft also announced that it will host its first Data Science Summit in Atlanta in September.
(Read the full article on TechCrunch)
4 – Russian Facial-Recognition Startup VisionLabs Raises $5.5M After Partnering with Facebook and Google
Russian-based AI startup VisionLabs announced its most recent securement of $5.5 million in funds from Sistema Venture Capital in exchange for a 25 percent stake in the company (estimated to be worth $21.5 million). In addition to software packages Luna and Face_IS, VisionLabs partnered with Facebook and Google in 2015 to create an open-source computer vision platform, which integrates OpenCV and Torch libraries centered on deep learning technologies, to help further developers’ progress in creating artificial intelligence-based products and services. Sistema Venture Capital was founded by Russian-based AFK Sistema, which has already funded over $300 million in targeted startups to date.
(Read the full article on VentureBeat)
5 – Are Face Recognition Systems Accurate? Depends on Your Race.
Machine learning systems are not biased, but the training they receive based on data may have unchecked biases that affect the training outcomes of systems – and the facial recognition systems used by the FBI may be an example of such a case. This past June, the Government Accountability Office released a report that revealed the FBI has not satisfactorily tested its face matching software or the state-level databases from which it sources information. While 95 percent of images are accurately matched under controlled conditions, imperfect conditions – like bad lighting or awkward angles, as well as the batch of data on which the system is being trained – leads to more inaccurate or skewed cases of identification. Though the technology has improved over the last five years, inaccuracy remains a problem, and new deep-learning technologies like those from Google and Facebook are still suspect to being skewed based on the batches of data provided.
(Read the full article on MIT Technology Review)
Image credit: BBC News