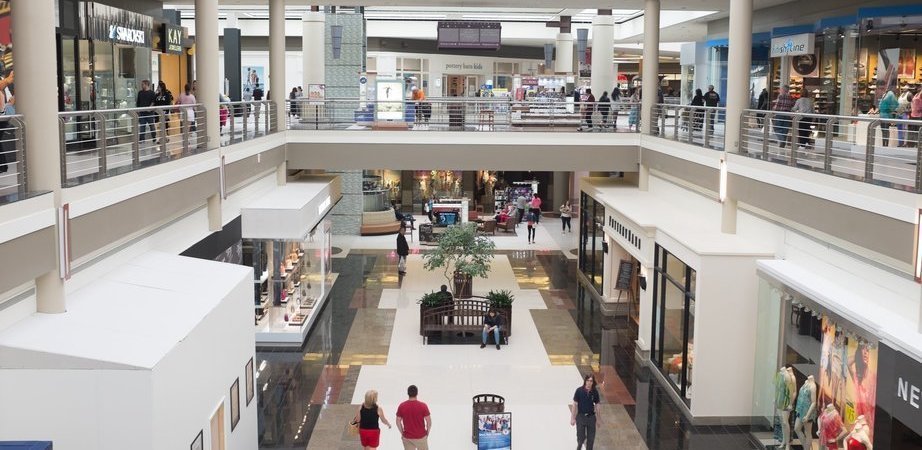
Modern AI and machine learning software require large sets of data in order to train its algorithms to make judgments, make predictions, and take actions. Data is a critical part of bringing artificial intelligence to life in different industry sectors. The applications we’ve highlighted below involve organizing historical and real-time data from existing businesses in the retail sector from which data scientists can build machine learning models.
While not all AI applications require existing stores of rich data on the part of the buyer company, many predictive analytics models do. The intention of this report is to demonstrate how existing data stores and real-time streams of data can be leveraged by different AI vendor solutions to bring value to a business. The goal of this report is to highlight the value of big data in the retail sector to show how a company might manage their data to create machine learning models that could be put to productive use in a company.
The World Economic Forum recently forecast that by 2026 the retail and consumer packaged goods industries will reach $2.95 trillion in value. The report explains that 32% of this value will be the result of the shift away from brick-and-mortar stores to eCommerce, while the remaining 68% represents cost and time savings on the part of consumers.
Big data powers AI, and so it follows that AI would continue to find its way into the retail industry. Numerous big data companies claim to assist marketers, retailers, and eCommerce companies in managing their data in a way that would allow them to personalize customer engagement, forecast inventory, and segment customers.
We researched the space to better understand where big data and AI comes into play in the retail industry and to answer the following questions:
- What kinds of data management platforms are available to retailers looking to leverage AI?
- How are big data-driven AI applications used in the retail industry?
- What tangible results have big data-driven AI applications garnered for retail companies?
This report covers vendors offering software across two applications:
- Data Management
- Building Machine Learning Models
This article intends to provide business leaders in the retail space with an idea of what they can currently expect from big data management applications selling into the retail space. We hope that this article allows business leaders in retail to garner insights they can confidently relay to their executive teams so they can make informed decisions when thinking about data management for AI adoptions.
Data Management
Reltio
Reltio is a California-based company that offers the Reltio Cloud, a data management platform which the company claims can help retail businesses organize enterprise data of different formats that comes in from a variety of sources. The company claims the way it organizes enterprise data could enable data scientists working at retail companies to creature machine learning models that drive business value.
Data scientists at retail companies can first upload the company’s database of customer information and past transaction history into Reltio’s software. Then, the data is organized in a way that allows data scientists to filter customers based on specific attributes and create segments out of these filters. This could allow data scientists to build machine learning models for use in product recommendation engines and for providing predictive analytics that could inform marketing campaigns.
Below is a short 7-minute video from Reltio explaining how Reltio’s platform might prove useful for businesses with large volumes of data:
Reltio claims to have helped an unnamed global fast food restaurant chain increase rework its customer loyalty program across more than 40,000 of its outlets in more than 100 countries. To do this, the restaurant chain needed a consolidated view of its customers from all channels.
The client used Reltio Cloud’s Consumer 360 tool to view the details of each customers’ profiles, track their previous online purchases, group household members together for promotional purposes, and create customer segments with similar attributes.
According to Reltio, this enabled the client to learn about their customers’ preferences, behaviors, product interests, and choice of channel (social or search, for example). Being able to segment customers also enabled the client to send customers more personalized messages on mobile via messages apps and social media. No other details were provided. Additionally, we caution business leaders to bring a healthy skepticism to this case study because the client is unnamed.
Reltio does not reveal the names of its clients and its C-team does not seem to include individuals with robust AI experience. However, the company has raised $117 million in funding from Crosslink Capital, .406 Ventures, Sapphire Ventures, and New Enterprise Associates.
Dataiku
Dataiku offers a data science software called Data Science Studio (DSS), which the company claims can help retail businesses manage large volumes of data in order to help data scientists build predictive analytics models for the retail companies at which they work.
The company claims DSS is built for data scientists and those with similar roles. It is not a plug-and-play AI tool that business leaders should expect employees at their companies to use who are not data scientists.
With that said, the company claims data scientists could use DSS to build predictive analytics models that might help retailers price their products. Data scientists could sort through the retailer’s past purchase data and customer demographic data in DSS to figure out the likelihood customers will purchase certain products at certain price points.
A data scientist could also use DSS to build a predictive analytics model to aid in inventory management by predicting the demand customers might have for certain products based on dimensions such as time of year and region of the world.
Below is a short 1-minute video that briefly explains DSS and explores its interface. Once again, Dataiku’s DSS platform is built for data scientists.
Dataiku claims to have helped Coyote optimize its loyalty program and get customers to increase the use of its product. Coyote sought a method of segmenting its customers by their profiles and quantify how customers used its product.
Although Dataiku claims Coyote had success using DSS to build the model the company used to segment their customers and find a metric for product use, it is unclear how much DSS contributed to Coyote’s results. Somehow, Coyote reported an 11% increase in the efficiency with which they conducted outbound call campaigns as a result of the model they built from the data they stored on DSS. The case study does not report any details on the model Coyote reportedly built, and so it is again difficult to quantify how much Dataiku contributed to Coyote’s success.
Dataiku also lists BGL BNP Paribas, Sephora, Unilever, Fox, and GE as some of its past clients.
Thomas Cabrol is co-founder & Chief Data Scientist at Dataiku. He holds a 5-year degree in Analytical CRM from the University of Montpellier. Previously, Cabrol served as CRM Intelligence and Data Mining Manager at Apple Europe, where he developed a spacial analytics platform.
MapR Technologies
MapR Technologies is a company that offers a software called MapR Converged Data Platform, which it claims can help eCommerce businesses build a product recommendation engine on its platform.
MapR claims its software can hold large volumes of data relating to a customer’s demographics and behavior from a variety of channels, such as their social media accounts, past on-site search history, and past purchase history. Then, the software finds patterns in that data that might reveal the customer’s preferences, such as the colors, sizes, or styles they might prefer when shopping for apparel. This then allows data scientists to use MapR to build a recommendation engine to make informed product or service suggestions in which the customer might be interested.
eCommerce companies can expect three to 10 weeks of engagement with MapR’s professional services engineers and data scientists in order to integrate MapR’s solution into their sites, according to MapR. This integration service includes archiving data files, copying data to and from the MapR cluster, and building a user interface that collects customer data into one view.
Below is a short 5-minute video explaining how a company might set up a recommendation engine on MapR:
MapR claims to have helped Fishbowl house the client’s data infrastructure. Fishbowl is a data, marketing, and analytics solution provider that helps restaurant brands grow by enabling them to better understand and engage with their guests by utilizing their volumes of data.
The company was collecting and processing data in different forms and from several disparate sources. With a growing variety and greater volume of data requiring daily updates, Fishbowl sought a new and more scalable platform at a lower cost.
The case study further reports that deploying MapR’s data platform enabled Fishbowl to spend only 1/10th of what it was spending previously on licenses and 1/3rd of what it was spending previously on data storage. Fishbowl’s query speed also increased five to ten times more than it would have had Fishbowl used a different data platform, according to MapR. We could not verify if this was or was not actually the case, as MapR provides no context into that statistic.
MapR also lists Cisco, Boehringer Ingelheim, Credit Agricole, HP, Ericsson, Novartis, and SAP as some of their past clients.
Ted Dunning is the Chief Application Architect at MapR Technologies. He holds a PhD in Computer Science from Sheffield University. Previously, Dunning served as CTO at Deepdyve and Chief Scientist at SiteTuners.
Building Machine Learning Models
DataRobot
DataRobot is a Massachusetts-based company that offers a namesake software which the company claims can help retailers create machine learning models that can provide them predictive analytics that might be used to plan inventory, develop promotional campaigns, and find new paid media sources from which to buy.
First, data scientists upload the retailer’s database, which might contain customer information or transaction history, into the DataRobot software. Although we were unable to determine how the software might do it, DataRobot claims its software generates multiple predictive analytics machine learning models based on the retailer’s data.
Then, the software ranks these models based on an accuracy score the software provides them. This approach may allow data scientists to determine which models they might prefer to integrate into the retailer’s systems. Once the data scientist has chosen the model they prefer, DataRobot generates an API code that the data scientist can then use to integrate the model into the retailer’s systems.
Below is a short 2-minute video explaining and showing the interface in which DataRobot presents several predictive analytics models at once:
DataRobot claims to have helped Lenovo Brazil predict out-of-stock volume at retail stores to ensure the availability of inventory and meet customer demand. To do this, Lenovo Brazil required data scientists in addition to the two it already had on staff. However, data scientists were hard to find. Lenovo Brazil sought a tool that could help their team automate the creation of machine learning models that could predict out-of-stock volume.
The Lenovo Brazil team had identified factors that might affect out-of-stock volume at retail stores. These factors included average product price, rebate period, marketing campaigns, and the price differences between Lenovo Brazil’s products and those of its competitors. Lenovo Brazil wanted to know what factors truly affected out-of-stock volume.
According to DataRobot, Lenovo Brazil was able to increase the speed with which it built machine learning models by using DataRobot’s software.
Lenovo Brazil selected a DataRobot-generated model that allowed the company to figure out the largest factors that might affect the out-of-stock volume at their retail stores. They provided this information to stakeholders. According to the case study, this model made predictions with 90% accuracy, although we could not verify how accuracy was defined.
DataRobot also lists NTUC Income, DemystData, Steward Health Care, Avant, Symphony Post Acute Network, and Harmoney as some of their past clients.
Xavier Conort is Chief Data Scientist at DataRobot. He holds an MS degree in Statistics and Actuarial Science from Ensae ParisTech and another MS in Statistics and Stochastic Models in Economics and Finance from the Universite Paris Diderot. Previously, Conort served as Principal Research Engineer at the Institute for Infocomm Research, Secretary General & Risk Manager at AXA Insurance Singapore, and Chief Actuary and Finance Director at Sino French Life Insurance.
Takeaways for Business Leaders in Retail
All of the companies discussed in this report offer data management platforms, but only DataRobot claims to offer data scientists the ability to build machine learning models. We are, however, unable to determine how the company’s software might go about doing this, and the company’s Chief Data Scientist is not a PhD-level talent in computer science. The company has raised $124.6 million, possibly pointing toward its legitimacy. That said, of all the companies covered in this report, MapR Technologies has raised the most funding at $280 million.
Big data-based predictive analytics require large volumes of data on which machine models can make those predictions. The companies in this report claim to offer data management platforms capable of organizing and structuring data so that data scientists can work with it to build models.
Data scientists are the key to using these platforms. None of the companies discussed in this report offer platforms business leaders should expect employees to be capable of using unless they have a strong background in data science. Business leaders in retail should consider these platforms only if they are planning on having the resources to employ one or several data scientists to work with their data and build machine learning models.
Most of the use cases for big data analytics in retail involve developing more personalized content and marketing, segmenting customers, managing inventory and sales, and understanding customers better. None of the companies discussed in this report offer solutions specifically for retailers, although they do have case studies reporting success from retailers using their platforms.
Only MapR company specified a three- to 10-week integration period, but we can infer that because these platforms house a company’s data that the integration process could be lengthy, perhaps even longer than MapR claims.
Additionally, these platforms seem to be for enterprise retailers with large volumes of data that might have difficulty organizing that data. Smaller firms may not require the kind of storage and organization that the companies covered in this report offer with their platforms.
Header Image Credit: NYup.com