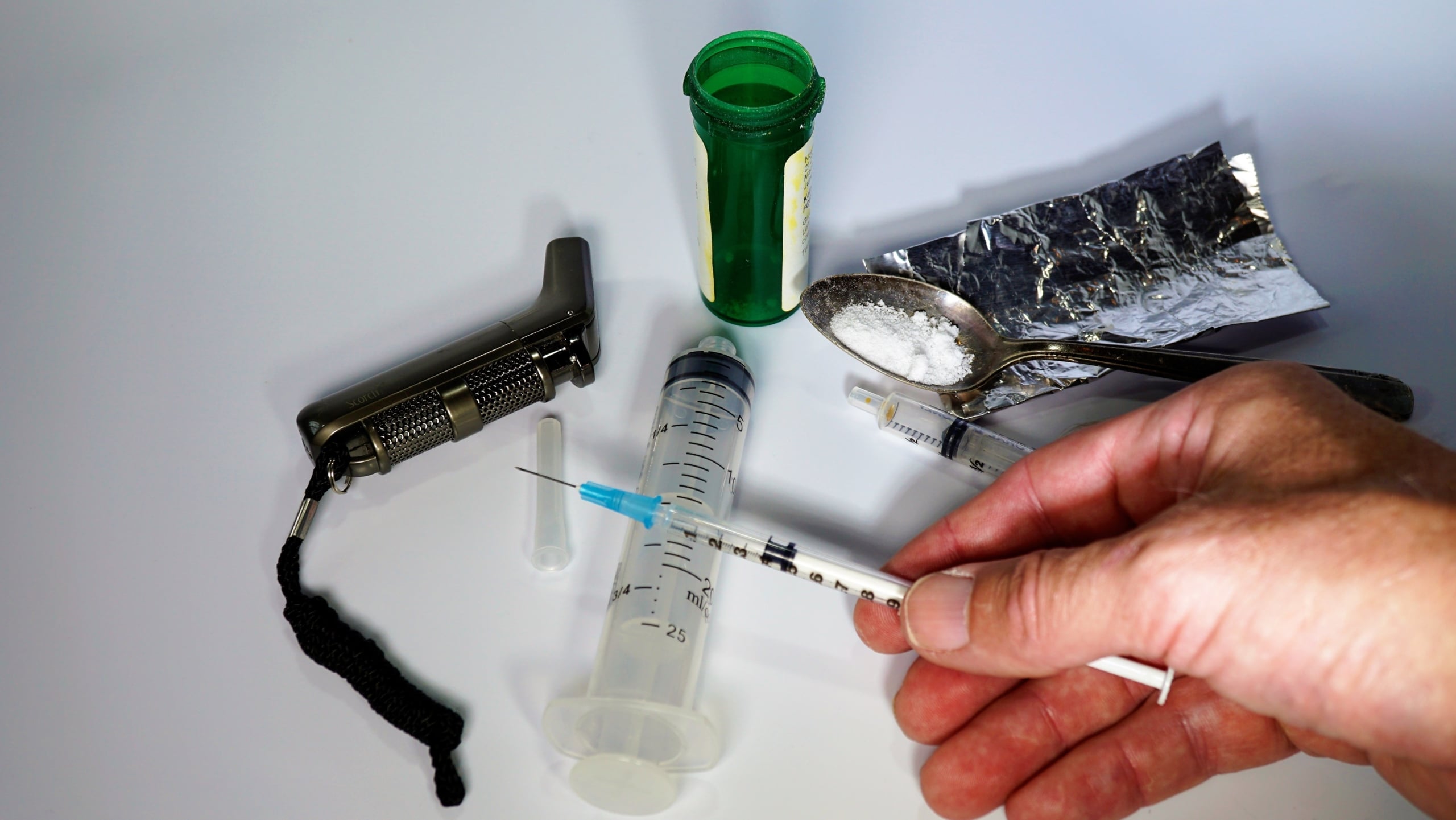
The opioid overdose crisis continues to maintain a strong grip on the nation. According to the National Institute on Drug Abuse (NIDA), the epidemic claims more than 115 lives in the United States every day.
The cumulative effects of this public health crisis also extend to loss of workforce productivity, addiction treatment costs, and criminal justice involvement. Research published in the official journal of the American Public Health Association has estimated the total economic burden of the opioid crisis at $78.5 billion.
Amidst research efforts focused on preventing substance use disorders, artificial intelligence is emerging as a component of technologies aimed at fighting the opioid overdose crisis. However, there is limited research to help healthcare business professionals navigate the growing amount of use-cases and their potential benefits.
In this article, we will explore the emerging role of AI in efforts to fight the opioid overdose epidemic, and include representative examples of use-cases and the potential benefits of these applications.
Background on Opioids and the Opioid Epidemic in America
Opioids are a class of medications prescribed by physicians to relieve severe or chronic pain. Common examples where physicians may prescribe opioids include patients recovering from surgery, pain associated with cancer treatment, chronic headaches and backaches or pain associated with sports or accidental injuries.
NIDA traces the beginnings of the overprescribing of opioids and subsequent incidences of misuse and addiction back to the late 1990s. Reportedly, the healthcare industry was reassured by pharmaceutical companies that prescription opioids posed a negligible risk of addiction. As a result, the rate of prescribing steadily increased.
However, it would be later discovered that addiction to these substances was indeed a high risk.
For example, in 2015 opioid overdoses contributed to over 33,000 deaths and prescription opioid misuse affected an estimated 2 million individuals in the U.S. that same year. While young adults from 18 to 25 years of age are among the hardest hit, the crisis is not limited to this age demographic and also impacts youth and the elderly.
Predicting Opioid Misuse Using Machine Learning
Hc1.com
Founded in 2011, Indiana-based hc1.com is a cloud-based, customer relationship management (CRM) software company for the healthcare industry. In November 2017, the company announced its hc1 Opioid Dashboard and claims that the platform integrates machine learning with the stated goal of detecting potential patterns of misuse and abuse among individuals who use opioids.
The company trained its algorithm on massive datasets, drawing data from an estimated 3.8 million providers, 51 million individuals and 5 billion diagnostic test results, across all 50 states.
For example, medical provider data on the number of prescriptions written in a given state may help to identify correlations between prescription volume and misuse. The algorithm can then learn patterns associated with incidences of misuse and abuse and predict potential emergencies before they occur.
The state of Indiana is among the companies partners which are prioritizing improving surveillance of the epidemic. In 2014, the state experienced 1,100 drug overdose deaths, generating over $1.4 billion in medical costs.
“We use machine learning to generate a view into what’s happening across America…it’s an early warning system. We can see leading indicators that allow us to predict where to put resources to lower the misuse of opioids.
Federal and state governments are deploying funds to reduce opioid use, but it’s very hard to measure impact. If we can look by county or ZIP code, it really helps to say whether an investment in a population is lowering misuse and abuse.”
– Brad Bostic, CEO, hc1.com (January 2018)
The image below depicts a screenshot of the Opioid Dashboard pulling data on the state of Indiana. Examples of indicators are visible to the far right such as zip code, age, and gender and rate with the regions at greatest risk of misuse and addiction highlighted in red on the map.
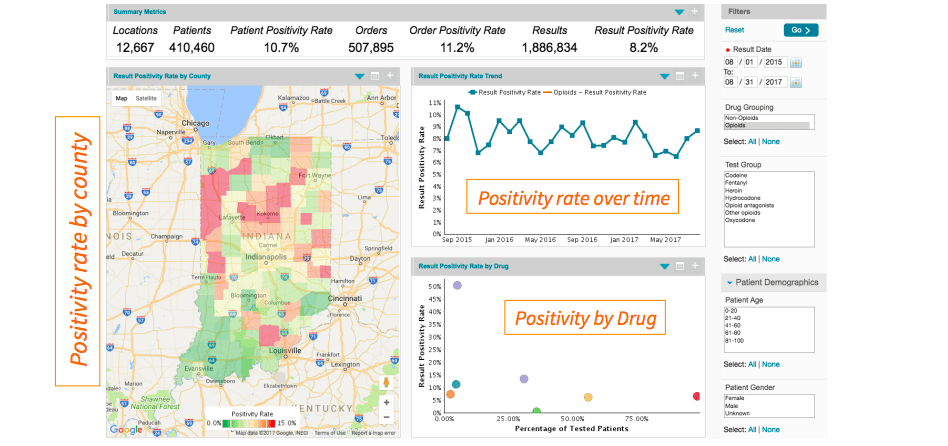
“Appriss Health and hc1 solutions are complementary and can be used in multiple ways to help states contend with the opioid epidemic. By delivering the dataset of drug positivity rates and insights along with prescriptions written statewide, we can empower state governments to better understand where problems are emerging. In addition, this data can be used at the point of care to give providers easy access to real-time data when writing prescriptions.”
– Rob Cohen, president of Appriss Health (April 2018)
Preventing Addiction Treatment Relapse Using Machine Learning
Triggr Health
Founded in 2014, Chicago-based Triggr Health claims that its platform uses machine learning to predict addiction recovery relapses to help target prevention strategies.
The company’s algorithms are trained to monitor smartphone data and activity such as texting patterns, phone location and sleep history seeking patterns indicating a stronger likelihood of a relapse. This information is combined with data such as client drug history and certain keywords such as “craving” for example, from communications between Triggr Health users and staff.
Clients are referred by their healthcare provider to use the Triggr platform as depicted in the screenshot below. The client would download the app to his or her smartphone for a user fee of $2 per day.
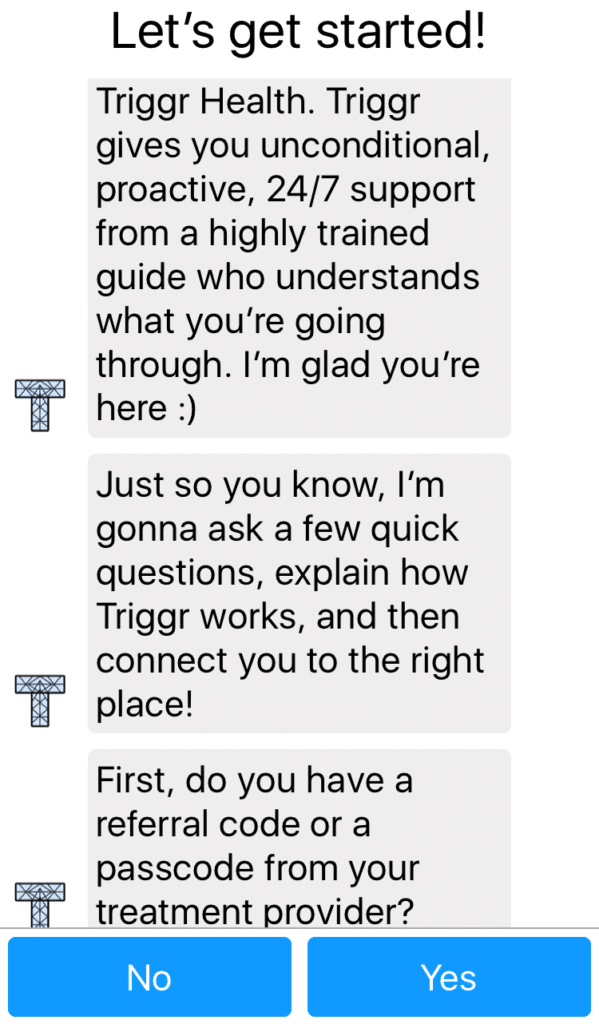
Triggr provides access to 24/7 support and in the event of risk reaching peak levels, a member of the individual’s addiction treatment team is immediately notified. The company claims that it maintains the privacy of user phone data and only has access to general information and not specific content.
The company claims that its platform achieves an accuracy rate of 92 percent at predicting regressive behavior, such as opioid misuse, three days before it would begin and is generally three times more effective than traditional treatment strategies. Traditional treatment approaches may include entering a recovery program at a rehab facility. With a goal of personalizing treatment, it also aims to support individuals for extended recovery periods, such as one or two years instead of shorter time spans which may not provide adequate support in every case.
“Over the past two years, we have worked with thousands of people at all levels of substance use and recovery as well as hundreds of providers.
Our technology leverages machine learning to understand the components of an individual’s behavior in real-time and turn evidence-based therapy into a proactive, in-the-moment experience that can dramatically alter the trajectory of opiate and alcohol-dependent individuals.”
-John Haskell, co-founder and CEO of Triggr Health (January 2017)
According to the company’s website, the platform is exclusively marketed to healthcare systems and providers at this time. Client acquisition follows a referral-based approach and the platform appears to be in pilot phase. The company aims to expand its app to individual consumers in the near future.
Behaivior
In June 2016, IBM launched the IBM Watson AI XPRIZE, a $5 million competition in an effort to expand the potential societal benefits of AI applications. The 4-year, global competition invites teams to develop innovative approaches to societal challenges. IBM Watson AI XPRIZE culminates in three finalist teams presenting their work at TED 2020.
Behaivior (an intentionally misspelled variant of “behavior”) is one of 147 initially accepted into the competition. The emerging Pittsburgh-based startup is aiming to help recovering opioid users in treatment avoid relapse. While the use of machine learning prediction algorithms is similar to the Triggr Health model, a main distinction is the integration of wearable technology which the company describes as somewhat similar to FitBit.
“We are creating software that can take real-time data streams from wearable devices that detect heart rate, heart rate variability, skin temperature, motion, and galvanic skin response (which is related to stress levels). This data is combined with other digital information about behavior, such as GPS location.
As behavior and physiology changes, our software screens users for whether or not they are in a pre-relapse craving state, and therefore at higher risk of relapsing in the near future. When people in recovery from substance use disorder are in a craving state, the propensity for relapse is high. By the time a traditional intervention occurs, they are already reusing again.” – Behaivior Website
The Behaivior team claims to draw data from IBM Watson and is also working in partnership with Carnegie Mellon University through their startup incubator, Project Olympus. Currently at the halfway mark of the IBM Watson AI XPRIZE competition, the startup is still in its early stages and its business model is not clear from its website.
Predicting Opioid Dependency Using Machine Learning
USC Machine Learning Center
Yan Liu, PhD, is a computer scientist with expertise in machine learning and director of the USC Machine Learning Center. In a 2017 study led by Liu, the researchers claim that they have developed machine learning algorithms to predict opioid dependency.
The algorithms were trained on a large sample size of 102,166 patients; a dataset that Liu claims is one of the largest for a study on addiction. The research team sourced data and worked in collaboration with the Mayo Health Clinic. Electronic health records were accessed through the Rochester Epidemiology Project. Liu emphasizes that patient privacy was a top priority and strict data-use agreements were upheld. All data, such as information on patient diagnoses and prescriptions, used was done so with patient consent.
Results from the study organized the users in two major categories: 79 percent were categorized as short-term users with 21 percent were considered long-term. A smaller percentage of 3.47 percent were categorized as opioid-dependent. However, this percentage carries another level of significance when compared to the 0.7 percent who were diagnosed as opioid-dependent prior to the study.
Through this research Liu aims to use patient medical history as a guide for machine learning methods so that opioid dependency can be identified before a physician ever prescribes opioids to patients at high risk. The machine learning study is part of Liu’s ongoing lab-based research efforts to bring tools to market to help combat opioid addiction.
Concluding Thoughts
The rate of opioid overdoses and misuse necessitates innovative and efficient approaches. In the medical field, multidisciplinary collaborations between professionals such as clinicians, researchers, public health agencies, and academic institutions are becoming increasingly common.
AI applications in this space appear to be focused on prevention of new overdoses or incidences of misuse by identifying patterns in users both in and outside of addiction treatment. Startups seem to be gravitating more toward developing applications for the addiction treatment support.
Addiction treatment will continue to be a priority at both state and federal levels. For example, Substance Abuse and Mental Health Services Administration (SAMHSA) provides grants for state treatment systems and support services.
Ideally, AI tools such as prediction of opioid dependency would add another level of value for federal investments in treatment services. However, personalizing addiction support platforms for every user in contrast to amassing data to identify trends can often drive up costs.
Therefore, cost-benefit analyses will be important to gain a more comprehensive perspective of the value of these platforms. Public health agency partnerships as in the example of hc1 make smart business sense and can serve as important data sources. This is particularly relevant for states with the highest rates of substance use disorder and opioid overdoses.
With the majority of studies and startups still in the early phases of their efforts, applications with ease-of-use for multidisciplinary teams would be expected to be implemented at a faster rate.
There are some significant challenges to bringing these applications to market which must be considered. For example, data privacy is a common challenge for health applications, however, the sensitivity of data on opioid use heightens the level of risk for patients
Current applications claim to use anonymous data or to obtain direct patient consent. Nevertheless, with a reported 1,768 healthcare data breaches occurring between October 2009 and December 2016, patient data privacy will be a major challenge going forward.
Another challenge to consider is a sustainable and profitable business model. Some of the highest rates of opioid overdoses have occurred in poorer regions in the country. Therefore, personalized treatment may not be accessible for the populations at highest-risk. Partnerships between government and businesses and government incentives for innovative applications in the fight against the opioid epidemic will become increasingly important.
Header image credit: Adobe Stock