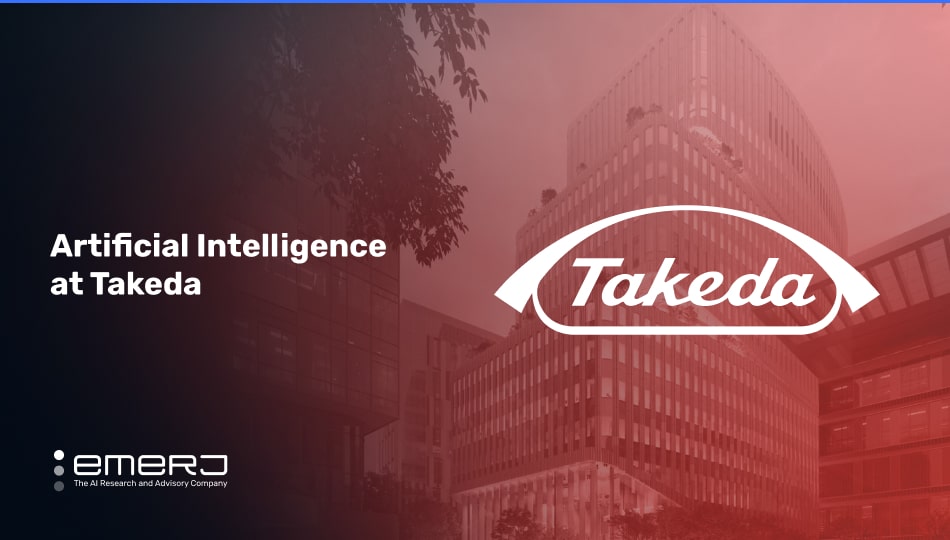
Takeda Pharmaceuticals is a 240-year-old, leading biopharmaceutical company headquartered in Tokyo, Japan, with a global hub in Cambridge, Massachusetts. The company has a global presence, operating in more than 80 countries across North America, Europe and Asia.
Takeda reportedly accrued approximately $30.3 billion in revenue for fiscal year 2022. The company employs about 50,000 people. The company is traded on the NYSE (symbol: ‘TAK’).
Takeda offers a diverse portfolio of products, including both pharmaceuticals and vaccines. Takeda focuses its research and development efforts on four therapeutic areas, including central nervous system (CNS) disorders, gastroenterology, oncology and rare diseases.
Targeted R&D investments are made in plasma-derived therapies and vaccines. The company conducts R&D both internally and externally, partnering with leading-edge institutions such as MIT to create innovative treatments.
Regarding its use of AI, Takeda appears to integrate several related technologies across its operations, including drug discovery and development, manufacturing and supply chain optimization.
In this article, we examine two timely and mature use cases for AI that speak to how these technologies support Takeda’s larger business goals and present robust opportunities for today’s life sciences executives:
- Procurement integration and automation: Using a combination of AI technologies, machine learning, and predictive analytics to integrate procurement systems post-acquisition, decreasing costs and increasing efficiency.
- Enhancing pharmaceutical production: Using AI-driven statistical tools, scientists diagnose and adjust factors affecting cell culture yield, improving the drug production process.
Use Case #1: Procurement Integration and Automation
Following its acquisition of Shire, Takeda Pharmaceuticals found it necessary to integrate and automate the former’s procurement platform.
The primary business problem stemmed from issues with scalability and usability with their on-premises solution. The previous system was configured on its own data center, limiting access to the system for 5,000 global employees who needed access.
Takeda required a robust solution capable of consolidating data and applications from both companies in a streamlined, cost-effective way. The company ultimately decided to utilize Bizagi’s Intelligent Process Automation (IPA) platform, a cloud-native platform-as-a-service solution. According to Bizagi’s documentation of the use case, IPA leverages a combination of different AI and AI-adjacent technologies to manage, automate and integrate digital processes.
Bizagi lists two specific AI-adjacent capabilities — Robotic Process Automation (RPA) and Digital Process Automation (DPA) — integrated within their IPA platform, along with what it describes as “artificial intelligence” but does not detail any specific, distinct AI capabilities. The case study documentation IPA is a low-code software designed to help the client organize workers, information and systems within the organization to achieve more accurate and efficient workflows.
Another way to summarize the company’s claims would be to say that IPA can most accurately be described as an advanced form of RPA that also likely leverages machine learning and other AI capabilities, such as predictive analytics. In Takeda’s case, they sought a robust, scalable solution capable of handling the additional work required to store and analyze Shire’s procurement system effectively.
According to company documentation, the Bizagi IPA platform consists of three separate yet integrated systems, each with different functions and processes:
1. Modeler: Visually diagram, model and document business processes in the industry-standard Business Process Model and Notation (BPMN) format.
2. Studio: Transform process models into functional applications, facilitating automation, integration, customization and control over business processes and workflows.
3. Automation: Run applications developed in Bizagi studio across devices and users.
The case report does not provide details regarding Takeda’s workflow within the Bizagi IPA system. However, we can extrapolate the likely inputs, outputs and changes to end-user workflow from the information available.
As the platform was integrated into the company’s procurement system with the primary objective of making it easier for business users to engage, these inputs likely included various supplier agreements and details, as well as documentation regarding pharmaceutical products. Data that was probably entered into the system include:
- Supplier names and contact details
- Product names, prices, quantities and other related details
- Order dates, the cost to the customer, products ordered and other related details
Takeda would have had to re-analyze procurement to incorporate Shire’s suppliers and products, requiring analysis of Shire’s procurement processes, supplier contracts, product details, pricing and schedules. End users would need to model new workflows in the IPA interface to account for scope.
Machine learning would be able to analyze procurement data to predict supply chain issues, identify improvement opportunities and create workflows for managing Shire products and suppliers not previously part of Takeda’s portfolio. Specific outputs likely included:
- Automated tasks for supplier selection, purchase order generation or invoice processing
- Integrated supplier data for enhanced supplier management
- Real-time monitoring and analytics for up-to-date procurement performance metrics
A screenshot of the Bizagi Studio dashboard. (Source: Bizagi)
Reportedly, Takeda was able to migrate its data and process documentation, providing access to the 5,000 employees previously without. The process took approximately four months to complete. It is reported that procurement activity increased and successfully delivered cost savings in about ten weeks.
Use Case #2: Enhancing Drug Production
Takeda’s Switzerland branch experienced issues increasing its cell culture yield (the number of cells produced in a lab for study) of blood clot drugs, hampering the remaining drug production process. Cell culture is an essential tool in optimizing the conditions for cell growth, and viability is critical to the drug development process.
The problem was that the process of understanding which parameters affect cell culture was labor-intensive, time-consuming and challenging to interpret for some.
According to a case study report from Minitab, Takeda’s partner on the project, AI-driven statistical tools such as Partial Least Squares (PLS) regression and Classification and Regression Trees (CART) were used to identify critical factors affecting yield.
PLS is thought to be helpful when:
- There exists a high correlation of predictors
- There are more predictors than observations
CART decision trees were used simultaneously to both confirm the results of the PLS analysis and make the data more accessible for scientists and engineers to understand and use.
According to Minitab, the analysis was conducted on its Minitab Statistical Software product.
Prior to the analysis, the analytics team monitored multiple culture batches while collecting data on approximately 30 process parameters. During the exploratory study, the team used feature engineering techniques (methods to create or modify dataset variables to produce a more robust, accurate predictive model).
Following the feature engineering, PLS regression and CART decision trees analyzed the process parameter data using their respective algorithms.
It is not mentioned which ‘process parameters’ were used in this analysis, but research indicates that the following variables may have been included:
- Dissolved Oxygen (DO)
- pH levels
- Temperature
- Nutrient Concentration
- Cell Density
- Agitation Speed
As an output, PLS describes the relationships between the predictor variables and one or more of the continuous responses. CART decision trees generate a Relative Variable Importance plot, ranking the variables on their improvement score.
A screenshot of a chart depicting PLS output of parameters from the Minitab use case documentation. (Source: Minitab)
A screenshot of a chart depicting CART output and relative variable importance plot from the Minitab use case documentation. (Source: Minitab)
Specific business outcomes for the project were not published. We can deduce potential effects based on Minitab’s report.
According to Minitab, the solution can correctly identify critical parameters affecting culture yield, which was the primary objective. Minitab cites the “alignment of CART decision tree results with those of PLS regression” as confirmation of the platform’s accuracy. Reportedly, the result is a more expeditious process.
If true, the platform likely has three significant business implications for Takeda:
- Increased efficiency: The ability to quickly identify critical parameters can significantly streamline the culture yield process, possibly shortening the time to market for new drugs that prevent blood clots.
- Cost reduction: By pinpointing critical parameters more efficiently, Takeda could reduce the number of experimental runes needed, thereby saving on materials, labor and operational costs.
- Improved yield: Identifying critical parameters with high accuracy directly correlates with the potential to optimize parameters that increase culture yield. These tangible results affect both efficiency and cost as well as the overall productivity of the drug development process.