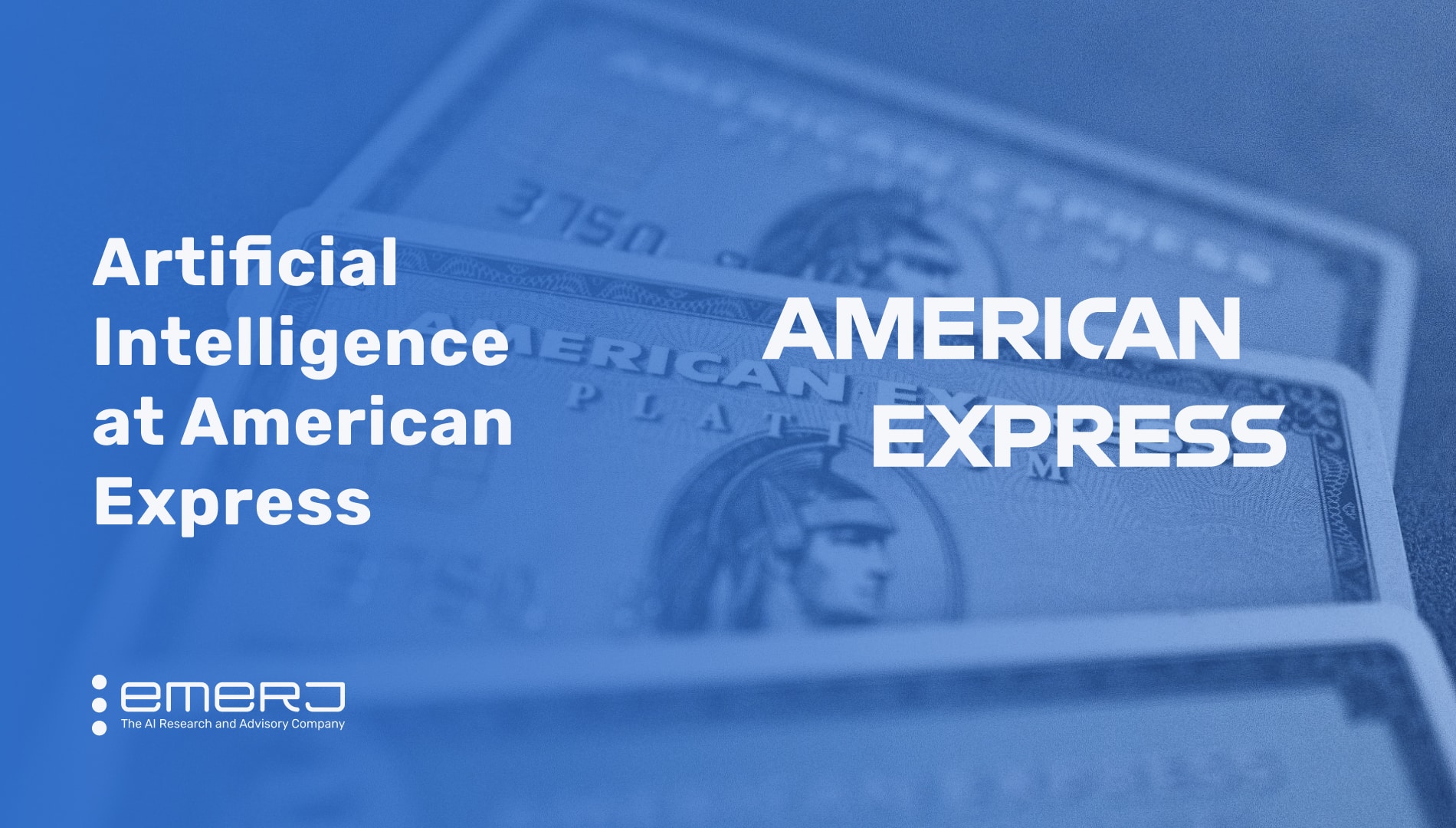
American Express began as a freight forwarding company in the mid-19th century. Expanding over time to include financial products and travel services, American Express today reports some 114 million cards in force and $1.2 trillion in billed business worldwide.
American Express trades on the NYSE with a market cap that exceeds $136 billion, as of November 2021. For the fiscal year ended December 31, 2020, American Express reported revenues of $36.1 billion, according to its Fiscal Year 2020 10-K.
American Express generates its revenue from merchant fees, interest on outstanding balances, and fees for card membership, late payments, foreign currency conversions, and other fees like those generated from its prepaid card and Travelers Cheque services.
American Express has established American Express AI Labs, which works with business leaders to ideate and advise on AI-based products and services. Its research areas include machine learning and optimization, natural language processing, AI products, document recognition and processing, and cloud platforms.
In this article, we will look at how American Express has explored AI applications for its business and industry through two unique use-cases:
- AI-Driven Fraud Detection — American Express has used its Gen X model to combat rising credit card fraud during the pandemic.
- Automating Customer Service Through NLP — American Express uses BERT models to determine customer intent and process requests through its award-winning customer service function.
We will begin by examining how American Express has turned to its tenth-generation fraud model to identify and eliminate fraud in the billions of credit card transactions the card issuer processes every year.
AI-Driven Fraud Detection
During 2020, Americans reported over 390,000 instances of credit card fraud, an increase of more than 40% over 2019 levels, according to research published by The Ascent, a service of the Motley Fool. In fact, the research found, credit card fraud ranked #1 as the most common type of identity theft suffered by victims between 20 and 39 years of age.
As a top-five credit card in terms of active cardholders in the US, American Express has a vested interest in proactively detecting and eliminating credit card fraud. However, American Express must balance its fraud detection and prevention controls with the customer experience they provide to their cardholders. Simply put, they do not want to lose customers and disrupt customer business in order to root out fraud.
To improve the customer experience and reduce fraud risk, American Express has developed a “fraud model [that] is one of the most advanced in the industry,” said Dr. Dmitry Efimov, VP of Machine Learning Research at American Express, during a recent Emerj AI in Business podcast.
That model, called Gen X since it’s now a tenth-generation model, has grown to monitor and reduce fraud risk throughout American Express since the model’s first iteration in 2014. It’s also the biggest model within the card issuer’s operations, Dr. Efimov says.
AmEx built the model by first prioritizing the underlying business question the company wanted to answer, Dr. Efimov advises. With $1.2 trillion in worldwide billed revenue in 2019 alone, the model evaluates some 8 billion transactions every year and considers many factors within its algorithms, including whether the customer account has been victimized before.
The model also segments customers into different categories, e.g., frequent travelers, and considers that assignment when evaluating fraud risk. This segmentation, Dr. Efimov claims, allows AmEx to use a single model for both international and US markets, but still make fraud predictions for specific segments, unlike earlier models that required customers to be split into those segments before the models could be trained.
Although AmEx does not publish the list of red-flag fraud indicators sought by the Gen X model, the card issuer does disclose “certain behaviors [that] can indicate that a transaction has a higher risk of being fraudulent,” including customers that:
- Order the same item multiple times
- Are first-time shoppers
- Send purchases to postal codes or countries with high past incidents of fraud
- Purchase products that have high resale demand
The model’s algorithms assign weights to hundreds of fraud risk indicators and thousands of decision trees, which are subject to continual refinement based on comparisons between predictions and real-world observations.
AmEx strives to develop the science that will automate the ultimate decision that determines whether a transaction is fraudulent. Today, the company prioritizes reaching lower numbers of false positives and false negatives by pending questionable cases to human researchers.
Within AmEx’s credit and fraud risk group, its strategy department brings decision science to the outputs delivered by the fraud detection model. By balancing the probability that a transaction is fraudulent with the importance of protecting the customer experience, AmEx has arrived at thresholds that determine which red-flag transactions get pended for human review.
For American Express and its internal workflows, better fraud-detecting algorithms could mean maximizing the efficiency of their analysts’ time through missing less true fraud and pending fewer legitimate transactions for fraud review.
From a cardholder’s perspective, improved fraud-detection algorithms help provide more assurance that cardholders will be less likely to fall victim to credit card fraud while also being less likely to experience annoying delays or denials of legitimate transactions due to misplaced fraud suspicions.
AmEx aims to keep customers satisfied—and find fraud simultaneously. While our research did not identify detailed information about the instances of fraud that AmEx discovers through its AI-enhanced efforts, the company does claim that it has “maintained the lowest fraud rate among the major credit card networks for 14 consecutive years according to the February 2021 Nilson Report.”
Automating Customer Service Through NLP
Nine of every ten people assess a company’s customer service levels when they decide whether to keep doing business with that company, according to 2020 research published in Microsoft’s Global State of Customer Service report. And it costs five times more to convert a new customer than to keep an existing one, claims Invesp, a consulting firm based in Chicago and Istanbul.
Customer service matters to today’s businesses and American Express is no exception. The credit card issuer has been open in its efforts to integrate machine learning into its customer-facing functions, as the company’s AI director and VP of natural language processing and conversational AI told VentureBeat in October.
Dr. Di Xu, Vice President and Head of Information Management Decision Science and AI Labs at American Express, recently spoke at the second international symposium on Data Analytics, Risk, and Technology (DART), organized by the DART Lab in collaboration with RBCDSAI. Among the several AI deep learning use-cases he detailed was AmEx’s proprietary NLP-based customer service solution, NOVA.
For American Express, NOVA represents “NLP-based automation for customer servicing.” NOVA, the presentation claims, underpins many of the financial service firm’s customer service applications, including:
- Transcribing voice to text
- Processing travel bookings
- Automating customer service chat
- Enabling search in the AmEx mobile app
- Classifying emails for delivery to the right departments
For example, in processing travel itineraries, Dr. Di Xu explains, American Express relies on a BERT model to identify the customer’s intent—booking travel—and then another BERT model to extract their desired itinerary based on the words they use.
To overcome the challenges inherent in annotating the large amounts of data needed to train the NLP models, American Express has a dedicated 12-person team that labels and annotates the intents and name entities in the data, his presentation explains.
With the data prepared and the models ready, NOVA provides American Express’ customer service callers with NLP technology that supports the company’s automated customer service capabilities, whether they need travel bookings, a concern resolved, or need to find something in the Ask AmEx app.
The company has not lost sight of the need for human assistance in the AI technology it is pursuing, however. While American Express is “automating a large chunk of the queries” it receives, Madhurima Khandelwal, Head of AmEx AI Labs recently told Experian, AmEx works to integrate human-assisted AI, “for very complex queries, transitioning those seamlessly to live customer care professionals.”
While American Express does not share its internal customer service metrics or results, the company was recently ranked No. 1 in the J.D. Power Customer Satisfaction Study among credit card issuers in the United States, the tenth time American Express has achieved the designation in the last fourteen years. While American Express seems to perform well in customer service even without AI, the card issuer’s AI efforts seem geared toward maintaining this existing market edge into the future.