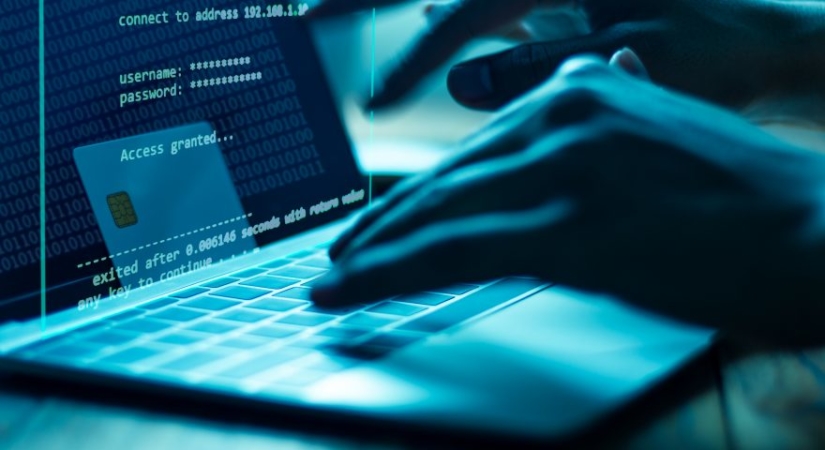
Leading retailers – like Walmart, Stop & Shop, and Home Depot – are enhancing their payment and fraud detection systems, using artificial intelligence that learns transaction norms and infers risk from the context of each transaction.
This article highlights two powerful AI use cases for retail fraud detection.
- Predictive Analytics – Analytics platforms for large-scale customers and transactional which can detect suspicious behavior correlated with past instances of fraud.
- Anomaly Detection – Applications for detecting deviations from normal payment activity compared to historical data over a time period which can be scaled differently for separate investigations.
Predictive Analytics
AI fraud detection services – aided by predictive analytics – can integrate with retail payment processing systems at the point of sale. The machine learning models empowering fraud detection services learn to spot patterns associated with fraud.
Examples of predictable fraud activities:
- Employee Theft – Discounts and write-offs at the point of sale can hide fraud. For example, irregular transaction patterns associated with certain item types or individuals could signal that a cashier is easing inventory out the door for friends, family, or a pseudonym. Trained algorithms can flag transactions matching high-risk patterns for review by management.
- Identity Theft – Transactions far away from normal shopping areas may signal fraud. Detailed customer profiles, enhanced by transaction geolocation data, empower identity theft protection services.
- False Returns – AI algorithms can learn to identify patterns using transaction data in real time for high-variance activities like false returns.
Anomaly Detection
Listing Unilever, Stop & Shop, and The Home Depot among its users, Profitect claims these firms deploy their prescriptive analytics services for fraud detection within their brick and mortar retail locations, via a tablet dashboard:
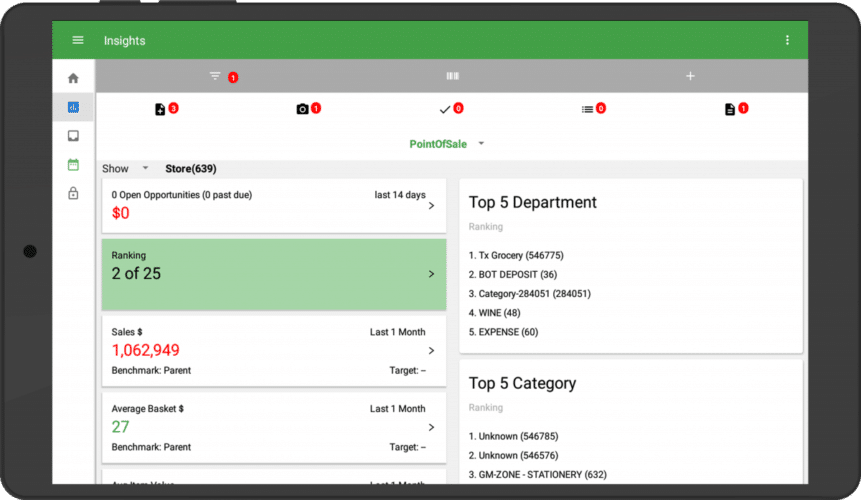
Prescriptive analytics systems recommend a “next best action” immediately following detection. They function especially well with a steady stream of fresh training data from recent transactions.
Benefits of in-store fraud detection include:
- Approval for Acceptable Deviations – Should John be allowed entry with his sister’s retail membership card? In real time, management may be notified of risky events, quickly accepting or denying actions upon review. Management decisions may also be fed back to improve the machine learning model.
- Awareness of Periodic and Daily Seasonality – AI models can learn the ways that normal patterns vary throughout the day/month/year, providing a baseline for defining adjustable risk parameters. For example, a retailer may want a higher level of protection against false returns during the holiday season.
This Anodot demo walks through use cases for anomaly review, research, and decisioning. Anodot claims to serve Lyft in detecting anomalous payments. Per this case study, significant losses were prevented, and detection times decreased:
Header image credit: Newconomy Media