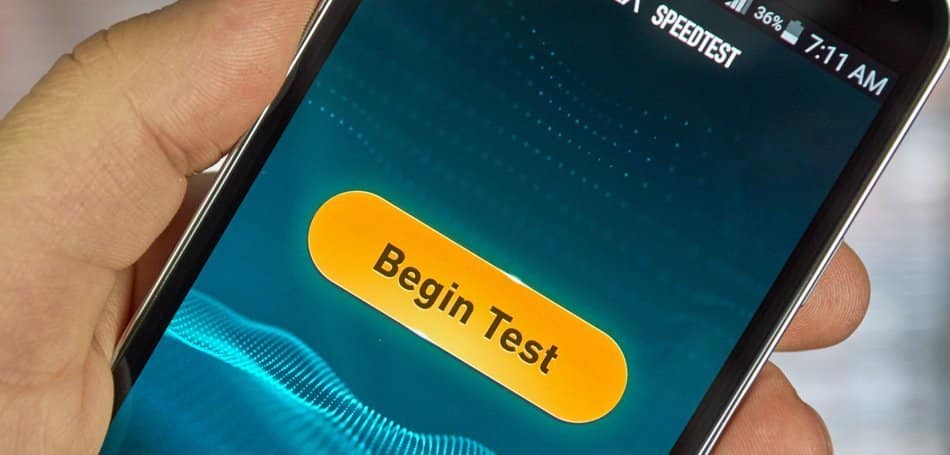
Before getting into this report, we have to inform readers that none of the companies discussed below claim to offer software that provides diagnostics, except Cognoa, which has FDA approval to call itself a diagnostic tool. We suspect this is because these companies are not legally allowed to do so. We usually don’t refer to a dictionary to determine what constitutes a concept, preferring to create our own informed definitions, such as in our What is Machine Learning? piece, but Merriam Webster defines “diagnosis” as the following: “the art or act of identifying a disease from its signs and symptoms.”
The companies listed in this report seem to provide diagnostics based on that definition, but again, readers should be informed that these companies do not technically provide diagnoses for illnesses and conditions, except Cognoa. Rather, they provide information to users on their symptoms (for legal reasons). Without further ado, let’s get into the report.
Reuters references an Orbis Research report estimating the size of the mobile health technology (mHealth) sector around $23 billion in 2017. It follows that AI would find its way into the mHealth sector as it has in the broader healthcare industry. As of now, numerous companies claim to offer consumers and hospitals mobile apps to help them diagnose illnesses and conditions in themselves and their family members as well as monitor their health.
We researched the space to better understand where AI comes into play in the mobile medical diagnostics sector or mHealth and to answer the following questions:
- What kinds of mobile devices are available for medical diagnostics and health monitoring?
- What tangible results has AI driven in the medtech sector?
- Are there any common trends among these innovation efforts? How could these trends affect the future of medical diagnostics?
This report covers companies offering software accessible to users through two channels:
- Smartphones and IoT Devices
- Chatbots
This article intends to provide business leaders in the medtech and healthcare spaces with an idea of what they can currently expect from Ai in their industry.
We hope that this article allows business leaders in medtech and healthcare to garner insights they can confidently relay to their executive teams so they can make informed decisions when thinking about AI adoption. At the very least, this article intends to act as a method of reducing the time business leaders in medtech and healthcare spend researching AI companies with whom they may (or may not) be interested in working.
Smartphones & IoT Devices
AliveCor’s Kardia App
AliveCor offers Kardia, an EKG application for Apple Watch and smartphones that the company claims allows Watch wears to monitor their own heart rates using what seems to be machine vision.
A sensor is installed underneath the Apple Watch which allows the Kardia app to shine a light on the wearer’s skin and feed visual information into the Kardia app’s machine learning model.
Specifically, the amount of light that is reflected back to the sensor as it scans the wearer’s skin is affected by the expansion and relaxation of the wearer’s arteries. This light data is then compared to similar data in AliveCor’s database that might be labeled as different instances of a heartbeat. In other words, the Kardia app can determine a Watch wearer’s heart rate based on how expanded their arteries are, information it obtains from its sensor.
In order for the Kardia app to work, customers must first purchase the Kardia Band on which the sensor is installed. They can then attach their Apple Watch to the band.
In addition to showing Watch wearers EKG information, the AliveCor claims the Kardia app tells wearers when their heart rate is too high or too low and if their heart rhythm is normal or abnormal.
Similarly, AliveCor offers the Kardia app for smartphones. The same technology that makes its EKG function possible on Apple Watch makes it possible on smartphones; however, customers instead purchase the sensor as a pad that can be attached to the back of their phones. This is demonstrated in the short video below:
According to a case study from Digital Health London, the patients of health providers affiliated with the National Health Service’s Innovation Accelerator in the UK had success with the Kardia app. While the case study did not provide specific results, it states that doctors have found that patient use of the application has led to quicker diagnoses and a patient’s further understanding of their condition.
Additionally, a study published in the Journal of Medical Internet Research found the Kardia app’s EKG measurements were consistent with the traditional EKG measurements of 88 randomly selected study participants. AliveCor also says they have a partnership with Mayo Clinic.
Frank Petterson is CTO of Alive Cor. He holds PhD in Computer Science from Stanford. Previously, he worked as a software engineer at Google.
Cognoa
Cognoa claims to offer employers a customizable platform which a company can brand and send to employees for childhood behavioral screening assistance. With the platform, an employee can log into a registration page with both the employer company and Cognoa’s logo.
Once they are on the website or Cognoa app, they can fill in information about their child’s health and behavior. Then, the Cognoa app sends the employee to screening results predicting the level of risk that their child has of developmental delays or developing autism. Cognoa claims this prediction is done through machine learning. The company’s machine learning model seems to be trained on data from both the app users and “labeled” clinical patient records; although, it is unclear how those patient records are labeled exactly.
Halim Abbas, Chief AI Officer at Cognoa, said this of the two sources from which Cognoa collects its data:
We might mine our user-base data to better understand the dimensions and/or segments that are most relevant to the problem at hand, and the nature of the (heavily non-linear) relationships and dependencies interconnecting the relevant dimensions. These insights would then influence the way we seek to collect, filter, and balance clinical patient records used for training our behavioral health screening models.
The 1-minute video below explains how a company employee can log in to the Cognoa website or app to get diagnostic information on their child’s risk for developmental delays:
It seems that the company’s work with employers is nascent. We could not find any case studies at this time in which the company reports success with its software. This could be because the FDA just recently allowed the company to begin promoting itself as a diagnostic tool. However, Cognoa lists SAP, a large product development company, as one of its clients.
Abbas was previously a senior research scientist at eBay and a principal data scientist at Teradata. He also holds an MS in Machine Learning and Artificial Intelligence from Columbia University.
Chatbots
Babylon Health
Babylon Heath offers a chatbot called Ask Babylon, which they claim can help consumers acquire information about their symptoms using natural language processing (NLP).
Babylon Health claims users can first type their symptoms into the chatbot. Then, the Ask Bablyon chatbot will ask a series of questions related to those symptoms in order to gather more specific information about them.
As the user answers these questions, the chatbot compares the answers to Babylon’s database of medical information—information that has been “collected and input by [Babylon’s] scientists and doctors,” according to the company. If the chatbot matches a user’s symptoms with information in Bablyon’s database, the chatbot then provides the user with information about the user’s symptoms. A user might then seek advice from a medical professional in the chatbot window.
If the chatbot does not find information for the user’s symptoms or the information it finds is particularly serious, the chatbot will recommend that the user either seek advice from their own doctor or consult with one of Babylon’s doctors on staff.
In addition, users can manually input their own medical information into Bablyon’s system in order to improve the chatbot’s recommendations for them.
Below is a short 2-minute video demonstrating how Ask Babylon works:
In a study published by Babylon in conjunction with the Imperial College of London’s School of Public Health, the Northeast Medical Group, Yale New Haven Health, and Stanford University School of Medicine, researchers conducted a scientific study in which human doctors and Babylon’s chatbot were provided vignettes detailing symptoms and medical histories.
The doctors and chatbot were then scored on how accurately they provided information about the correct illness from the symptoms and medical histories. The study found that the information Babylon’s chatbot gave were nearly just as accurate based on symptom-laden vignettes, and, in some cases, Babylon’s chatbot was more accurate than human doctors.
Babylon also lists Prudential Insurance as one of their past clients.
Mohammad Khodadadi, Babylon’s Director of Data and AI Cognition, holds a PhD in Computer Science from the University of Manchester. Previously, he was the CTO of Klappo Ltd and the co-founder of its AI-based venture, um.ai.
Healthy.io
Healthy.io claims to offer an FDA-approved home testing urine analysis kit that uses computer vision to scan and compare the colors of a test strip and to a result pad.
To use the product, called Dip.io, a consumer opens the app which will show them a chatbot interface. The chatbot will then give them instructions on the step by step process of using the product. First, the bot will prompt them to fill up a provided test cup with a sample of urine and wet the test strip.
Then the bot tells them to place the strip on a pad with a number of different color swatches. According to Healthy.io, each color on the swatch relates to a potential illness which could cause urine discoloration, such as a urinary tract infection, dehydration, or yeast infection.
From the chat panel, a user can open a camera-like scan screen and hold the phone over the swatch and strip. Even in poor lighting, the app claims to normalize the image, match the colors on the stick to the swatch, and identify illness possibilities.
Within moments of the scan, the chatbot gives the user the results that seem most likely based on the color match. It also gives the user advice, such as when and if they should seek medical attention.
We could not find a demonstration video showing how the user interacts with the chatbot.
According to the company, the app also offers a dashboard where users can see specifics of results and the presence of certain toxins that may be in their systems.
While Healthy.io does not make case studies or client listings available on site, a press release noted that they recently took on a clinical trial with Pennsylvania-based Geisinger, an affiliate of the National Kidney Foundation. Because kidney diseases and hypertension can be detected through urine samples, Geisinger noted that they believed this application and kit would help at-home consumers prevent and monitor kidney conditions.
The United Kingdom’s National Health Services Accelerator also notes that its affiliate doctors are encouraged to use or recommend the use of Healthy.io’s kit.
Healthy.io’s CTO, Ido Omer, holds both a PhD in Computer Vision from the Hebrew University of Jerusalem. The company’s algorithms developer, Igal Dmitriev, holds a Master of Science in Computer Engineering, Computer Vision Algorithms, and Image Processing from Ben-Gurion University of the Negev.
Takeaways for Business Leaders in Medtech and Healthcare
All of the companies covered in this report show evidence of AI and/or computer science talent on their leadership teams. This indicates that the applications detailed in this report are serious technological endeavors and not deceptive marketing crafted to secure venture funding.
As of now, many of the companies listed in this report are only beginning to find adoption in hospital and clinical settings. That said, we would expect these applications to see greater adoption from hospitals as a result of the talent behind them. While many of the applications still seem early on in their usage with hospitals, the UK’s National Health Service has been working on implementing diagnostic apps, such as those offered by Babylon, AliveCor, and Healthy.io, into their workflows.
The mobile health technology sector will likely continue to grow. That same Reuters-referenced Orbis Research report estimated that the mHealth sector would grow at a compound annual growth rate of over 35% over the next three years. This will likely be on the backs of PhDs offering products and software that clinicians and hospital systems can take seriously.
Header Image Credit: PhoneCheck