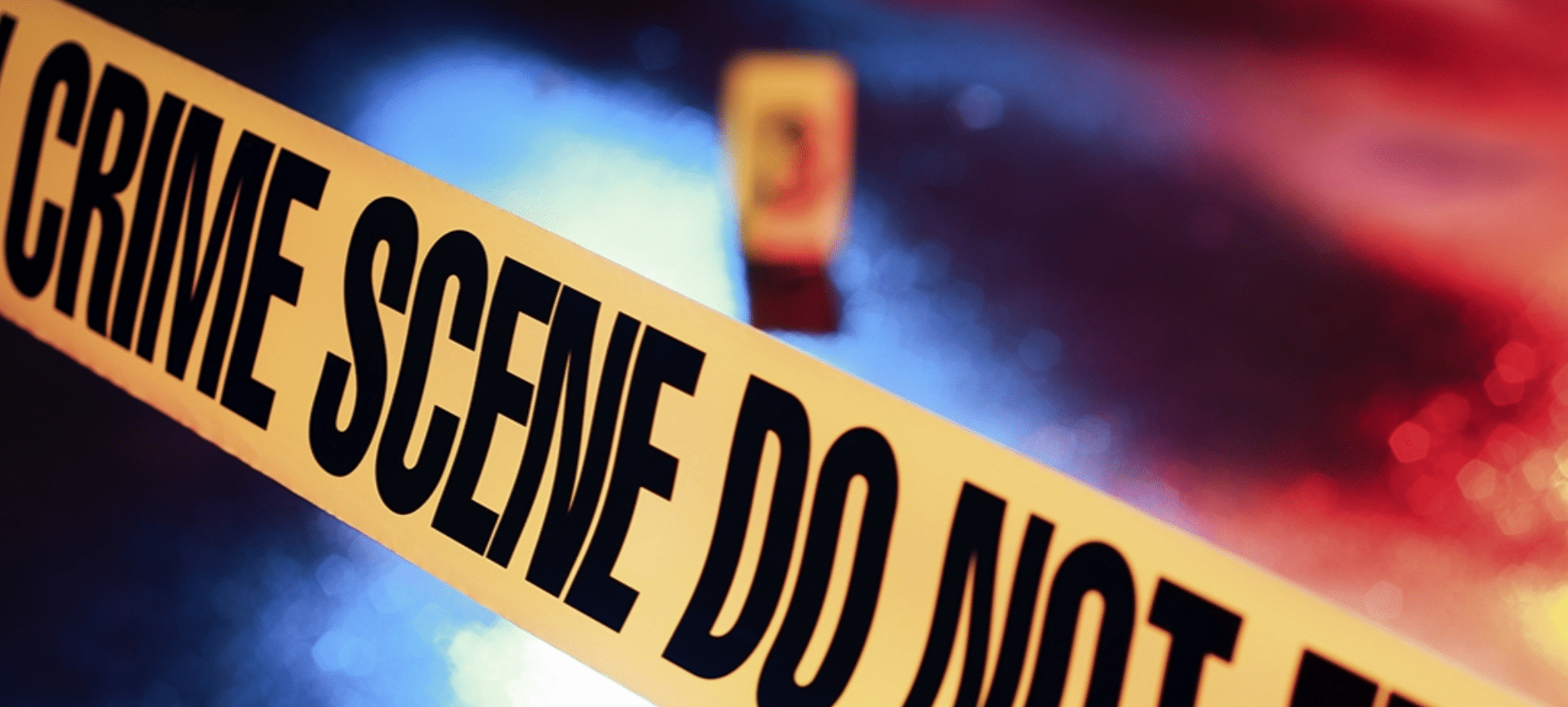
Companies and cities all over world are experimenting with using artificial intelligence to reduce and prevent crime, and to more quickly respond to crimes in progress. The ideas behind many of these projects is that crimes are relatively predictable; it just requires being able to sort through a massive volume of data to find patterns that are useful to law enforcement. This kind of data analysis was technologically impossible a few decades ago, but the hope is that recent developments in machine learning are up to the task.
There is good reason why companies and government are both interested in trying to use AI in this manner. As of 2010, the United States spent over $80 billion a year on incarations at the state, local, and federal levels. Estimates put the United States’ total spending on law enforcement at over $100 billion a year. Law enforcement and prisons make up a substantial percentage of local government budgets.
Direct government spending is only a small fraction of how crime economically impacts cities and individuals. Victims of crime can face medical bills. Additionally, high crime can reduce the value of property and force companies to spend more on security. And criminal records can significantly reduce an individual’s long-term employment prospects. University of Pennsylvania professor Aaron Chalfin did a review of the current research on the economic impact of crime and most analysis puts the cost at approximately 2% of gross domestic product in the United States.
This article will examine AI and machine learning applications in crime prevention. In the rest of the article below, we answer the following questions:
- What AI crime prevention technologies exist today?
- How are cities using these technologies currently?
- What results (if any) have AI crime prevention technologies had thus far?
Companies are attempting to use AI in a variety of ways to address crime that this article will break down into two general categories: (a) Ways AI is being used to detect crimes, and (b) Ways AI is being used to prevent future crimes.
Crime Detection
City infrastructure is becoming smarter and more connected. This provides cities with sources of real time information, ranging from traditional security cameras to smart lamps, which it can use to detect crimes as they happen. With the help of AI, the data collected can be used to detect gunfire and pinpoint where the gunshots came from. Below, we cover a range of present applications:
Gunfire Detection – ShotSpotter
The company ShotSpotter uses smart city infrastructure to triangulate the location of a gunshot, as they explain in this 3-minute video:
According to ShotSpotter, only about 20 percent of gunfire events are called in to 911 by individuals, and even when people do report the event they often can only provide vague or potentially inaccurate information. They claim their system can alert authorities in effectively real time with information about the type of gunfire and a location that can be as accurate as 10 feet. Multiple sensors pick up the sound of a gunshot and their machine learning algorithm triangulates where the shot happened by comparing data such as when each sensor heard the sound, the noise level, and how the should echoed of building.
They claim to be in use in over 90 cities including New York, Chicago, and San Diego. Most of their clients are in the United States, but last year they added Cape Town, South Africa to their list of customers.
The company had their IPO in July 2017, and their current market cap is $183 million.
AI Security Cameras – Hikvision
While ShotSpotter listens for crime, many other companies are using cameras to watch for it. Last year Hikvision, a Chinese company which is a major security camera producer, announced they would be using chips from Movidius (an Intel company) to create cameras able to run deep neural networks right on board.
They say the new camera can better scan for license plates on cars, run facial recognition to search for potential criminals or missing people, and automatically detect suspicious anomalies like unattended bags in crowded venues. Hikvision claims they can now achieve 99% accuracy with their advanced visual analytics applications.
With 21.4% of the market share for for CCTV and Video Surveillance Equipment worldwide Hikvision was the number supplier for video surveillance products and solution in 2016 according to IHS.
Movidius explains the benefits of having this capacity directly built into new cameras
Their systems have been using AI to perform tasks like facial recognition, license plate reading, and unattended bag detection for several years, but that video processing has traditionally taken place on a centralized hub or in the cloud. By performing the processing within the cameras themselves, they are making the process faster and cheaper. It can also reduce the need for using significant bandwidth since only relevant information needs to be transmitted.
Among the successes Hikvision cites is a 65% drop in crime in Sea Point, South Africa following the introduction of their cameras system.
AI for Crime Prevention
The goal of any society shouldn’t be to just catch criminals but to prevent crimes from happening in the first place, and in the examples below, we’ll explore how this might be achieved with artificial intelligence.
Predicting Future Crime Spots – Predpol
One company using big data and machine learning to try to predict when and where crime will take place is Predpol. They claim that by analyzing existing data on past crimes they can predict when and where new crimes are most likely to occur. Currently their system is being in several American cities including Los Angeles, which was an early adopter.
In this video Predpol co-founder explains how their system works.
Their algorithm is based around the observation that certain crime types tend to cluster in time and space. By using historical data and observing where recent crimes took place they claim they can predict where future crimes will likely happen. For example a rash of burglaries in one area could correlated with more burglaries in surrounding areas in the near future. They call this technique real-time epidemic-type aftershock sequence crime forecasting. Their system highlights possible hotspots on a map the police should consider patrolling more heavily.
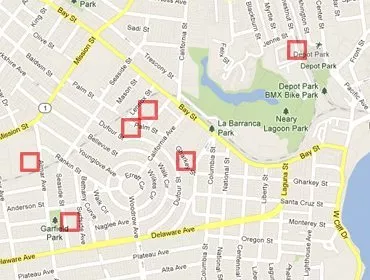
One success the company highlights is Tacoma, Washington, which saw a 22 percent drop in residential burglaries soon after adopting the system. Tacoma started using Predpol in 2013 and saw the drop in burglaries in 2015.
Given that crime is such a complex issue with numerous causes, it is very difficult to isolate the impact any one tool has. However, one study by researchers at Predpol concluded that police patrols based on near real-time epidemic-type aftershock sequence crime forecasting (what Predpol uses) results in a 7.4% reduction in crime volume.
Predicting Who Will Commit a Crime – Cloud Walk
The Chinese facial recognition company Cloud Walk Technology is trying to actually predict if an individual will commit a crime before it happens. The company plans to us facial recognition and gait analysis technology help the government use advanced AI to find and track individuals.
The system will detect if there are any suspicious changes in their behavior or unusual movements. For example if an individual seems to be walking back and forth in a certain area over and over indicating they might be a pickpocket or casing the area for a future crime. It will also track individual over time.
The company told the FT, “Of course, if someone buys a kitchen knife that’s OK, but if the person also buys a sack and a hammer later, that person is becoming suspicious.”
Pretrial Release and Parole – Hart and COMPAS
After being charged with a crime, most individuals are released until they actually stand trial. In the past deciding who should be released pretrial or what an individual’s bail should be set at is mainly now done by judges using their best judgement. In just a few minutes, judges had to attempt to determine if someone is a flight risk, a serious danger to society, or at risk to harm a witness if released. It is an imperfect system open to bias.
The city of Durham, in the United Kingdom, is using AI to improve on the current system deciding to release a suspect. The program, Harm Assessment Risk Tool (Hart), was fed five years worth of criminal data. Hart uses that body of data to predict if an individual is a low, medium or high risk.
The city has been testing the system since 2013 and comparing it’s estimates to real world results. The city claims Hart’s predictions that an individual would be low risk were accurate 98 percent of the time, and predictions that an individual would be high risk were accurate 88 percent.
The intent is for Hart to advise authorities on which suspects are most likely to commit another crime.
Jurisdictions in the United States have been using more basic risk assessment algorithms for over a decade to make decisions about pretrial release and whether or not to give an individual parole. One of the most popular is Correctional Offender Management Profiling for Alternative Sanctions (COMPAS) from Equivant, which is used throughout all Wisconsin and numerous other locations. A 2012 analysis by the New York Division of Criminal Justice Services found COMPAS’s, “ Recidivism Scale worked effectively and achieved satisfactory predictive accuracy.”
COMPAS has recently come under fire after a ProPublica investigation. The media organization’s analysis indicated the system might indirectly contain a strong racial bias. They found, “[T]hat black defendants who did not recidivate over a two-year period were nearly twice as likely to be misclassified as higher risk compared to their white counterparts (45 percent vs. 23 percent).”
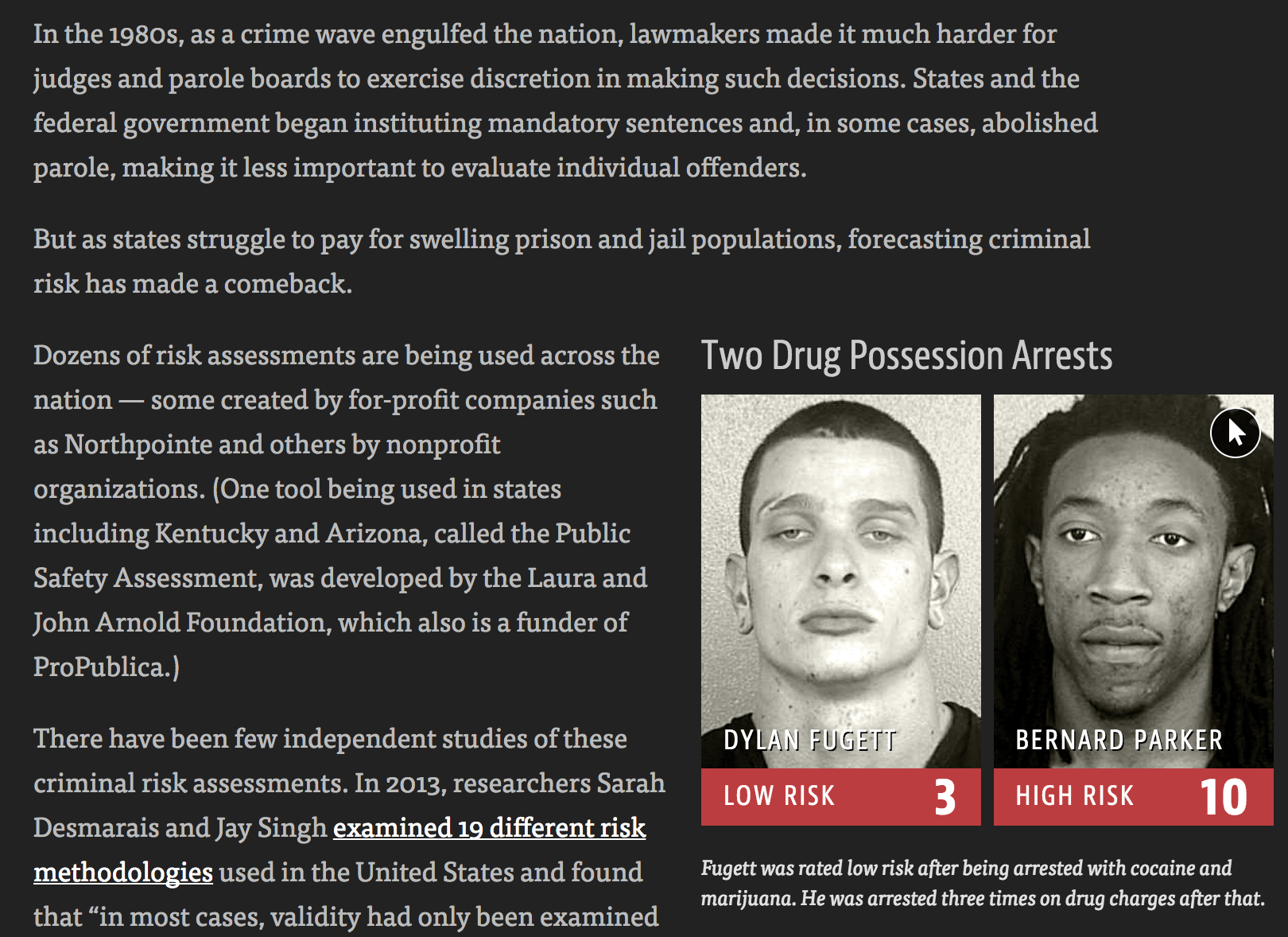
The report raises the question of whether better AI can eventually produce more accurate predictions or if it would reinforce existing problems. Any system will be based off of real world data, but if the real world data is generated by biased police officers, it can make the AI biased.
Concluding Thoughts and Future Outlook
The ability of AI to allow governments to collect, track, and analyze data for the purpose of policing does raise some serious questions about privacy and the threat that machine learning could create a feedback loop that reinforces institutional bias. This article wasn’t dedicated to these important issues but the AI Now Institute at New York University is a research center dedicated to understanding the social implications of artificial intelligence which can provide more details about these concerns.
While civil liberty concerns do exist, they so far have not stopped the spread of AI technology in surveillance and crime prediction. According to IHS, there were 245 million professionally installed video surveillance cameras operating in 2014 and the number of security cameras in North American effectively doubled from 2012 to 2016. There is more and more data being fed to security and law enforcement agencies; it is only natural they are going to want to keep investing in more and more AI tools to shift through this ever-growing stream of data.
The use of AI and machine learning to detect crime via sound or cameras currently exists, is proven to work, and expected to continue to expand. The use of AI in predicting crimes or an individual’s likelihood for committing a crime has promise but is still more of an unknown. The biggest challenge will probably be “proving” to politicians that it works. When a system is designed to stop something from happening, it is difficult to prove the negative.
Companies that are directly involved in providing governments with AI tools to monitor areas or predict crime will likely benefit from a positive feedback loop. Improvements in crime prevention technology will likely spur increased total spending on these technology.
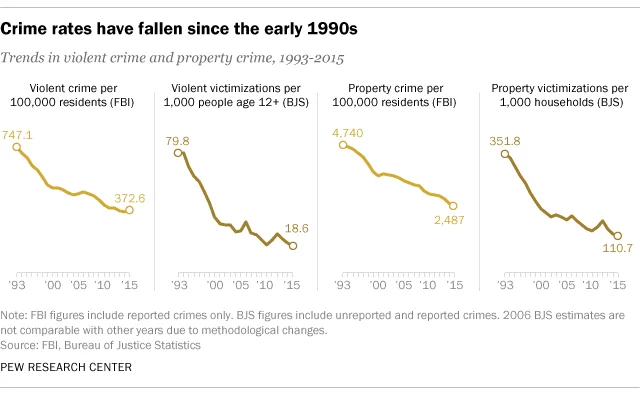
While effectively all categories of crime have been trending down for decades, in major American the share of general funds being spent on law enforcement has grown steadily. In American politics, there remains strong emphasis on law enforcement. It seems that the drop in crime has possibly even created a feedback loop. Instead of a lower crime rate being seen as a reason to cut police services, it is seen as proof that law enforcement is working so therefore deserves more money.
After all, a lower crime rate has broad social benefits for a community and real political benefit for the local elected officials responsible for budgeting. In New York City both liberal mayors like Bill De Blasio and conservative mayors like Rudy Giuliani heavily citing the drop in crime under their tenure during their re-election campaigns.
Most of these technologies which are or were mainly developed with government clients in mind have spillover benefits for private companies. The same AI security cameras used by government are also being used by private companies to protect their assets. Technology used to predict crime or automatically catch suspicious behavior can help companies with loss prevention or deciding where establish new locations.
Header image credit: Slate