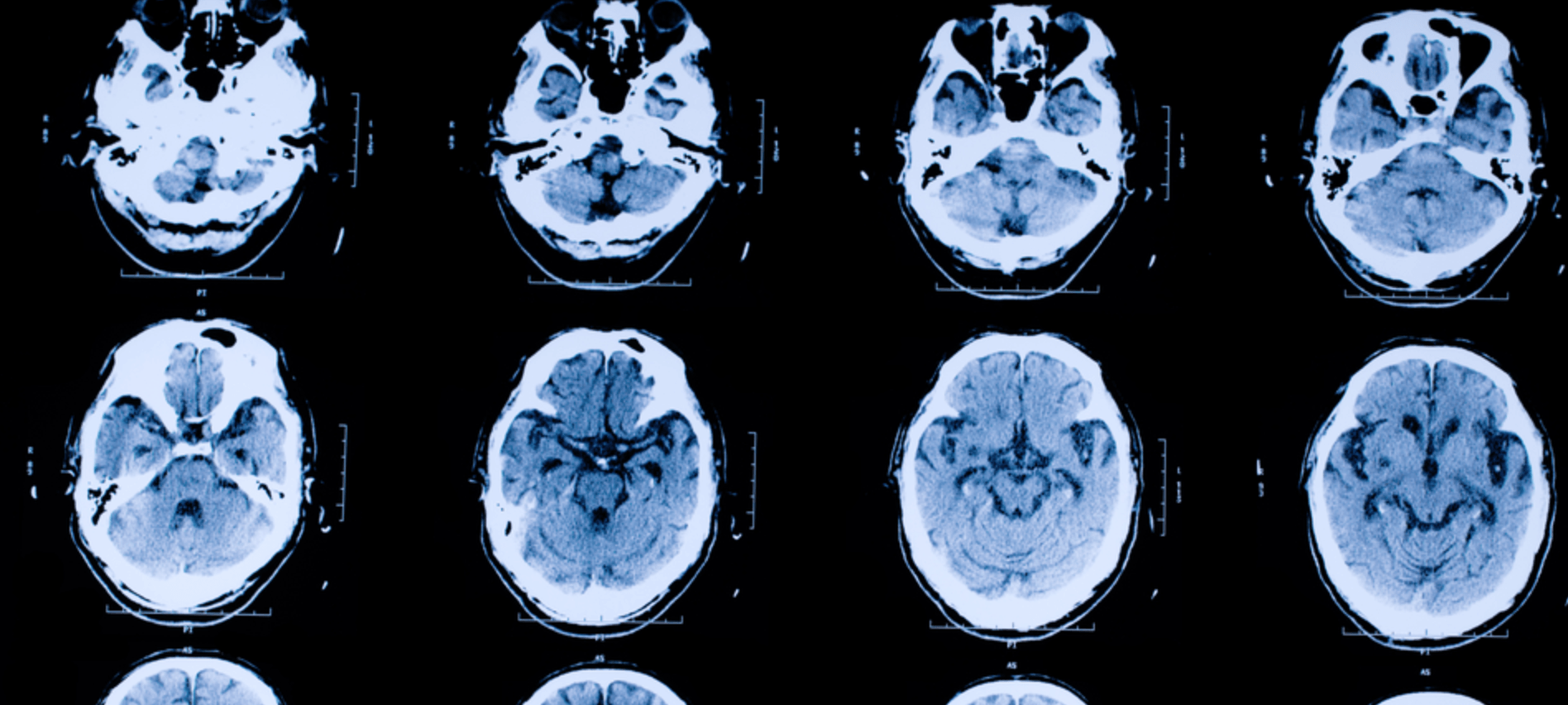
Episode Summary: This week’s episode covers the medical applications of machine vision for the diagnosis and treatment of cancer. Medical science has integrated AI since the late 90s, and it’s been useful in the fight against cancer. This week’s guest is Dr. Alexandre Le Bouthillier, founder of Imagia. Imagia is a medical imaging company which specializes in using AI and machine learning to detect cancer in its early stages so that oncologists can make quicker, more accurate diagnoses for patients.
AI is a useful tool in the detection of breast cancer, colon cancer, and lung cancer. It can even detect genetic mutations, something humans certainly cannot. Learn just how important AI has been over the last two decades in developing the medical infrastructure necessary for patients to have a chance at surviving and even curing their cancer.
Subscribe to our AI in Industry Podcast with your favorite podcast service:
Guest: Dr. Alexandre Le Bouthillier is the current COO and founder of Imagia
Expertise: Medical artificial intelligence, Operations Research
Brief Recognition: Dr. Alexandre Le Bouthillier is the current COO and founder of Imagia, a medical imaging company specializing in the use of AI and deep learning for the detection of cancer. He is also the co-founder of Planora, a software company which provides services for solving complex problems with regards to the allocation of human and material resources. He sold the company in 2012 to RedPrairie. He dedicates most of his time and resources to ventures in the fields of machine learning, operations research, the human brain, finance, and big data.
Current Affiliations: COO and founder of Imagia, co-founder of Planora
Big Ideas on the Future of Medical Machine Vision
AI and machine learning are absolutely essential to medical science in today’s world. Making sense of scans and other kinds of medical imaging would be near impossible for a human specifically due to the sheer volume of images that a typical imaging procedure may produce. For example, a virtual colonoscopy can produce thousands of images that, without AI, a medical professional would need to sift through manually to find an image worthy of further scrutiny in order to detect a possible problem. After it’s recognized the pattern in images that indicate, say, colon cancer, AI can fill the role of the medical professional here. It can quickly search through those thousands of colonoscopy images to find those worthy of further examination so that the patient can receive a diagnosis in little time.
In the last few years, AI has become so sophisticated in this field that it’s oftentimes no longer necessary to code a machine to search for specific images. Through deep learning and pattern recognition processes, the software can find these images on its own when presented with similar images, thus reducing the time a medical professional is spent “training” the machine. This is extremely useful for radiologists and oncologists with a large caseload.
The AI can then go on to detect subtleties in these images that can reveal patterns humans wouldn’t ordinarily be able to figure out. As a result of breakthroughs in deep learning, AI can correlate the subtle features of medical images hidden to human scrutiny with patient diagnoses. As a result, medical professionals discover patterns that can influence which images they choose to focus when the AI selects it for them out of thousands. In other words, medical professionals learn from AI which images are cause for concern. This of course then translates to earlier diagnoses for patients, which can greatly increase their chances of survival and cure.
[tecarousel]
Interview Highlights with Dr. Alexandre Le Bouthillier
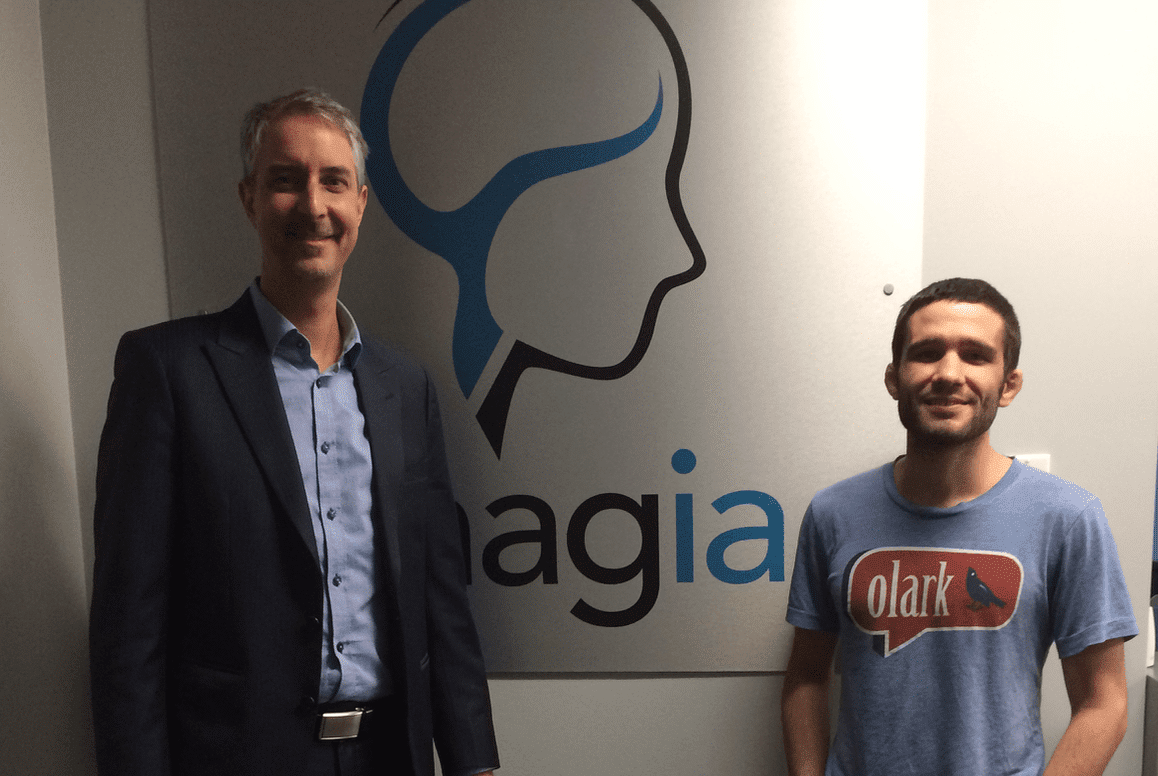
Daniel Faggella (3:12): What is possible today with regards to machine learning and medical imaging?
Alexandre Le Bouthillier: With these computer aided diagnostics techniques, deep learning is now able to present information so that you don’t have to explain in code to the machine what to look for.
With this technology, were are more easily able to tackle more difficult identification, segmentation, or even classification problems for cancers that are more subtle and harder to detect.
Dan (5:50): You mention that since the late 1990’s, AI has played some role in oncology. My assumption is that was more [an approach involving] hard-coded descriptions of what a system should be looking for in terms of imaging. Is that safe to say, or was there some degree of machine learning even back in the 90’s?
AL: Yes that was the neural network that we had at the time, but people who were doing AI really needed the knowledge to explain to the machine what image features were important. For example, the code will explain if an image found has homogeneity in texture or [it will explain] the brightness of an object.
With that you could see hundreds of these features and come up with a certain percentage of accuracy for detecting and identifying different types of lesions for breast cancer, for example. But you’re limited by human imagination and also the knowledge of what a particular type of cancer looks like. So the revolution in deep learning is, rather than explaining in code what to look for, you stack neural networks together. So, when you show an example to the layers, the layers will figure out the right answer to the questions.
When you show a second example, the configuration to provide the same answer would be slightly different, and the way that those layers are connected will change to minimize the chances of producing the wrong answers. The more images and examples you give the program, the better it can function and produce more and more accurate images.
Dan (8:47): We can think of a pattern that appears to constantly repeat itself as something that we as humans are able to detect and describe and those are the things that we can hard-code. But really there are all kinds of subtle patterns that machines are able to pick up that humans cannot even articulate. Is That accurate?
AL: It’s very difficult for humans to understand fractal representations, but this is something that machines are capable of doing. So when an oncologist looks at a series of images and they want to represent how statistically those pixels have evolved over time, this is a task that is difficult for humans to do. This is when machines comes in to help the radiologists and oncologists as a decision support tool.
People are really looking for quantitative measures. Right now, they’ve been using those systems to measure distance, progression, or regression of the tumor, and that was the way that the systems was designed: to look at what’s happening. [Through deep learning, AI] is able to take thousands of features and correlate those features with patient outcomes, even with regards to genetic mutation. Machines are able to detect things such as genetic mutation.
Dan (13:18): How pervasive is machine learning in the healthcare industry?
AL: So, for breast cancer, [medical professionals] use machine learning programs for imaging. Outside of imaging, there are other companies that do speech-to-text, which is widely used by radiologists. When they speak to a computer, the machines listens to those words and types it for them. The accuracy of these programs has increased significantly even in the last two years.
Dan (15:15): Are there any other kinds of cancer where it’s not uncommon to use AI?
AL: When we look at the screening programs, what’s interesting about these programs is that if you can catch a cancer earlier (stage 1 or stage 2), patients can survive and even be cured. So there’s a strong interest to have those screening programs in place.
In most countries, you will see screening programs for breast cancer, lung cancer, and also colorectal cancers where there are a lot of screening programs in place. There’s a presence of AI in virtual colonoscopy where, instead of taking an endoscope, you can take an image of a polyp to see if there’s any sign of cancer. AI is very present in cancer screening.
Dan (16:37): Is this something you can find easily in hospitals and cancer centers around the world?
AL: Yes, because otherwise there’s too many images to look through when you inspect a colon manually. Most of the time doctors use [AI] to go through the images, and that’s the challenge. It becomes too time consuming for the radiologist to look at all of those images [produced by the machine]. They want to focus on the images that can help make diagnoses, and machine learning can help with that.
Dan (18:30): Can you speak a bit to what breakthroughs in the coming five years are going to help on the predictive side and what that will allow?
AL: The breakthrough actually occurred a few years ago with deep learning with regards to what you can see with patient outcomes and even genetic mutations. Just by looking at an image, the computer can see if there’s any genetic mutations, so we wouldn’t need to do a blood sample or biopsy. The ultimate end goal would be to, just by looking at an image, be able to accelerate the standard of care. By combining all of the available data, we will be able to find the best treatment for each patient.
Subscribe to our AI in Industry Podcast with your favorite podcast service:
Header image credit: MS Society of the UK