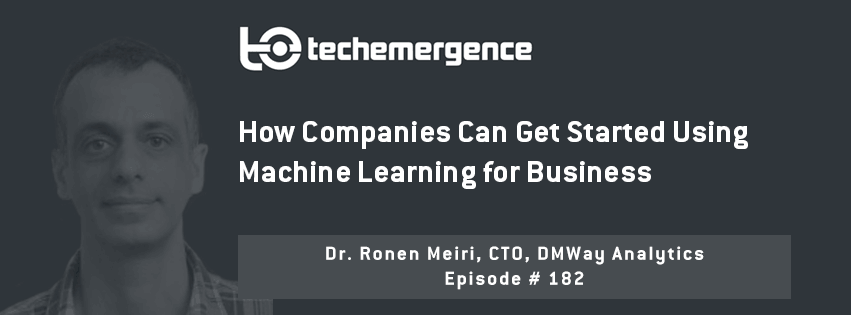
Episode Summary: Predictive analytics and machine learning are all the rage in Silicon Valley, but how do companies actually derive value by leveraging these technologies? We asked this question to Dr. Ronen Meiri, CTO and Founder of DMWay, a predictive analytics and machine learning platform company based in Israel. In this episode, Ronen speaks about what his company does and how smart executives are starting to make decisions on how to choose and decide on a smart, user-friendly platform that fits their business’ needs.
Expertise: Data mining and predictive analytics
Brief Bio: With more than 10 years of experience in data mining, developing algorithms & models for marketing, behavioral targeting, mobile, and fraud, Ronen is in charge of DMWay’s predictive analytics platform. Prior to DMWay, Meiri was CEO of Chi Square Systems LTD, which develops and uses data mining algorithms for improving business performance. He also served as a project manager at Mivtach-Simon, a product manager at Octavian and Qware, and a lecturer at Tel Aviv University. Meiri received his bachelor’s and master’s degrees in Physics from Tel Aviv University, as well as an MBA and a PhD in Operational Research.
Current Affiliations: CTO and Founder of DMWay
Interview Highlights:
(1:43) When you see companies do this right, really set their ducks in a row properly before implementing ML, what do smart companies do before they bring in a ML solution?
(3:52) Getting the business problem clear, it sounds like that means not just saying, ‘hey we need to figure out customer churn’, but ‘how do you define that?’….are you talking about making the business problem clear so that everyone on the team knows what we’re trying to get at?
(7:10) What are other examples here, what does this really imply, what is the nuts and bolts of the meaning of “cleaning the data” when you really go into build a robust machine learning application?
(10:05) In your experience…what have been some examples of generally lower hanging fruit for companies, places where so long as you have the information and business problem right, this particular thing is something that you can probably gain value from…are there any examples across industry that are easy wins compared to more complicated applications?
(12:48) It’s probably quite different per company in terms of what you’re doing with customer lifetime value, or are there some similarities there…have you seen patterns?
(15:00) Right now there are companies aiming to implement predictive analytics, aiming to implement machine learning…there is no real easy comparison of the options available to them, there is no Gartner report comparing the various solutions…how are companies kind of gauging who’s the right person to go with, what is the decision processes they’re going through?
(18:28) How are they going about making education decisions between yours or other companies in machine learning and predictive analytics…what kind of benchmarking are people doing?
Related Emerging Technology Interviews/Articles: