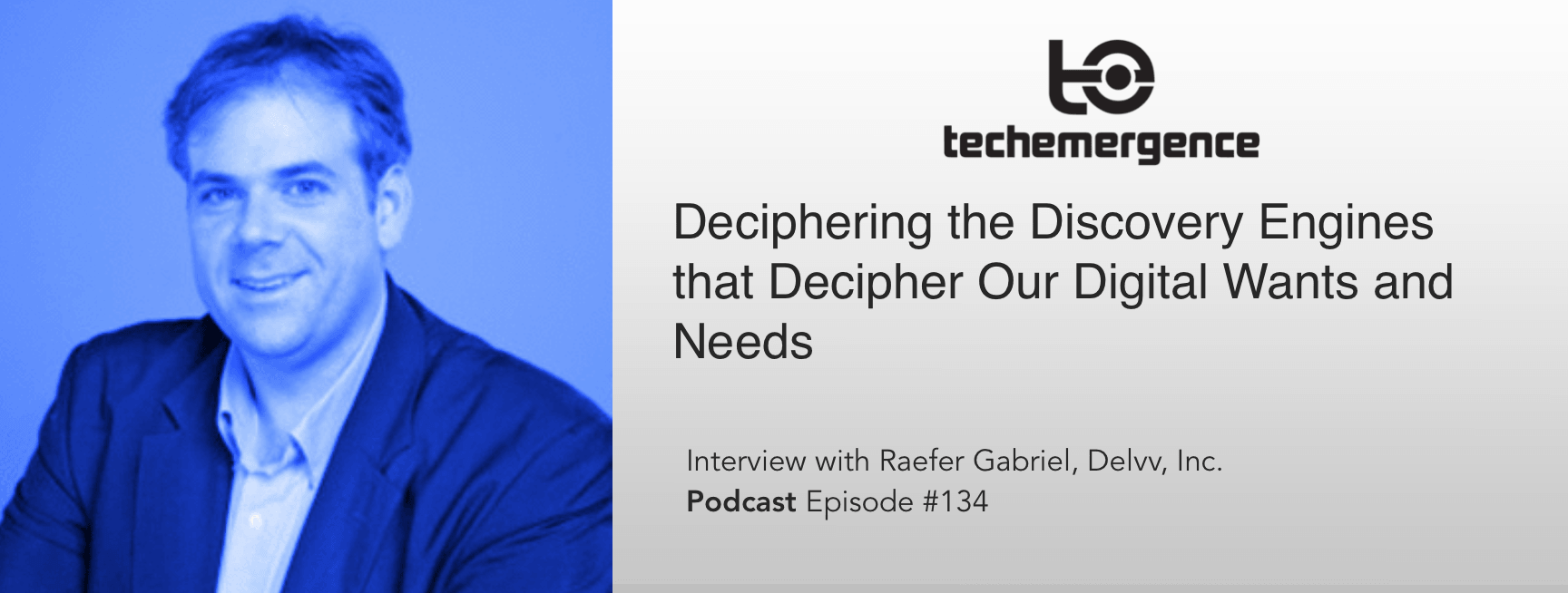
Episode Summary: Ever had the perfect book recommended to you by Amazon or gave a pleasantly-surprised thumbs up for a song selected for you by Pandora? Both services are powered by recommendation engines, which are gaining steam int he commercial space. In this episode, we speak with Entrepreneur Raefer Gabriel, who works for Delvv on the commercial applications of recommendation engines. We talk about how this technology works, and how it comes to learn from reviews, ratings, and consumer interactions. Gabriel also gives perspective on how these engines might be enhanced and applied in the future, a good topic for those of you in the startup world.
Guest: Raefer Gabriel
Expertise: Software Development and Predictive Analytics
Recognition in Brief: After receiving his AB in Physics at Harvard and an MBA from Columbia Business School (where he was an inaugural recipient of the Feldberg Fellowship), Raefer launched his career as a technology entrepreneur, specializing in solving the hard problems of how people relate to technology. He founded Reputation.com, a software for monitoring personal information and reputation on the Internet. He also founded TruExchange, a venture-backed software company building statistical arbitrage trading system, which was acquired by the New York Mercantile Exchange in 2003. In 2013, he co-founded Delvv, Inc. with the goal of decluttering people’s digital lives through machine learning technology.
Current Affiliations: Delvv, Inc.; Principal at Alaricus Capital
More Data, Smarter Recommendations
When it comes to discovery engines, what does the public really know about them? Netflix is a service powered by a discovery engine (also called a recommendation system) to which many people can quickly relate, says Delvv’s Raefer Gabriel. Even if the person is not a subscriber, he or she has likely seen movies being recommended, a tool powered by a content discovery recommendation engine. Spotify and Pandora are also popular services powered by similar discovery engines.
What do Netflix, Spotify, and Pandora have in common? They’re examples of more constrained domains (visual media and music) that have shown a lot of success at applying recommendation system techniques toward content discovery, while some of the more unbounded domains, like recommendations about general web content, have struggled a bit to make good use of the technology available. “It’s hard to represent well and match well with other kinds of things people are consuming,” says Gabriel.
It’s not just consumers engaging with these services but how they engage that counts. Constrained domains allow for more accurate recommendations. Conversely, when making recommendations for general web content, there’s lots of dispersed material of which to make heads or tails. I asked Gabriel if Amazon might be a good example of an almost unbounded category that tries to make sense of more diverse data sets.
Gabriel agrees that Amazon has more types of recommendations in one interface than he’s seen another company do, and it’s made possible by different types of collaborative filtering; a more recent and common one, for example, is a recommendation correlated between people who bought a particular product and other consumers who bought the same and other products. Of course, Amazon has the funds and the large swaths of data to put into these efforts. In an extension of its web services, Amazon has even released recommendation engine software that anyone can use, as detailed in this Wired article by Cade Metz.
Most of the collaborative filtering algorithms built around these techniques tend to work better if you bound the domain in some way, says Raefer; it’s really about how many different products a service is trying to compare at one time, which is built into a matrix that represents user preferences and inferences to be made. Amazon does something like this, but they do both item-to-item collaborative filtering within product categories, as well as in a broader consumer category.
In many cases, if an individual is a sporadic or infrequent consumer or a product is new, you may only have small sets of data; however, the whole key to coming out with good results is more about the volume of data that is fed into an algorithm. “Once you get to a certain critical mass of data about a user’s preference, it definitely becomes a lot easier to recommend good stuff for them that’s similar to what they’ve bought in the past,” says Gabriel. Discovery engines have to be able to extract something from the first click for new clients, but this process refines itself over time and with more use.
The point of intersection between data warehousing and machine learning problems is critical, because machine learning doesn’t do much without data. There is a definite need for good systems to collect data, manage the flow of data, and then apply models to test the scale of data in production. This is one of the strengths of Google’s TensorFlow open source software, which focuses on data flow modeling. There are now lots of open source and commercial packages out in the public sphere, and how companies periodically retrain a system is reflected strongly in new systems, which Gabriel says is “key to building real systems in real world that work well around recommendation systems, collaborative filtering, and other machine learning algorithms.”
The Frontier of Personal and Predictive Engines
In the coming five or 10 years, how might continued development of discovery engines permit us to interact differently with our technology? The field of big data analysis and AI learning is moving fast (as discussed by Brad Power in this Harvard Business Review article).
Gabriel thinks that the current wave of big data analytics and led to more usage of content-based ads. “It’s hard to find a news website that doesn’t feature sponsored results from Tabula or another sponsored content company.” Content marketing companies are using collaborative filtering algorithms when they have more limited domains of content to draw from, but they’re also using cookie tracking to see the kinds of websites that a person has visited in the past, which helps narrow down targeted ad options.
On the flip side of all this targeting, says Gabriel, is that key phrases like, “we found some other things you might be interested in…”, have become closely associated with advertising. “These phrases that might mean something real in the world of recommendation systems are now becoming, here’s something that someone paid to stick in your face…it can leave a bit of a bitter taste if it becomes cliche and misleading.” Raefer calls this “the grey market of recommendation”, the kind we don’ t like to talk about because it’s currently being used a lot in advertising.
Might our future overt searches also be augmented by these types of engines? Delvv’s vision statement is encapsulated in the slogan, “search less, know more”. Could machines come close to reading our minds, discerning our intention more quickly?
Gabriel says that there is a lot of effort in this direction. “Google, at certain points, has presented the Google Now platform to push predictive analytics ahead of the search problem, to be there with the search result before you even get to type it in,” he says. We might think of this burgeoning area of technology as similar to the division of “pre-crime”, except in this case we might dub this intersection of search and recommendation as “pre-search.” Only time will tell if future search engines are able to decipher what we want before we even finish the thoughts in our own head.
Image credit: Delvv, Inc.