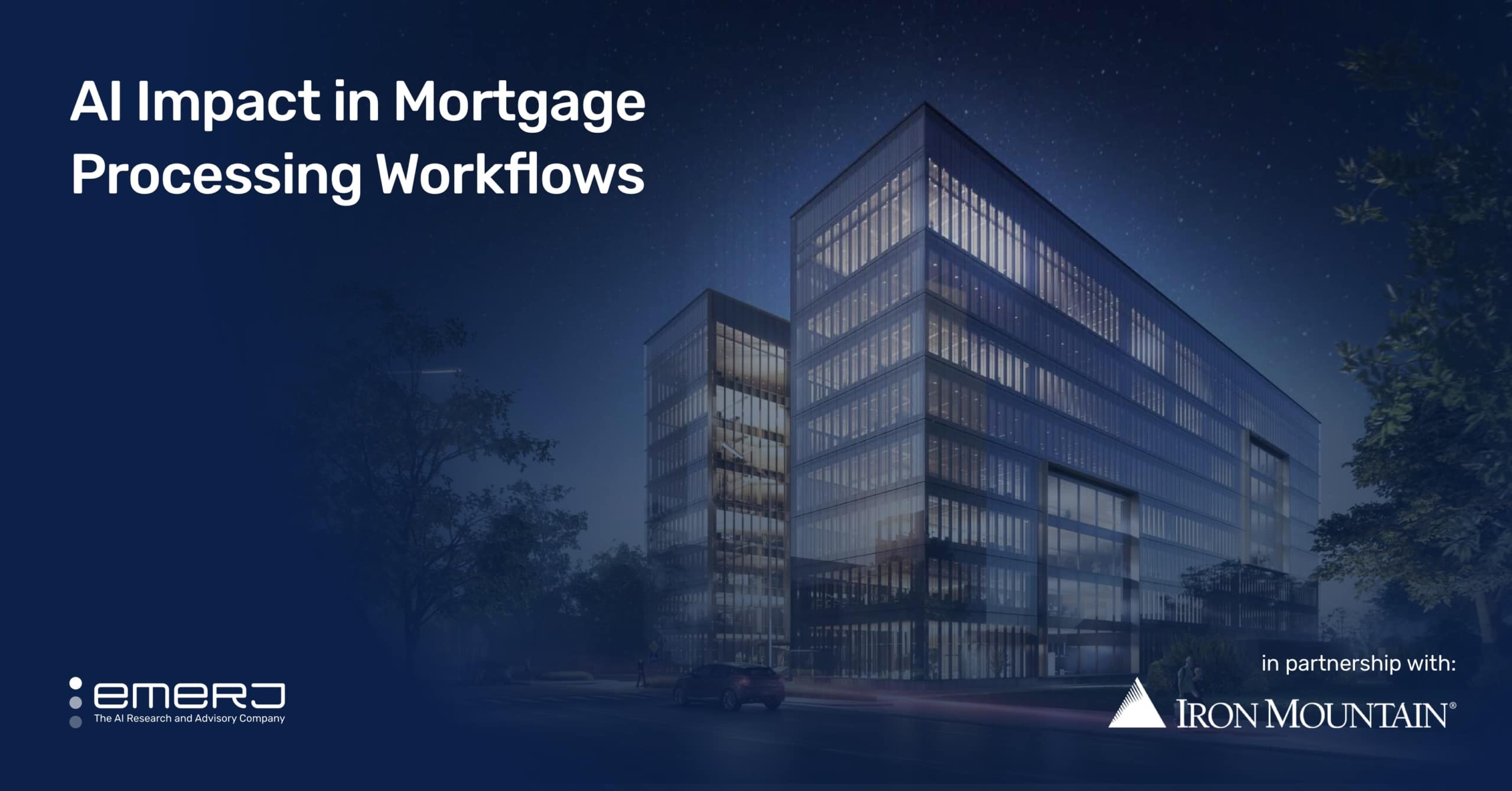
This article has been sponsored by Iron Mountain, and was written, edited, and published in alignment with Emerj’s sponsored content guidelines.
Buying a house is often one of the most significant financial investments of one’s life – and paperwork involved in the mortgage process is notoriously intense. Deeds, agreements, terms – verified by various parties.
Why hasn’t technology made the process easier?
Hopefully, AI can help.
Today’s guest is a veteran of the mortgage technology space. Kristopher Lorang is Financial Services Product Manager at Iron Mountain, a publicly-traded international services firm. Iron Mountain is a leader in secure storage, which now includes digital record storage and management. In this episode, Kris shares some of the use-cases for AI-based document search and discovery in the complicated (and paperwork-heavy) mortgage process. Listen to the full episode or read the transcript below.
Subscribe to our AI in Business Podcast with your favorite podcast service:
Guest: Kristopher (Kris) Lorang, Product Manager of Financial Services at Iron Mountain
Expertise: Mortgage processes and automation
Brief Recognition: Before joining Iron Mountain’s Insight product group, Kris held a position as VP of Mortgage Technology at BNY Mellon, the 8th largest bank in the United States by total assets. Kris graduated with a Master’s degree in Industrial Management from Northern Illinois University.
Key Insights
- Comparisons and verification could be low-hanging fruit for AI systems. Ensuring that documents have the same name, address, loan amount, or terms across various documents could be the role of AI systems. Computer vision and natural language processing can find matches or mismatches and notify workers, instead of relying entirely on human scanning. These systems might save humans time for more complex tasks, or might serve to flag potential mismatches that workers may have failed to notice.
- Automation and streamlining is more important when demand fluctuates. It’s hard to change the headcount in a mortgage operation with the up and down volumes of mortgages to process. Seasons, interest rates, and a variety of other factors can shift mortgage volumes, leaving teams either overstaffed or overwhelmed. AI systems handling some of the processing and workflows might help even out the headcount requirements of mortgage firms, and allow team members to focus on more cognitive and creative tasks – rather than rote processes and verification.
Full Interview Transcript
Daniel Faggella: So Kris, today, we’re going to be talking about some of the opportunities for artificial intelligence in the mortgage process. Obviously, one of the more paperwork, heavy processes in the financial services space, or notoriously so.
Before we get into AI, what I like to do is kind of paint the picture of what the process is now. And then we talk about those junctures where AI can layer value. Can you let us know, after a mortgage closes, because I know that’s what you’re most familiar with there in Iron Mountain, what has to happen in terms of paperwork and processes in the backend of the institution?
Kris Lorang: Sure. So as many of the listeners probably know, a lot of the front end of the process in mortgage is heavily automated today. You can get a mortgage on your phone, you can upload documents on your phone, your computer, etc. But when it gets to the closing process, as you mentioned, everything essentially papers out, meaning you have to print all of the documents and then you have to go in sometimes to an office and sign those documents with ink signatures. And then those papers have to be sent back to the lender.
Kris Lorang: Now, of course, in this pandemic environment, a lot of these processes have been altered. A lot of notaries will come to your hous so you can ink sign things. I mean, there’s even a little bit of remote online notarization, that sort of thing. But for the most part, a lot of mortgages are still closed using wet-ink signatures on paper documents.
So really that process, once it gets back to the bank, it enters what they call a post-closing team or department. In that department, they have to look at each of the individual documents to make sure all of the data points are accurate to make sure, for example, the rate on the note agrees with the system and the rate it was locked at. It has to make sure that everyone signed every document, that it was notarized in all the right places. Every document is present that is expected as it reaches the investor, they expect certain documents. So it’s really just a lot of manual intensive labor and a lot of stare and compare activities. So they’re staring at their screen, verifying data that appears on the document.
Daniel Faggella: “Stare and compare.”
Kris Lorang: Yes.
Daniel Faggella: So the bulk of this is really just in-depth fact checking for an unfortunately still paper ecosystem, wonder how long it’ll take for it to not be that way? But it sounds like that’s a big part of it. You mentioned, do the rates match, did people sign things properly, is there anything else that’s involved in this stare and compare? I imagine somebody just, a individual human being with a visor on and a lamp just pulling out these to go on to page 20, going on to page 18, making sure these things match, going to page five, going to page six, making sure these things match. Is it that kind of a hyper monotonous workflow where there’s just a guy named Steve who just sits down and he puts on his visor for the day, because that’s his life, and just does that, or are there multiple people that are involved in different parts of these agreements? What’s these various handoffs kind of look like?
Kris Lorang: So there’s typically a department that receives it. Now different lenders are at various stages of their digital transformation. So it may be that these documents will come in and they’re scanned into their ECM system, and then that department gets notification that that file’s been scanned. So yes, Steve could be essentially there, looking at a stack of files on his desk that he has to get through that day, or he could be looking at his queue in the EM system, looking at a bunch of images he has to get through and instill that stare and compare activity on two screens.
So yes, it’s that process, it’s typically a team of people, and that team could be really, really busy and have a stack of work or depending on volume that could be pretty inactive. So there’s always that resource constraint and then that management you have to do as a lender as well. So in these times today with rates so the low, there’s a ton of refinanced volumes. So there needs to be a room full of Steve’s to be able to process all of these documents. Where as rates get higher, as you get into the holidays, typically in the lower transaction time, that room full of Steves may not be as busy.
Daniel Faggella: Yeah. Steves, they’re crowding the place up… Now you got to worry about how many foosball tables you have. Do you have enough foosball tables when the Steves have nothing to do?
Kris Lorang: That’s correct.
Daniel Faggella: And pardon anybody out there named Steve. I use you constantly as a representative example of a human being, doing a process. I don’t mean to do that. But, okay, so this is really helpful just as a general picture of where things are. Stare and compare, we’ve got different volumes for different seasonality. Some folks have part of the process digitized and they notify different squads. Other folks might just put the stack on one person’s desk and that person is manually going through. But in either case, we’ve got to verify that all the dots line up, because I take it, if we don’t, there’s regulatory concerns, there’s legal concerns, and we just wouldn’t be able to feel confident lending this person, what we agreed to.
Kris Lorang: Correct. And there’s even financial concerns as you sell these loans on the secondary market, certain investors have guidelines that you have to meet when you sell them alone. So part of that post-closing process is to ensure all of the documents that that investor requires and all of the signatures and so forth that is required within that package is accurate and that it meets their guidelines.
So if you were happened to sell a loan to an investor, and that investor audits that sale, as they tend to do, right, when you buy a loan, there could be buybacks that are involved. And what essentially that is, is an investor could say, “Hey, this loan doesn’t meet our guidelines. You have to buy it back, lender.” So that’s money out of that person’s pocket or that lender’s pocket, because they didn’t do that audit accurately.
Daniel Faggella: This feels like 2008.
Kris Lorang: Sort of, yes.
Daniel Faggella: Yeah. When we talk about reselling mortgages and loans, I’m certainly not from the industry, but those of us tertiarily familiar with the last crash, or I guess aware of… The reason why there might be some regulations around that being pretty stringent, what are we actually selling an offering here? So, okay. That makes sense. Now, I guess we can talk about where AI fits into the mix.
Daniel Faggella: So we’ve got a manual process, varied levels of digital transformation between companies, maybe between departments of companies, maybe some is digitized, some isn’t, it might be a team of people working on different parts of the agreement, it might be one person, but no matter what, there’s a lot of dots that have to connect around who’s agreed to this, what have we agreed to sell them, are these terms based on the standards that we told the sales folks they needed to be on in the first place for us to be able to actually underwrite this thing, whatever those stipulations are, stare and compare. So that’s a lot of manual, where do we start to find AI’s fit in all of these processes?
Kris Lorang: So where you start to find a good fit for AI and ML technology is in the actual… So let’s assume that documents are digitized at this point. So once you have a digitized image, you can take that image and classify it and extract data from that using AI and ML. So once you classify that image, then it can be easily sent to your ECM system, classified in the right categories, so you don’t have to manually go back and do that.
But even further down, once you extract that data, that AI can start to learn what type of loan it is. So once it learns that it’s a fixed-rate loan, that it’s a condominium, what kind of property it is, that it’s in a certain state, it can start to look, if you will, for the required documents for that particular state or that particular loan program or that particular property type. Instead of having a person sitting there going, “Okay, this loan is in Pennsylvania. It’s a fixed-rate loan. It’s a condominium. I need to look for these 10 different documents because of those characteristics of the loan.” AI starts to learn that.
And then again, instead of having someone do that, stare and compare activity, they’re essentially maybe looking at some fallout from that AI and ML process, meaning, “Okay, AI and ML has analyzed that particular loan record, but it’s noticed that this particular document is missing because it’s a Pennsylvania-required document and it’s not in the package.” So that person then is doing some more high-level activity. Maybe they have to reach out to the borrower, maybe they have to reach out to the settlement agent, the closer and say, “Hey, we need this document.” So they’re able to do a little more high-value activity than that stare and compare activity.
Daniel Faggella: Yeah. There’s a couple analogies that come to mind, Kris. And I want to see if these click for you, because I really want this to click for everybody listening in, whether they’re in mortgage or not. And I know we have a lot of financial services folks tuned in, but I’m going to try to make this idea click and make sure I’m following you.
As you bring this up, part of me thinks that, okay, in some circumstances, as lending company X, who does mortgages, in some of those cases, when these things come in the front door as paper or as a scanned doc, they’ve already got that appended label that says, “This is a condominium. This is a whatever.” So maybe some folks are already at that labeled phase, and then this application might not apply to them, but probably a lot of companies, they aren’t. They just come in as a raw file, somebody decides what type of doc, and then someone says, “Okay, because it’s a condo, because it’s within this price range,” or whatever the hell that the guidelines are, “now, we need to follow this 30 point checklist. If it’s a multifamily home and it’s in this kind of area, now we need to follow this kind of guideline.”
So it sounds like the distinguishing factor between what kind of a mortgage is this, sometimes maybe companies already have that handled, but some don’t. And for that reason, we might be able to train a system, so when it plops it on the human screen, instead of it just being, “Hey, figure out what this is and what you need to check,” it’ll say, “Hi, this is a condo, and here’s your six checkboxes already pulled up because I’m kind of prompting you to do what you need to do for this workflow already, so you don’t have to think about the light stuff.” Am I tracking here, Kris?
Kris Lorang: Yeah, I think you are Dan, and a good point, is there’s various levels of implementing this type of technology. So I think at least a lot of what I’ve seen is those companies that do the imaging, they’ve simply put the whole closing package in an image, the whole file, and it comes out so-to-speak at the other end, as a large PDF file with all the documents in there. So you can’t tell what documents are in there and which ones aren’t.
Daniel Faggella: So nobody labels it when it’s getting scanned. What you’re getting at is the scanning process is not context-aware. The scanning process is not appending some kind of label to it, that just has to happen after the fact.
Kris Lorang: That’s correct. And in the past that PDF file would go straight into their enterprise content management ECM system. And then it would be labeled as the low number one, two, three, four, five six, seven, eight, nine. So you’d have to find that loan record in your system, pull it up and then you’d have to scroll through, let’s say 300 pages, in a PDF file to find the document you’re looking for if you had to go back and look at it later.
But with AI and ML technology, we take that PDF file in, and some of the industry call that a blob file, take that in and will split that out into individual files. So you can go into a record and say, “I need to look at the mortgage. I need to look at the note.” Whatever the document you need to look at, it’s there, it’s labeled, it’s classified, so you can find it quickly and not have to dig through a 300 page PDF file.
Daniel Faggella: Okay. So part of what we’re getting at here is that AI can label what kind of mortgage this is, but can also kind of set the page breaks to what the different sections are. So if you say, “Bring me to the note,” or, “bring me to everywhere that has a signature,” or, “bring me to…” It’ll be able to sort of label whatever those pages or those sections of the blob file are, so that humans can navigate it a little bit more swiftly?
Kris Lorang: Correct. It’s not so much of a bookmark within a single PDF, I would look at it as more as like splitting it out to it to different parts.
Daniel Faggella: Got it. Breaking document into multiple pieces. So the analogies that come to mind immediately, Kris, are right now chat bot conversation, for example, is not really going to be replacing robust high context, human conversations anytime soon. Similarly, AI may not have the authority to do all the double and triple checking to underwrite a million-dollar mortgage or whatever, or audit a million-dollar mortgage.
Kris Lorang: Correct.
Daniel Faggella: But what some conversational systems can do is they can at least label the conversation as, “This is a refund request. This is a product inquiry about product X. This is a delivery issue. This is a…” And then they can route it to the right human. It sounds like similarly, we’re able to do the labeling on the front end so that the human doesn’t have to say, “What is on my plate?” They say, “Oh, a condo is on my plate. Boom. Here it is.” And then here’s my prompt for what I need to do.
Kris Lorang: Correct. Yeah. That’s certainly probably what I would cal the first level of being able to use that AI and ML technology. Looking further ahead, or for a more advanced system, you could take that data that you’ve extracted from the document using AI and ML technology and place that into a rules engine.
And that rules engine that is built out to have perhaps the guidelines of the investor or your own internal policies or governmental policies around certain regulations. So then you take that data and you can analyze that data and you can perform some of those standard tasks that a person would, “Does the rate on the note match the rate on the mortgage?” Having someone to do the stare and compare, you can actually take that data that’s been extracted and do a complete audit of the file.
Daniel Faggella: Okay. Got it. And so what I would imagine would be necessary here is we would need to, at least on some level, we’d need to train the system on the particular way that we format these particular docs, because I can imagine for every bank or even different departments within a bank, the exact place where people sign X and the exact place where people list how much was Y, in terms of a cost, might be formatted differently.
So in order to actually do that matching and give it the green checkmark, we would need a lot of instances of whatever document format we have and then train an AI system to really, really, really succinctly and reliably extract the right set of numbers from page, somewhere between, 25 and 35 versus most of the time, the right numbers. So it seems as though there’s some bespokeness here to the client’s workflow, the paperwork format, that this particular workflow uses. But if we have enough instances, then we could potentially match two items on different pages. Let me know if that sounds accurate to you?
Kris Lorang: Yeah. That does sound accurate. And certainly, having those trained ML models, machine-learning models, to be able to recognize those different formats of the document within the mortgage industry would be key. Some are more standard than others in the industry. If you look at the mortgage and the note, and in some of the more prescriptive documents that come from a governmental regulations, those look very similar from one ledger to another, depending on the system they use to generate them, but they look very similar. Other ones are not. So it’s those, I think, where you can get those trained models up and it can recognize that it’s that type of document.
Daniel Faggella: Understood. Okay. And I imagine the way that this would work from the user’s perspective, so I always liked to have people kind of, when they’re listening to podcasts, be able to close their eyes and see the workflow play out. The way that I kind of imagine this is the auditing person might have a set of six check button, maybe it’s 16 check boxes on the right-hand side of their document, because they know that this is a condominium mortgage or something. And I have kind of a two-fold guess, number one, when the system says, “Hi, this is a condominium mortgage.” If that’s wrong, the human can relabel it and potentially use that as feedback to the system.
Also, if the machine already has, so let’s say you have 16 checkboxes on the right, if it already has a blue check or a green check next to three of those, because it’s validated them, then the human probably goes in and makes sure maybe that the machine actually was right in putting that green check box and maybe is able to correct the system if one of those assumptions was off in some sense. My guess is that there’s a bit of a loop here in terms of feeding the system, because this thing is presuming here’s the type of doc, it’s presuming, “Hey, these two bits of information match,” but maybe sometimes we need to educate it. What’s that part of the workflow look like?
Kris Lorang: So typically what you’ll find in a lot of AI, ML systems is the ability to set some sort of confidence score. And now what does that mean? So if you look at a document, you could set the confidence score at, let’s say, 90%. Well, then if the system goes through it, as it analyzes the document, it will also say, “I’m about, let’s say, 65% certain that this is a note.” So that it would create a, what we would call, at least at Iron Mountain, an exception.
So that exception has to be viewed by a human in the loop, a human that looks at a cue that says, “The system thought it was 65% sure this was a note, but we’ve set the threshold that it has to be 90% sure before a human doesn’t have to look at it. So we’re going to present this to a human.” So the person, the auditor, we’ll go back to Steve, looks at that document and says, “Yeah, system, you were correct, marked it.” And then the system, as you said, that goes back into the learning or the knowledge of the system itself.
So maybe next time it’s 70% accurate, 80, as it sees this document again and again and again and again. And eventually, the thought is that there would be so few exceptions that it would just be those oddball documents or maybe a document that was a bad image that would fall out of the process. And most of them would be straight through and a person would never have to look at them.
Daniel Faggella: That the goal, I guess, would be to pick… Now, the challenge for you guys running a product company here, an AI product company is what are those junctures of the workflow that we think we actually can get to that point with, right? Because there might be some parts of the workflow where it’s like, “Wow, it would take a long time to make it so that, most of the time, a human doesn’t have to look at this.” But then there are other parts where it’s like, “Hey, we might actually be able to get there.” So you talked about categorizing what type of mortgage it is. It sounds like that’s one that you folks have honed in on.
There are certain kinds of data validation from page X to page Y, that’s one that you folks have honed in on. It sounds like from the perspective of an AI company, you guys have had to really think through which of these do we think can get to a really high level of automated-ness and then build your systems and your offerings kind of around that? Because obviously not everything can be automated.
Kris Lorang: Correct. Yeah, for sure. Obviously, the lowest-hanging fruit are some of the documents that are in every mortgage loan, right? A mortgage or a security instrument, a deed of trust, a note, a closing disclosure, a loan estimate, those documents that are present in every loan package, obviously we have more of those and we’re able to train the system on every package we process, right? So those models are built up and a little more robust than some other ones that, if you look into New York, they have a lot of co-op loans and SEMA loans, which I won’t get into what those are, but there’s less volume of those. So those may take a little longer to train those models, to understand that loan and understand those documents.
Daniel Faggella: Yes, and I think people underestimate the challenge that product companies face. You’ve got to look at, “What’s the value of automating this workflow to the client? What is the viability of us being able to automate this to a degree that we think would be satisfactory to the client? And then maybe what’s the data volume we’re working with to tell us if we really think this is an area of strength for us versus an area where we just don’t have enough volume to actually make those kinds of improvements?” And you’ve got to look through that whole pachinko machine and then find those pinpoints where you can say, “Hey, here’s a spot that we think matters to the client, that we can handle, and that we have the data volumes to do.” And then you’ve got to double down and build out kind of solutions for that.
Kris Lorang: Right. Exactly. You have to look at the process where you’re going to be able to bring the most value to the customers, where you’re going to be able to really address some of their key pain points and make them more profitable and efficient, and ultimately to offer a better customer experience for their customers. Did you say “pachinko machine?”
Daniel Faggella: Pachinko machine, yeah.
Kris Lorang: That’s an old reference. My friend had one of those in his basement.
Daniel Faggella: I use the analogy a lot, for better, for worse. I think sometimes it’s not the best analogy, but I’ve only got so many in the can. So, all right. Yeah, I think a lot of people underestimate how challenging it is to really decide and build out an AI product. Obviously, you guys are already working with so many giant financial institutions, you have your hands on a good amount of docs here, but still being able to pick those areas of value is interesting.
So it’s cool to get your perspective on where are those junctures where we can start that automation. And it sounds like what kind of doc is it in the first place, but then also some of the individual stare and compares, if we can train a system, if we have the right volumes, we can actually just have a green check box that somebody doesn’t have to look at because we can gauge a confidence interval and hopefully make the whole process run smoother.
And you talked about improving the customer experience. Maybe we can end on that. When I think about what is the value of this to a business, the things that come immediately to mind are, “Well, the people maybe have to do a little bit less monotonous stare and compare, maybe some of that stuff is just done for them by the time they get the doc in the first place? And they’re doing maybe some of the more, either the challenging stare and compare or the things that involve some business context and some more strategic thinking.” So maybe that’s a kind of an employee benefit.
There seems to be a value here for the throughput, presuming we could speed things up, that’s also maybe for the business itself and their own efficiencies, but maybe there’s also a speed to having this mortgage all the way closed, and maybe clients would appreciate that. Those are the three things to come to mind for me. When you guys think about the benefits and kind of who’s benefiting from these processes being sort of embedded with AI in the right way, how do you like to articulate it?
Kris Lorang: I think you’ve hit them actually, all three of them, that we think of as well. So you think of the lender being able to scale. We talked about that earlier, having a room full of Steves when volume is high and then maybe one Steve, when volume is low. They have to go through that whole furloughing process, I’ll call it, as their volume increases and decreases, or maybe just a reallocation of assets.
So we think of being able to scale, maybe you only need one or two Steves and some Stephanies in the room to be able to handle all of the volume, regardless if it’s 10,000 loans a month or 1,000 loans a month, because they’re just managing the exception process throughout the automated system.
And then you look, most importantly probably, is the customer perspective. So if you go and close a loan and then two months later, by the time your lender gets through the process and the post-closing process, and you’ve already made your first mortgage payment and they come back to you saying, “Hey, I need this document signed.” You’re like, “What do you mean you need this document signed? I closed two months ago. Why are you coming back to me now? This is really annoying to me.” Sometimes customers will be like, “I’m not signing it.” And then you have to go back to them again. And that kind of process is very frustrating to borrowers. And they’re likely, especially nowadays when they have so many options, not to come back to you for any other financial transactions, maybe if they upgrade their house or refinance in the future, maybe that annoyed them enough.
So if you’re able to get back to them with that missing document within a day or two, perhaps, after the loan closes, that’s probably not quite as annoying, right? Because you’re like, “Oh, we missed this document. We apologize, could you just sign it?” “Sure, whatever.” Send it. So it’s really trying to get to that good customer experience as well as saving the lender some money per transaction. Having a room full of Steves is very expensive. Managing his people, hiring them, laying them off, etc.
Daniel Faggella: You got to get foosball tables for everybody.
Kris Lorang: Yes – foosball tables, real estate, you need a room for them, you need parking, etc. Where if you just have this AI, ML technology, you’d implement it once, it learns, you have these high-value employees that are sitting there processing the exceptions. You only need one foosball table for those two.
Daniel Faggella: I think common value around AI products is sort of, “Can we scale without scaling headcount?” Certainly a value prop there, again, Steves aren’t cheap and especially when volumes are fluctuating, we might have a room full of folks who really have very little to do for an entire season and that might not be the financially best call we can make.
So cool. So for those of you who’ve listened to many of our podcasts in the past, speaking to you listeners, now you know that we like to talk about here in our Tuesday use-case episodes, we look at what’s the business process before, what is it afterwards, and also what’s the business value? Why does this matter, why are we working on this problem? And Kris, I think we’ve hit all three of those today. So I really appreciate you being able to share your expertise with us and join us today on the show.
Kris Lorang: Yeah, thanks for having me, Dan. I really enjoyed it.