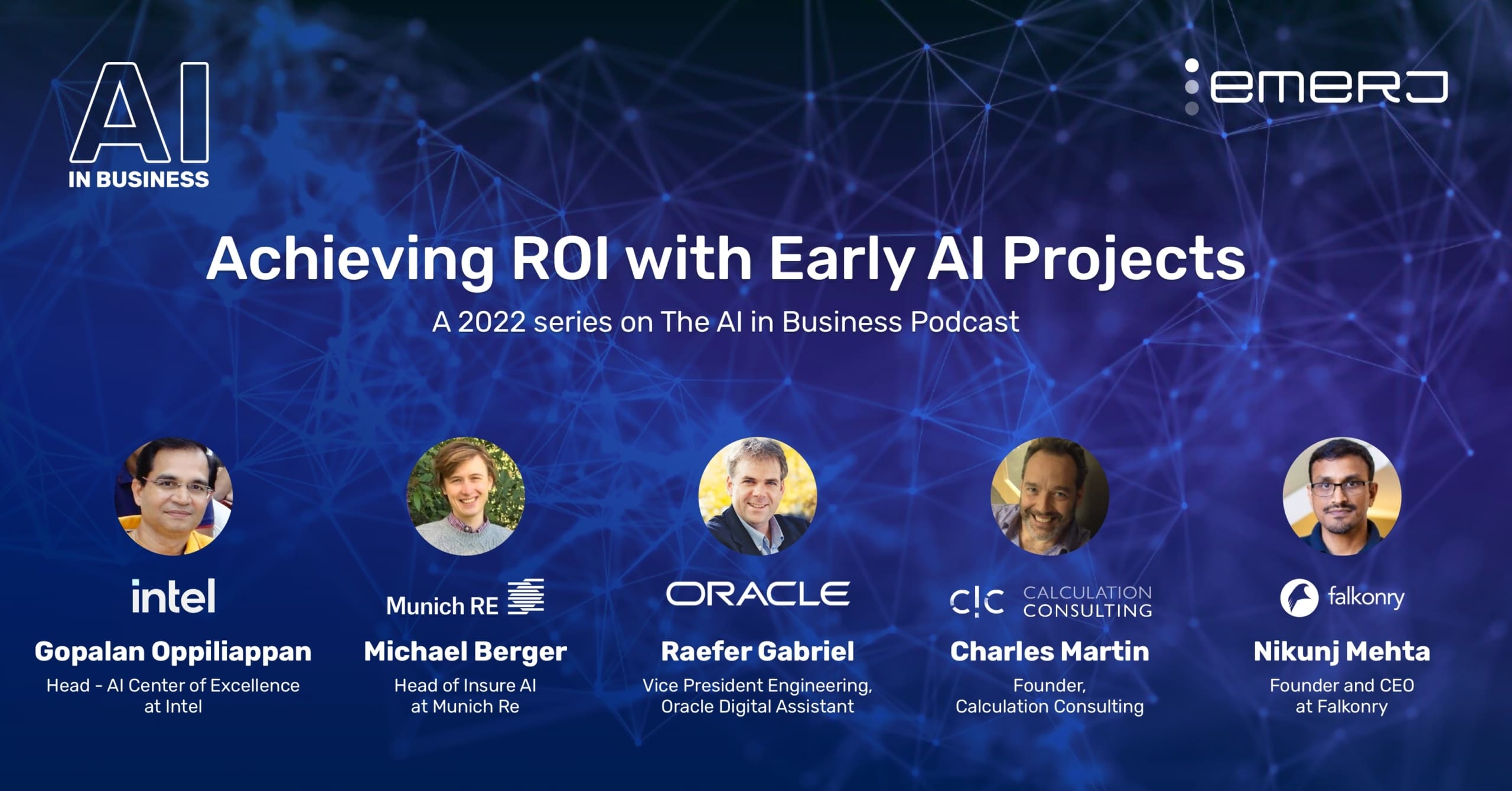
In the enterprise world, more and more companies are crossing the chasm to test, and then deploy, their first AI solutions. To navigate this sometimes unfamiliar territory, enterprise leaders increasingly scrutinize the AI project selection process.
Sometimes, they look back at the safe shore of IT for known precedents and in search of relevant experience, but—unlike even five years ago—they are realizing that AI is not IT. They see the experimental nature of many AI projects. They see the yellow lights of caution flashing ahead.
Understandably, business leaders exercise great care when they select projects for approval. They’re painfully aware that they need to prove some early signs of AI ROI to show wins, earn buy-in, and achieve continued life for their AI deployments.
Today, we start a special series for the AI in Business Podcast. In this series, titled Achieving ROI with Early AI Projects, we draw on the expertise of five AI leaders and explore their journeys through early AI adoption in their respective enterprises, from large multinationals to smaller startups.
In these next five episodes, we will center our discussions on their advice regarding how to achieve a return on the funds and resources we invest into early AI projects.
We distill actionable insights from these AI experts on how to select an organization’s first AI projects, pursue ROI, and gain the confidence of executive management.
Every episode centers on one unifying theme: Achieving ROI with Early AI Projects. We hope you’ll find the advice actionable and applicable to your own AI endeavors.
If you’re not already subscribed to the AI in Business Podcast, be sure to subscribe now on your favorite podcast platforms:
Following the success of our AI Culture Change in the Enterprise series last December, we’ll be bringing series like these to our listeners twice yearly and will continue to bring on high-level guests from organizations of all sizes and stages of maturity. Subscribe to our podcast to receive the latest updates.
For this article and during these five podcasts to be released over the next five days, we will distill the points you need to know to help you move the needle in selecting the right early AI projects that will deliver the ROI you need to convince management to cross the chasm into the land of digital transformation. I’m certain you’ll find as much value when you listen to these accomplished guests as I did putting together each session!
Achieving ROI with Early AI Projects-Advice from the Experts
EPISODE 1 – Best Practices for ROI Measurement
Guest: Gopalan Oppiliappan – Head, AI Center of Excellence – Intel
We open this series with the Head of Intel’s AI Center of Excellence, Gopalan Oppiliappan, who explores the process he advocates for identifying, evaluating, and choosing the early AI projects most likely to deliver ROI.
When it comes to assessing potential AI projects, use a consistent, structured framework and approach, says Gopalan. That means:
- Look for transferrable skills across your team – AI may not be IT, but similarities may extend across projects and you may find that you can bring value to an AI project from past IT experiences.
- Respect the unique challenges of AI and its experimental nature – With AI comes experimentation, but you can’t experiment forever. Experimentation has to be structured and timeboxed.
- Analyze your AI project and develop tangible, quantifiable success metrics – Bring in SMEs from finance and the business and look at cost versus value. Express targeted project goals using metrics like dollars, customer satisfaction score improvements, and growth in engagement.
EPISODE 2 – Quantifying AI Risk
Guest: Michael Berger – Head of AI Insurance – Munich Re
Michael Berger of Munich Re advises leaders to screen AI project candidates for risk immediately.
Michael elaborates on the many types of risks that companies can face in AI projects and talks through the questions that we can ask that will help identify and express those risks. In assessing those risks, he advises us to consider both the impact of the risk were it to occur as well as the likelihood of the risk materializing.
With the risk profile of the projects defined, we can then balance those insights against the projects’ potential upsides before coming to a rational decision point where we select the project that will get the approval to move ahead.
EPISODE 3 – Crawl, Walk, Run
Guest: Raefer Gabriel – Vice President, Engineering – Oracle
Raefer Gabriel, Vice President of Engineering at Oracle, compares AI projects to investment projects. He explains how AI project costs such as hiring data scientists, acquiring and labeling data, and incurring consultancy fees should be compared against potential project ROI and other benefits.
He also explores the possibility of unexpected risks occurring, which could cause liabilities and diminish potential returns. Raefer posits three questions we should consider as we’re sizing up the results delivered by an AI solution during testing:
- Does the AI work as expected?
- How often will I need to retrain the AI model to stay relevant in a changing environment?
- Will the AI solution be secure against cyberattacks?
EPISODE 4 – Data Quality Assessment for Non-Technical Leaders
Guest: Dr. Charles Martin – Founder – Calculation Consulting
Dr. Charles Martin, the founder of Calculation Consulting, emphasizes the importance of knowing when and how to bring in outside experts and appointing project champions who have high AI fluency. Both aid greatly in developing AI ROI and achieving management buy-in.
When choosing project champions, look for experts who have significant authority within an enterprise, believe in the project, and will support it through the hurdles you will likely face. Seek champions who understand the critical differences that exist between AI and IT.
Good project champions can work with data scientists and SMEs to explore the viability of a project and its data and see it through to completion.
EPISODE 5 – Go After Tuna, Not Whales
Guest: Nikunj Mehta – Founder and CEO – Falkonry
Nikunj Mehta of Falkonry points out the importance of including a strong subject matter expert in the workflow of an AI project.
If your AI solution will address a particular problem within a certain set of heavy machinery, for example, you will want to include someone on the team who understands the tools and processes that make the as-is process work. Including SME expertise within your project team is critical for three key reasons:
- SMEs bring strong context to the problems your solution will address
- SMEs often have the best understanding of the data that already exists, its strengths, and its shortcomings.
- SMEs bring credibility to your project and can serve as trusted allies and help build buy-in.
Appoint an SME project champion and develop a playbook to collaborate with them. That playbook will bring them up to speed on working on your AI project, and you up to speed on working in their subject matter area. With that, you can enable their success and win as much buy-in within the organization as possible.