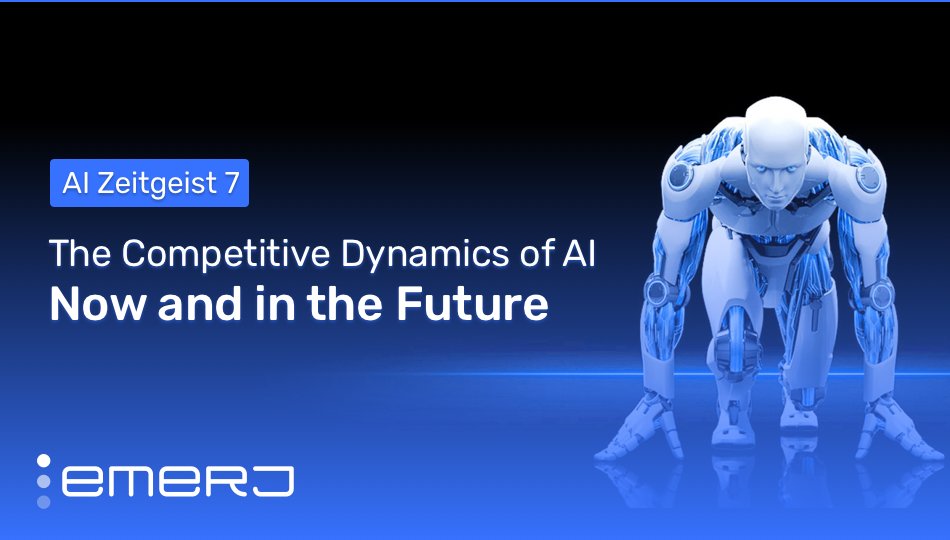
We’ve made it to article seven of seven in this “AI Zeitgeist” series. It’s been a while building up to this, and I’ve kept the competitive dynamics of AI as the topic of this seventh article because to me everything builds up to this.
Everything we do here at Emerj is always about who's going to win in the market in the future, and so we need to give an inordinate amount of thought to this topic.
(Note: If you haven’t read the first article in the AI Zeitgeist series [linked below], you should familiarize yourself with it first. In that article, I explain what the 3 phases of “Emergence,” “Adoption,” and “Dispersion” actually mean).
For those of you who may not have read the previous installments in the "Zeitgeist" series and may be interested in exploring the topics we cover in this article more in-depth, you can navigate to them below:
The 3 Phases of AI in the Enterprise: Emergence, Adoption, and Dispersion (AI Zeitgeist 1)
How “AI” Will be Discussed in the Future...
You've landed on exclusive content for Emerj Plus Members
Emerj Plus Membership
In-Depth Analysis
Consistent coverage of emerging AI capabilities across sectors.
Exclusive AI Capabilities Matrix
An explorable, visual map of AI applications across sectors.
Exclusive AI White Paper Library
Every Emerj online AI resource downloadable in one-click
Best Practices and executive guides
Generate AI ROI with frameworks and guides to AI application