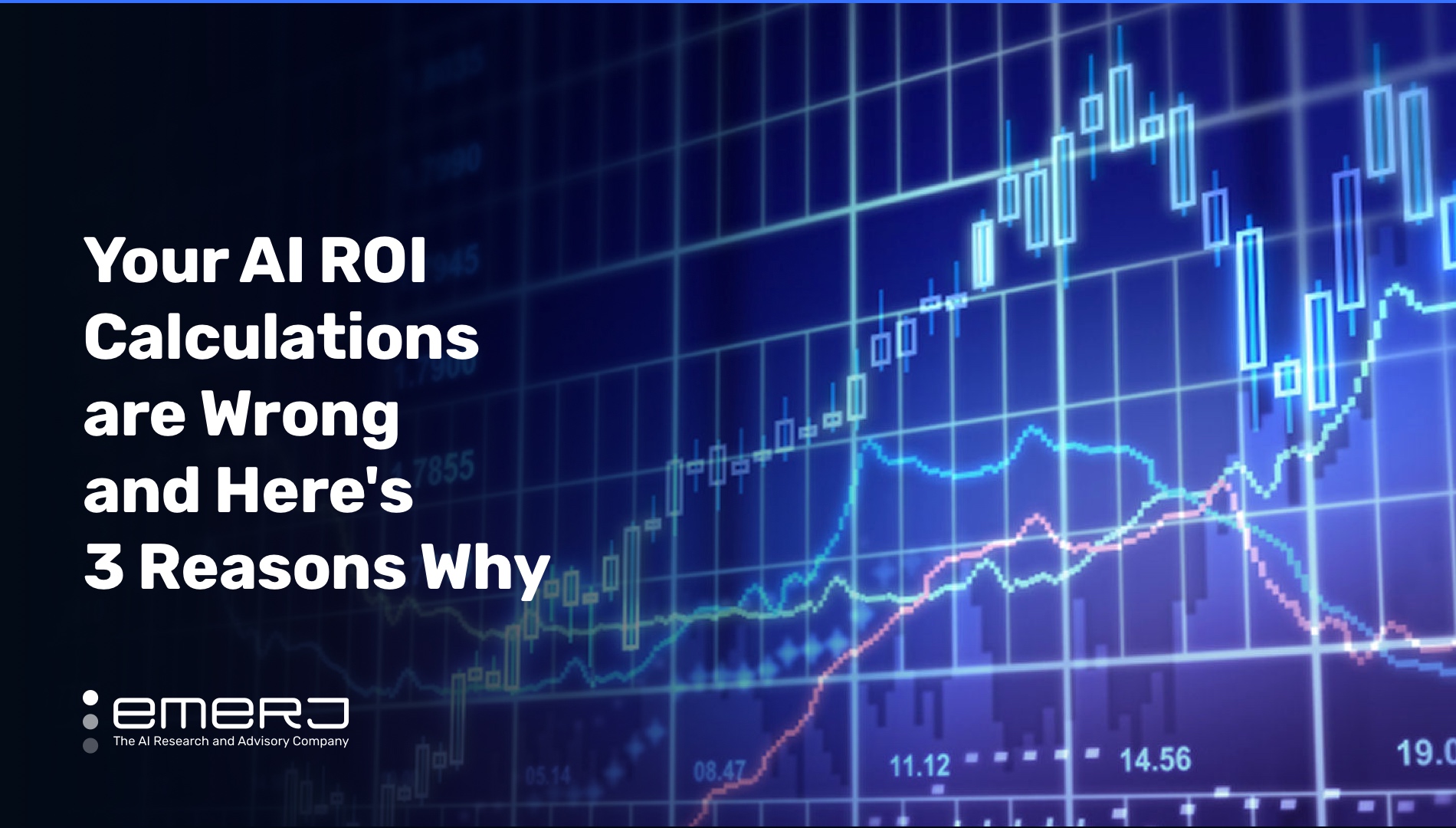
After spending the last two weekends putting the finishing touches on our new AI ROI Cheat Sheet, my mind is swirling with quotes and AI ROI "rules of thumb" from some of our smartest interviewees and research advisors.
There are two lessons that come through time and time again in our most recent batch of AI ROI research:
Determining AI ROI isn't easy. It often takes AI startups two or three years to determine the core "needle-moving" metrics that they use in presenting a business case to clients. There is a balance between measurable (often financial) ROI and strategic ROI that must be maintained. There is a balance between "metrics our client cares about" and "metrics we can reliably impact" that must be considered. Smart AI services providers know how critical it is to present an ROI case, and take the time necessary to do it well.
Framing AI ROI the wrong means failed (or short-lived) projects. For AI consultants - or for internal innovation leaders within an enterprise...
You've landed on exclusive content for Emerj Plus Members
Emerj Plus Membership
In-Depth Analysis
Consistent coverage of emerging AI capabilities across sectors.
Exclusive AI Capabilities Matrix
An explorable, visual map of AI applications across sectors.
Exclusive AI White Paper Library
Every Emerj online AI resource downloadable in one-click
Best Practices and executive guides
Generate AI ROI with frameworks and guides to AI application